Data analytics, machine learning help find network threats
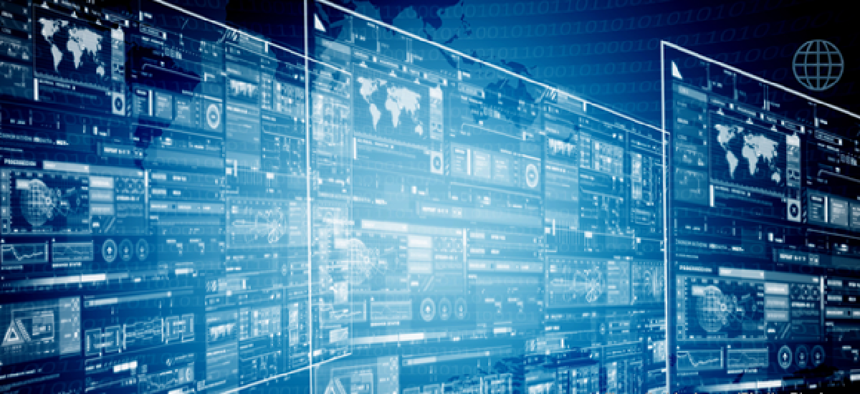
Connecting state and local government leaders
Enterprises can better protect themselves from cyberattacks if they combine automated network threat detection tools with traditional perimeter security methods, according to a new report from the SANS Institute.
Enterprises can better protect themselves from cyberattacks if they combine automated network threat detection tools with traditional perimeter security methods, according to a new report from the SANS Institute.
"The Expanding Role of Data Analytics in Threat Detection" describes how automated threat detection tools that use data science, machine learning and behavioral analysis can support traditional security methods. Such analytics can help organizations better monitor network traffic, CPU usage and port activity for unique events or trends, and assist in watching for abnormal behavior of end users and applications to identify potentially malicious activities.
In a research project sponsored by Vectra Network, the SANS Institute found that modern cyberattacks occur in three phases, which often take months to play out. In the first stage, attackers penetrate a network and establish a foothold, typically through a combination of social engineering and malware, as in the case of an email phishing attack. In the second, the attackers adopt legitimate user credentials or create new ones that let them escalate their privileges and move laterally within the network. In the final phase, attackers steal intellectual property, identify information or financial data.
There are three methods of detecting network attacks, according to SANS. The first and most common method is signature-based or misuse detection, which watches for patterns of events specific to documented attacks and uses this information to identify intrusions and viruses. However, this method can identify only documented threats. The second method is anomaly- or behavior-based threat detection, which creates models of normal behavior for networks, systems, applications, end users and other devices, and then looks for deviations from those patterns of behavior. The third method is continuous system health monitoring, which involves actively tracking the performance of key systems to identify suspicious activity or resource usage.
"These people have patience," said Sean Michael O'Connor, assistant CIO at Worcester Polytechnic Institute. "The acquisition of our university's data is somebody else's business model. That's how they make money. And once these people get in, they want to stay in as long as they can to elevate their privileges and to acquire as much data as they can.” Once hackers get inside a network, he said, “identifying that they are in is crucial to making sure that you shut these guys down before something bad happens and before they acquire that data."
Worcester Polytechnic Institute has just completed a six-month beta test of a security tool from Vectra Networks that detects lateral movement within a network and looks at the analytics and compares it to the university's baseline to identify anomalies, according to O'Connor.
The Vectra Networks system has already identified numerous command and control anomalies on the university's network. "Right there, it's worth the price of admission, as they say, if you're going to start being able to detect things that you wouldn't see normally," O'Connor said. After the system spends a few weeks to “learn” a network’s patterns and normal operations, “you start actually getting interesting data,” he said. “Now that we've had it in for six months, the stuff that we're seeing is really interesting. We wouldn't have caught that before."
Read the SANS report here.
This article originally appeared on Campus Technology, a sister site to GCN.
NEXT STORY: National Guard expands cyber protection teams