Turning the IoT side channel from liability to asset
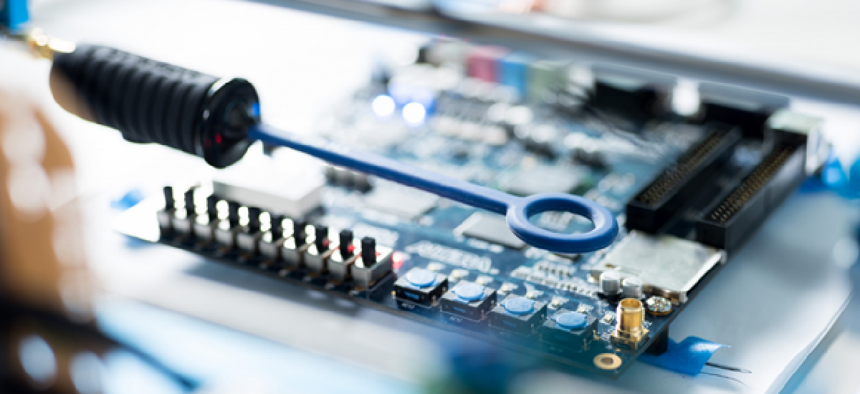
Connecting state and local government leaders
By monitoring the electromagnetic emissions produced by sensors on the Internet of Things, researchers hope to be able to detect when those devices have been compromised.
Security experts have warned that sensors on the Internet of Things are especially vulnerable to being hacked and opening access to the broader network. Because IoT sensors are small by design, they have so little computing power that traditional security software can’t be run on them.
To detect the presence of malware without running any programs on the IoT devices themselves, researchers at the Georgia Institute of Technology are focusing on what has to date been considered an Achilles’ heel of IoT -- side-channel signals, the unintentional electromagnetic emissions that are produced by the electronic devices as they execute programs.
The Georgia Tech team, led by Assistant Professor Alenka Zajic and funded by a $9.4 million grant from the Defense Advanced Research Project Agency, has developed software to analyze the magnetic fields generated by the emissions from transistors and other components of IoT sensors.
Once the software -- Computational Activity Monitoring by Externally Leveraging Involuntary Analog Signals, or CAMELIA -- registers the normal pattern of emissions of an IoT device, it monitors for changes that indicate the presence of malware.
"When a processor executes instructions, values are represented as ones and zeroes, which creates a fluctuation in the current," Zajic told Georgia Tech Research News. "That creates changes in the electromagnetic field we are measuring, providing a pattern for what each part of the program looks like on a spectrum analyzer."
Zajic said she and her team have been working on side channels for almost eight years. “We were trying to understand the relationship between the emanations that are coming out of the electronics and the software activity,” she said. “Once we understood that there is a relationship -- that it is not a random process -- then we said, ‘OK, maybe we can monitor what the program is doing.’”
"If somebody inserts something into the program loop, the peaks in the spectrum will shift and we can detect that," Zajic said. "This is something that we can monitor in real time using advanced pattern-matching technology that uses machine learning to improve its performance."
CAMELIA is particularly effective when it is able to work with prerecorded profiles of IoT devices that are known to be clean of malware. It can then simply observe the normal patterns and use machine learning to train itself to recognize those features, Zajic said. Accordingly, the Georgia Tech team is busy profiling a wide array of IoT devices and is building a catalog of profiles.
Currently, the range of CAMELIA’s monitoring capability is only a half meter, but Zajic said the objective is to extend that to three meters. “The goal is to have one monitoring device for multiple IoT devices,” she said. That may allow a single CAMELIA device to monitor an average-size room.
The remaining technical challenges to reaching that goal, Zajic said, are designing antennas that can pick up signals across greater distances and developing techniques for localizing the most effective sources of emissions to monitor in the IoT devices.
NEXT STORY: Federal BYOD: The mobile security conundrum