How AI can help improve intrusion detection systems
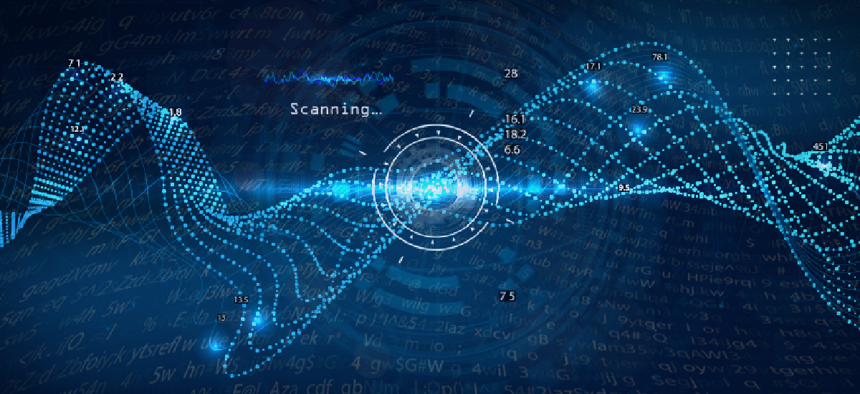
Connecting state and local government leaders
Researchers are converting real network assets into artificial intelligence-powered honeypots that learn from attacks, transforming each incident into live training data for machine learning-based intrusion detection systems.
Questions about user privacy and how organizations keep personal information safe are perennial concerns. With the coronavirus pushing more people online, both public- and private-sector organizations must be even more vigilant in protecting their networks.
For any network or system, frontline defensive duties fall on intrusion detection systems, which can either be rule-based or algorithm-based. A rule-based IDS subjects all traffic to a set of rules before allowing it to pass through, like a security checkpoint during a lockdown. An algorithm-based IDS, on the other hand, uses machine learning to dynamically create new detection algorithms based on the traffic.
Both these systems can analyze and identify traffic as good or malicious, playing a crucial role in defending a system or network from attacks. Many IDS systems today are AI-based because of the advancing technology available to both network security teams and cybercriminals. As threats evolve dynamically, however, it's becoming increasingly difficult to write a set of rules for these machines to follow. This is where letting machines handle writing the rules themselves comes in.
AI-powered intrusion detection
With all the cyberattacks and data breaches, IT teams are turning to AI to beef up their security efforts. AI adoption for intrusion detection is slowly getting there, with 44% of organizations worldwide using some form of AI to detect and deter security attacks on their network back in 2018. While the number using AI-based IDS should be arguably much higher, the technology is still under active development.
One challenge involves adversarial AI. Modern IDS, while good at detecting regular intrusions, is weak against adversarial AI attacks in which attackers inject malicious input – false positives and negatives -- into AI training data. A false positive occurs when good traffic gets incorrectly flagged as malicious and denied before it can enter the system. A false negative works in the exact opposite way: malicious traffic is considered good and allowed to enter the system. Adversarial AI uses false positives and negatives to fool the system into allowing malicious traffic to enter and infiltrate the network.
A promising development in the battle against adversarial AI is currently underway as researchers use honeypot-style defenses to beef up machine learning for deception technology. Deception technology is defined as decoy systems or traps placed at strategic areas around the network. These decoys, or honeypots, attract the attention of attackers that penetrate networks that have been deliberately engineered to confuse attackers. They make it harder for adversaries to locate where the real assets are and allow observers to watch attackers’ tactics as they probe networks.
A shortcoming of existing deception technology is that the defense is mostly static, and the system doesn't learn from previous attacks. An AI-enabled adversary, however, learns over time and, given enough time and data, will be able to distinguish a honeypot from a real asset and beat the decoy defenses.
Researchers at the University of Texas at Dallas aim to take the honeypot concept and develop it further. DeepDig, or DEcEPtion DIGging, “plants traps and decoys onto real systems before applying machine learning techniques in order to gain a deeper understanding of attackers’ behavior,” according to The Daily Swig. DeepDig uses real assets and converts them into traps that learn from attacks, transforming each cyberattack into live training data for a machine learning-based IDS.
There are many advantages to turning real assets into honeypots. Even the most proficient adversary “cannot avoid interacting with the trap because the trap is within the real asset that is the adversary's target, not a separate machine or software process,” UT Dallas computer science professor Kevin Hamlin said. The DeepDig defense will get better over time and will learn how to stop even the stealthiest adversaries, he said.
With the growing number and sophistication of cybersecurity threats, organizations and governments need to fast-track this type of technology.
NEXT STORY: How scammers can siphon off stimulus payments