What’s wrong with this picture? Teaching AI to spot adversarial attacks
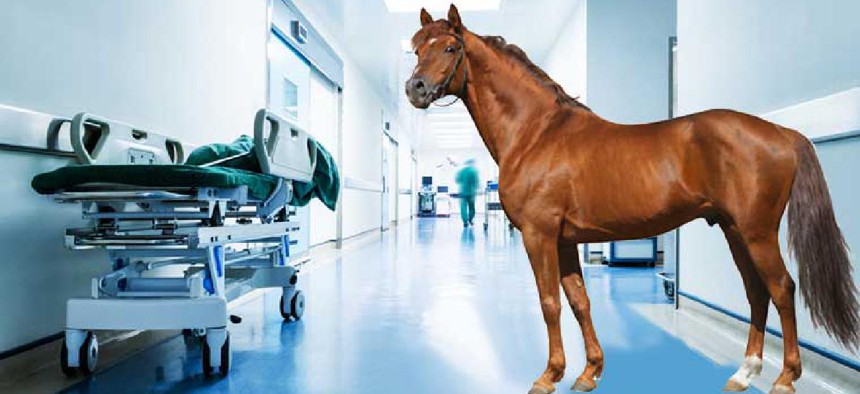
Connecting state and local government leaders
Researchers are attempting to harden computer-vision algorithms against adversarial attacks by teaching them to recognize small details in the scene that are absent or altered.
Even mature computer-vision algorithms that can recognize variations in an object or image can be tricked into making a bad decision or recommendation. This vulnerability to image manipulation makes visual artificial intelligence an attractive target for malicious actors interested in disrupting applications that rely on computer vision, such as autonomous vehicles, medical diagnostics and surveillance systems.
Now, researchers at the University of California, Riverside, are attempting to harden computer-vision algorithms against attacks by teaching them what objects usually coexist near each other so if a small detail in the scene or context is altered or absent the system will still make the right decision.
When people see a horse or a boat, for example, they expect to also see a barn or a lake. If the horse is standing in a hospital or the boat is floating in clouds, a human knows something is wrong.
“If there is something out of place, it will trigger a defense mechanism,” Amit Roy-Chowdhury, a professor of electrical and computer engineering leading the team studying the vulnerability of computer vision systems to adversarial attacks, told UC Riverside News. “We can do this for perturbations of even just one part of an image, like a sticker pasted on a stop sign.”
The stop sign example refers to a 2017 study that demonstrated that images of stickers on a stop sign that were deliberately misclassified as a speed limit sign in training data were able to trick a deep neural network (DNN)-based system into thinking it saw a speed limit sign 100% of the time. An autonomous driving system trained on that manipulated data that sees a stops sign with a sticker on it would interpret that image as a speed limit sign and drive right through the stop sign. These “adversarial perturbations attacks” can also be achieved by adding digital noise to an image, causing the neural network to misclassify it.
However, a DNN augmented with a system trained on context consistency rules can check for violations.
In the traffic sign example, the scene around the stop sign – the crosswalk lines, street name signs and other characteristics of a road intersection – can be used as context for the algorithm to understand the relationship among the elements in the scene and help it deduce if some element has been misclassified.
The researchers propose to use context inconsistency to detect adversarial perturbation attacks and build a “DNN-based adversarial detection system” that automatically extracts context for each scene, and checks “whether the object fits within the scene and in association with other entities in the scene,” the researchers said in their paper.
The research was funded by a $1 million grant from the Defense Advanced Research Projects Agency’s Machine Vision Disruption program, which aims to understand the vulnerability of computer vision systems to adversarial attacks. The results could have broad applications in autonomous vehicles, surveillance and national defense.