Researchers raise concerns with differential privacy use on census data
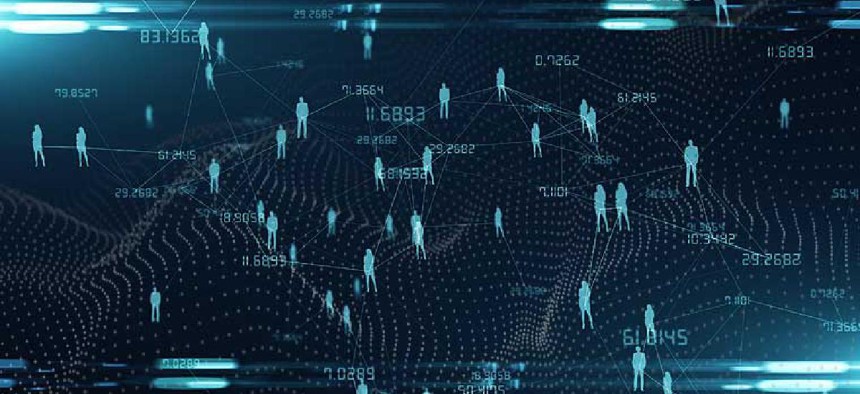
By focusing on mortality rates among racial and ethnic minorities, Penn State researchers found that the privacy-enhancing data technique caused undercounting in rural areas and overcounting in cities.
After the Census Bureau announced in 2018 that it would use differential privacy to protect the identities of individuals for the 2020 census, researchers at Penn State began to evaluate how these changes could affect census data integrity.
Differential privacy injects random "noise" into the aggregate data in an effort to better protect the identities of individual respondents when the data is published.
Nicholas N. Nagle, an associate professor of geography at the University of Tennessee who analyzed census test data, explained the technique this way: “In a nutshell, differential privacy involves not reporting exactly accurate numbers – like ‘5 people in Bigtown City are Hispanic males’ – but rather a random number relatively close to the accurate one, like 11. These random errors make it much harder for a data scientist to go back and figure out which Hispanic male in that city might be connected with a specific public record. And the public has some information, though it’s not exactly accurate or complete.”
Nagle said his analysis showed that state population counts are completely accurate, and estimates for large populations -- like the number of 20-year-olds in Virginia, or the number of Hispanic people in Los Angeles -- are relatively accurate. Data on small populations, however, was “unacceptably wrong,” he said, citing an example of Kalawao County, Hawaii, a former leper colony, which had so much randomness added to its data that its population count jumped from 90 to 716.
The Penn State researchers zeroed in on mortality rates among racial and ethnic minorities and found that, compared with traditional methods of identity protection, using differential privacy on the 2010 census data produced dramatic changes.
"We focused on mortality rate estimates because they are an essential population-level metric for which data are collected and disseminated at the national level and because mortality rates are a critical indicator of population health," Alexis Santos, assistant professor of human development and family studies, told Penn State News.
"We discovered that by using differential privacy, there were both instances of under- and over-counting of the population. In rural areas, there was undercounting of racial and ethnic minorities, while in urban areas there was an overcounting of these populations," he said. In some cases, discrepancies between the two methods of data analysis exceeded a 10% difference.
"This is very concerning because it could impact how much funding programs receive for a specific geographic area," said Santos. "These discrepancies could result in understated health risks in some areas, while overstating in others where there isn't a great need."
According to Santos, the findings highlight the consequences of implementing differential privacy and demonstrate the challenges in using the data products derived from this method.
"The Census Bureau has been very receptive to our research, and demonstrated concern about the accuracy of the data," Santos said. "We plan to move forward with additional research to determine how differential privacy may affect population growth estimates and populations changes from census year to census year. We still have time to fine tune the differential privacy algorithm, and our research will help pinpoint areas of improvement."