Facial recognition shows big improvements
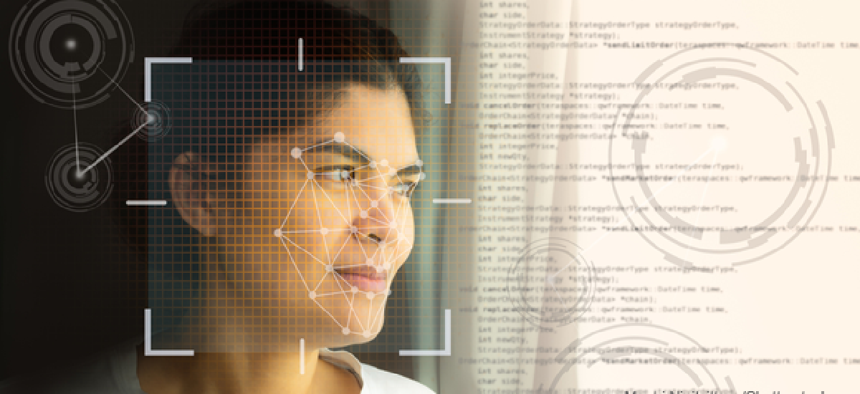
Gains in the accuracy of facial recognition technology over the last four years have been "revolutionary and not evolutionary," according to an expert from the National Institute of Standards and Technology.
Facial recognition technology has already made its way into the mainstream, but to get the most accurate results, organizations should be sure they're using the latest algorithms, said Patrick Grother, a computer scientist at the National Institute of Standards and Technology who administers the agency's Face Recognition Vendor Test.
Face recognition has undergone a big transformation in the past four years thanks to the use of convolutional neural networks for model training. More vendors have started using CNNs, which has resulted in significantly better accuracy as demonstrated in the most recent vendor test released by NIST at the end of November, Grother said.
Any organization that has been doing face recognition "should probably be thinking about going into a technology refresh cycle with their provider -- or maybe recompeting to find a new provider of the technology -- because the accuracy gains are revolutionary and not evolutionary," he said. "There is low-hanging fruit there -- easy gains in accuracy to be had just by replacing an algorithm.”
The NIST report looked at one-to-many facial search algorithms, which covers applications where one photo is compared against a database of images to find a match. This is different than one-to-one applications used for verification, like Apple’s Face ID.
The last time NIST evaluated this kind of algorithm in a vendor test was 2014. The 2018 results showed a 95 percent reduction in error rate compared to the 2014 test. The progress made is symbolic of a serious transformation in the underlying technology, Grother said.
“If I search a database [for] someone who is in that database then I fail to find that face 20 times less often than I did four years ago … I am successful on searches that four years ago didn’t succeed,” he explained.
But there remains a large range of accuracy across the industry. Open source tools like Caffe and TensorFlow make training CNNs fairly accessible, but considerations like the quality of data used to train a model and what exactly happens during training can separate performance of algorithms in the field.
“Those details mean that some [algorithms] turn out to be very accurate and some … much less accurate, so there’s this spectrum across the industry,” he said.
This was the first year Microsoft, a big player in the facial recognition space, entered an algorithm into the NIST vendor test. And it came out on top in one of the two categories.
NIST looked at two types of searches in this test. In the investigative search, one image is given to the algorithm and it is asked to return the 50 most likely matches from the database of images. NIST measured the algorithm's success by seeing how often the right result appeared at the top of this list.
“On that metric,” Grother said, “Microsoft has the most capable algorithm.”
The Chinese company Yitu took the top spot in the other category where the algorithm is asked to return a single image with a confidence score. In this case -- if the algorithm functions correctly -- nothing is returned if no match exists in the database.
Demographic differences are often discussed in conversations about the accuracy of facial recognition technology. There have been multiple tests showing that facial recognition systems can struggle with images of people with darker skin tones. Although NIST's November test did not address that issue, the agency is in the middle of another test designed to look specifically at these concerns. It plans to publish the report – that takes age, race and gender into consideration -- in the first quarter of 2019, Grother said.
He underscored that the report will look at the technological differences between algorithms and not at societal differences that result in some populations being more well represented in training data. But, as overall accuracy numbers improve -- as seen in this recent test -- the difference in error rate between demographics is also expected to shrink.
“So when we see a demographic difference, those differences are smaller now than they were four years ago,” he said.
Concerns over demographic differences have led to call for regulation in the space, especially given that it's being used by law enforcement. Joy Buolamwini, a computer scientist at the MIT Media Lab, recently spoke at a Federal Trade Commission meeting where she asked the agency to do more to regulate facial recognition technology.
The issue has also caught the attention of Democrats in Congress. In a letter to Amazon CEO Jeff Bezos, lawmakers voiced concern at the accuracy of the company's facial recognition technology and said it “places a disproportionate burdens on communities of color, and could stifle Americans’ willingness to exercise their First Amendment rights in public.”
Amazon’s technology, which has been used by multiple law enforcement agencies, came under scrutiny after the American Civil Liberties Union ran a test that resulted in 28 members of Congress being matched to pictures of individuals in a mugshot database. Amazon said the ACLU wasn’t using the technology correctly.
The their letter to Bezos, lawmakers asked for details on how Amazon tests its technology for accuracy differences between demographics and requested a response by Dec. 13.