Deep data inspection: The overlooked element in government data security
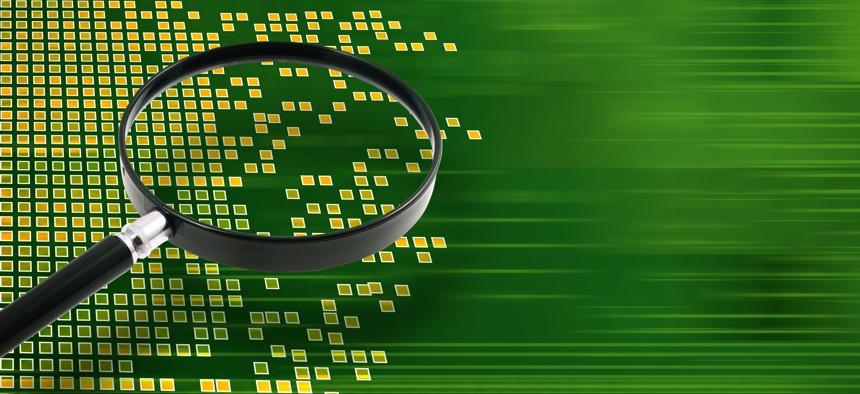
GettyImages/ dem10
Because encryption can make data quality problems harder to detect and isolate, good quality, properly formatted, exploit-free data is essential.
When people think about data security in government, they immediately think about encryption. And rightfully so: Encrypting data at rest and in motion has been a best practice for the past decade. In recent years, however, the data security arsenal has expanded to include what is becoming known as “deep data inspection.”
Deep data inspection goes one step deeper into data security and looks inside packaged data for threats and quality defects.
We've been trained to believe that security threats -- malicious or unintentional exploits --emerge as data is first created. What has been overlooked in many instances, however, is that data quality issues are actually an intrinsic part of data security.
Deep data inspection is analogous in many ways to network-based deep packet inspection. In the earliest days of the internet, information crossed the internet in clear text. As hacking became more common, IT managers concluded they needed to look inside individual network packets to determine whether the data contained in those packets was legitimate.
Today, data security is beginning to conduct deep data inspection on data files – especially those that fuel artificial intelligence and machine learning products that make sense of today’s enormous data warehouses.
A comprehensive data security strategy now must include both inspection and encryption – and, in fact, it makes the most sense to start with inspection. After all, if data is encrypted before it is inspected, it’s akin to locking the criminal inside the house, from a security perspective.
Consider the example of a comma-separated-value (CSV) file, similar to a spreadsheet. In the world of big data, these files can contain millions of rows and columns. Data files like these are typically encrypted because they must be protected as they move across the internet and are shared from one authorized user to the next. All that’s needed is an intentional or unintentional exploit in a single cell in one file for systems to be corrupted, crashed or taken over.
It’s essential, therefore, to be able to scan all those rows and columns to validate that not only are there no threats hidden in the data, but that the data itself is of good quality, properly formatted and ready for glitch-free AI modeling.
And let’s not underestimate the importance of data quality in AI modeling. Because of the massive size of data files, AI is an absolutely essential part of turning data into useable information for both internal and external customers. It is therefore also an essential aspect of the health and performance of the network itself.
Data doesn’t have to include malicious code to have a significant financial and operational adverse effect on AI models. Possible data corruption, incomplete data volumes, formatting errors, incomplete data or duplicate data are not only expensive from a storage and network management perspective; these problems also call into question the accuracy of AI modeling that makes big data useful to begin with. That’s why poor data quality can be as much of a network problem as security exploits.
Turning back to the larger issue, there’s a curious dilemma inherent in data security. Cloud service providers often underscore that security is a shared responsibility; providers are responsible for security of the cloud, and users are responsible for security in the cloud. Cloud providers commonly expect that customers will encrypt their data.
But data encryption makes it more difficult to judge the quality of data. Ironically, in many of the most secure environments in which I’ve worked, data encryption is regarded as a necessary evil at best, because of the limitations it puts on being able to examine data. If a data exploit has been proven, it becomes that much harder to find it once it has been encrypted.
None of this is to say that data shouldn’t be encrypted, but it’s important to be aware of the issues that emerge from data encryption that make exploits or data quality problems harder to detect and isolate.
A comprehensive data security strategy, therefore – especially as artificial intelligence becomes a commonplace method of analyzing and using data – must be rooted in an understanding that both deep data inspection and data encryption are essential to network health. And in terms of order, deep data inspection must come before data encryption to get the best value from data at rest or in motion.
Dave Hirko is founder and principal of Zectonal.