Safety in biometric identification: Liveness detection technology
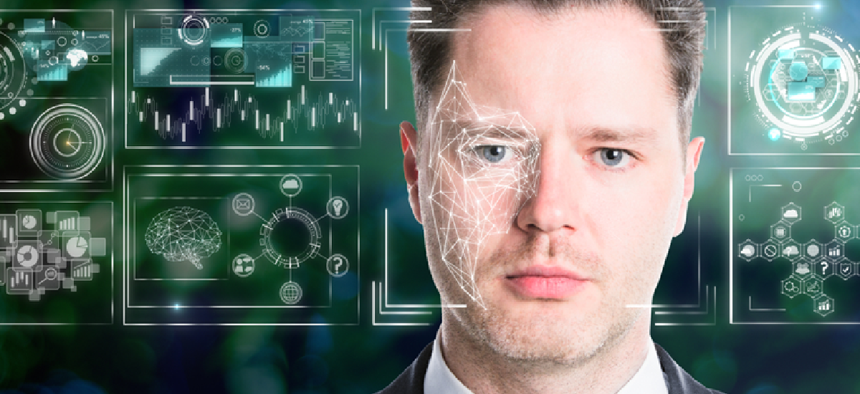
Liveness detection reduces the risk of spoofing by using advanced technologies ensure that biological identifiers are from the proper user and not from someone else.
As biometric authentication slowly becomes incorporated into security and law enforcement applications, increasing attention has been paid to the quality and accuracy of the technology. In an attempt to win public support and minimize misidentification and security breaches, many biometric systems now promote an additional security feature: liveness detection.
Biometrics are security technologies that use a person's unique features, such as fingerprints, face or retina and iris patterns, as a replacement for IDs, passwords and other methods of identification. To use biometric authentication, users who are enrolled in a system can be transparently identified as the person in view by comparing the current biometrics to those already in the system.
Facial recognition is improving with new 3D technology systems that can detect slight changes in a person’s features. Through advanced analytics, new facial hair, glasses and even partial obstructions of the face are less likely to prevent user identification, and progress is also being made on accurate identification in both dim and bright light and with people of all complexations. Yet while the rate of false rejections is falling and accuracy improving, the majority of the solutions can still be easily spoofed.
Spoofing biometrics is when individuals use any type of replication to convince the system they are someone else. Fingerprints have been spoofed with adhesive tape and gummies, while photos, videos, masks and makeup have all been used to defeat facial recognition. This is a documented weakness of all biometrics, and as this identification technology becomes increasingly common, these issues are impacting the confidence users have in it.
What is liveness detection?
Liveness detection is a security feature that can ensure biological identifiers are from the proper user and not from someone else. Traditional forms of detections can include eye or lip movement analysis, prompted motion instructions, texture/reflection detection in video feeds or zooming motion detection. Another new technique is 3D depth analysis.
There are two approaches for liveness detection: active and passive. Active liveness detection refers to methods where the user performs an action, such as blinking, making facial movements or keystroking, which makes it more difficult and time consuming for the user to spoof the system. Passive liveness detection uses internal algorithms to detect spoofs and requires nothing from the user.
When logging into a banking app using facial recognition, for example, an active liveness detection system may require users to blink while it scans their face. Passive liveness detection, meanwhile, may scan a user's face to ensure that a real human face is present with the individual’s proper depth contours. Many liveness detection methods, especially active approaches, take longer to identify users, reducing the speed, convenience and transparency of biometric identification.
Issues facing liveness detection
Although many of these methods can be easily implemented, most can still be easily defeated. This is because common biometric identification programs rely on 2D images, meaning that they process faces as 2D objects and lack spatial awareness or depth analysis. If the biometric security programs are viewing users as 2D objects, it is easy to see how they can be defeated using 2D photographs.
Systems must also consider the size of the object that is being presented. A photograph of a face on a three-inch screen should not be detected as a full-sized face, but many systems still do not have the spatial awareness to distinguish this difference.
Many liveness detection methods also only work in constrained conditions, such as in offices and labs. In environments where features are obscured, such as in bright glaring light or dim settings, systems can often misidentify a person, which results in false rejections. Security must also be available in real-world conditions, meaning that many of the common liveness detection methods working in the lab cannot be reliably implemented.
The future of liveness detection
Biometric identification has come a long way, and with it liveness detection methods have improved. Technologies are being implemented that make liveness detection more secure, yet still efficient enough to avoid user frustrations.
One current solution uses 3D cameras for biometric identification, which allow systems to detect when photographs or replicas, including 3D printouts and masks, are being used. This technology can be easily adopted into identification technologies, and it increases security without any extra effort from the user.
AI learning is also being used to improve biometric security and reduce rates of false rejection. Other techniques, such as forward learning and skin reflectance testing, are being employed to enhance anti-spoofing technologies and improve models on the fly.
For the public opinion of biometric security to improve, liveness detection and anti-spoofing methods must also improve. This will mean implementing accurate and accessible liveness detection that continues to have the speed of traditional biometric identification methods.