Privacy, efficiency on the agenda
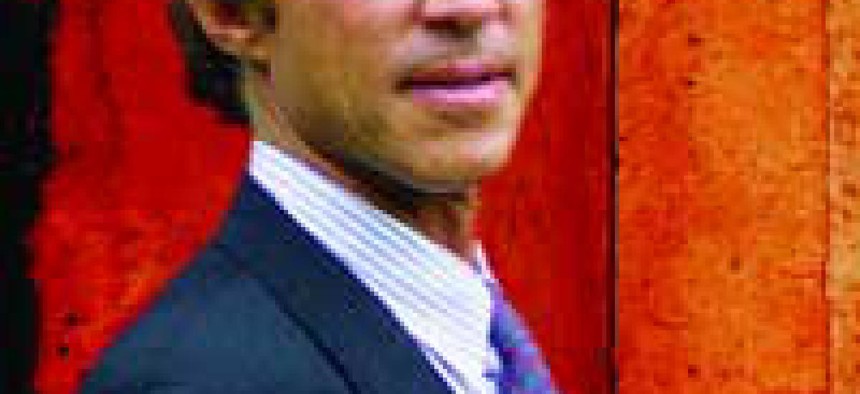
Kim Taipale takes an approach to data mining that melds his training as an attorney with evaluation of the uses of modeling and statistics.
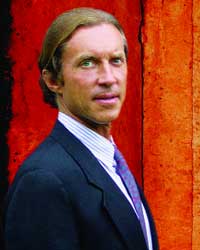
There is always the possibility for reverse-engineering and countermeasures. ... no system should be relied on by itself.'
Taipale, who is a partner and adviser to investment companies and banks, also serves on the board of the Markle Task Force on National Security in the Information Age and the steering committee of the American Law Institute's project on government access to personal data.
He testified earlier this month before the Senate Judiciary Committee about how federal agencies could use advanced analytic tools to protect privacy and improve investigative methods.
GCN: What types of data analysis would be most likely to pinpoint links to social networks of criminal gangs or terrorist networks?
TAIPALE: I am not sure that data mining should be thought of solely in regard to the question of detecting terrorists. I think [advanced analytic software] can be used effectively to allocate intelligence resources to more productive uses. It means you are not using automated means to cast suspicion on anyone in particular, but rather to shift intelligence and law enforcement resources to more effective uses.
For example, it is common for big-city police forces to use Compstat or similar statistically based policing tools to allocate resources to high-crime areas. When you do that, you are not saying that any particular person in that neighborhood is a suspect, you are targeting resources more effectively. In counterterrorism, it is the same construct, of deploying resources on a risk and threat management basis.
The goal is to use statistical models to shift resources depending on the particular [events, individuals, networks or incidents] you are looking for.
GCN: What types of models and algorithms are best for extracting useful counterterrorist data from large databases?
TAIPALE: This depends on the particular threat you are modeling and the particular data you think might be relevant. It is a very domain-specific thing. It requires human analysts with deep domain experience working together with computer specialists to design the algorithms that define the kinds of relationships or attributes that might be relevant.
GCN: How should law enforcement and espionage agencies define and segment their data-mining operations to make the distinction between searching for probable cause, with a view to prosecution, and intelligence information, which has other uses?
TAIPALE: I am not sure you need to segment the data at all. To some extent, the results of the analysis will segment themselves by virtue of the mission of the agency conducting the analysis.
The New York Police Department does analysis to decide how to deploy its law enforcement resources. I think, to a large extent, the agencies' and analysts' decisions are more related to the threats than [to] the particular methods they use. If it is a huge threat to national security, you would want to leave it to the intelligence agencies. If it is just a small group of guys plotting an attack in the future, you might want to prosecute them and get them off the street.
GCN: How do 'ensemble classifiers,' or analysis filters that process a database simultaneously, work with other tools such as rankings, multipass inferences, and relational and probablistic modeling, as well as with known facts, to build effective data-mining systems?
TAIPALE: Using multiple, independent models to analyze a database and extract information improves statistical accuracy. There are two aspects to this.
First, false positive results that suggest connections to a terrorist or a terrorist indicator can be weeded out by using cross-correlation via multiple models. This method greatly reduces the chances of false positive results quickly, because true positives have many attributes in common, and false positives will not.
The second point goes to relational and propositional database analysis issues. The statistical significance of correlating behavior from propositional data among unrelated entities [such as people] is highly related to the number of instances [for example, of meetings or phone calls].
But the correlation of behavior among related parties [such as known members of a terrorist group] may take only a single observation [such as a meeting].
GCN: What types of architectural designs for advanced analytical systems can help reduce false positive and false negative results?
TAIPALE:It's necessary to have a multistage classification architecture, where rule-based processing can govern the analysis and apply human decision-making points. At those decision points, further analysis becomes a policy question. You can build an architecture in a way that recognizes the need for human intervention and then, as a policy matter, decide what intervention you want at those points.
GCN: If the federal government uses rule-based processing to populate its controversial Advanced Tracking System tool for assigning risk scores to travelers, is there a possibility that terrorists could attempt to reverse-engineer the rules by challenging ATS with known test sets?
TAIPALE: Yes, there is always the possibility for reverse-engineering and countermeasures. Two critical points here are that no system should be relied on by itself. You need security in depth, such as random screenings. The other point is that you can actually spot avoidance behavior and countermeasures. So, again, an effective counterterrorism strategy or military strategy forces the enemy to take countermeasures that are known and that have signatures that you can spot.
GCN: How can the government provide a secure method of removing a person's name from a watch list or no-fly list while being assured that the 'real bad guy' still gets caught'especially if the shared identifier is only a name?
TAIPALE: This is a significant problem that has not gotten enough attention. The first [problem for a traveler] is even knowing you have been flagged. If you have been denied access to a flight, [that is an affirmative indication that you have been flagged]. We have to be very careful not to secretly tag people. It depends on what the context is.
If you are triggering adverse consequences, then we need to focus on allowing people to know that they have been tagged and to correct any mistakes. But if you are only allocating intelligence resources, disclosure is less important.
The second problem is, assuming you trigger the redress process, how do you allow knowledge of whether a person has been tagged, or correction of the records, to happen without revealing selection procedures? We need to think creatively about ways to have as open a correction process as possible without disclosing to the bad guys what is going on inside the government, so they take countermeasures.
In some cases, it might be appropriate to have an advocate inside an agency. The internal advocate can make sure the correction gets done in a way that the specifics of the internal selection process are not publicly revealed.
NEXT STORY: Feds split on FISMA's effectiveness