5 keys to getting big data under control
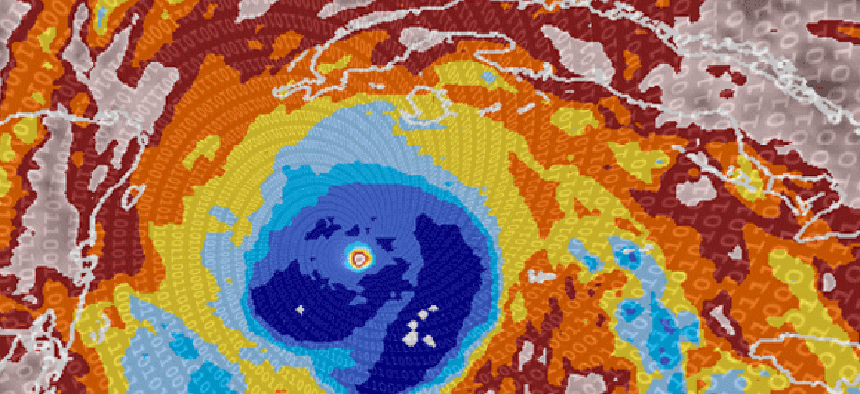
Connecting state and local government leaders
Commerce Department CIO offers insights on how to think about big data in ways that will keep its challenges down to size.
Agencies will face a lot of challenges with forthcoming big data projects, but one of them will not be generating enough data. In fact, consulting firm Gartner Inc. recently reported that enterprise data growth rates now average 40 percent to 60 percent annually.
At the Commerce Department — parent agency for some of the government’s biggest data producers, including the National Weather Service and the National Oceanic and Atmospheric Administration — those numbers might even be low.
“One thing that is not challenged is our ability to generate data,” Commerce CIO Simon Szykman said in remarks at the recent FOSE conference. “Our fundamental ability to generate data is growing tremendously and in many ways is outpacing our ability to process the data, to manage the data and to move it from one place to another.”
How to simply manage and move the agency’s data from one point to another is a big challenge.
Szykman also discussed some of the more important hurdles his department faces when working with big data. Here are five to keep in mind.
1. Data engineers
There are many scientists in the research community who are at work on sophisticated uses of big data, such as how to tap genome data in the fields of preventative medicine, drug design or pre-natal testing. What worries Szykman, though, is the limited number of people who really understand the technology infrastructures to support the use of big data in those areas.
“We need to give some thought to big data and how we’re going to work with it … particularly since in many cases, whether it’s a direct application in our agency or just providing funding to the research community, in one way or another, the government is driving the cutting edge of big data today,” he said.
2. Confidentiality vs. integrity
For agencies with a scientific or a research brief, big data security is more than a matter of confidentiality. Instead, the more critical concern is long-term data integrity.
“This is one of those things that in the IT world we struggle with,” Syzkman said. “Sometimes we aren’t always focused as much on security as we are in delivering results. People sometimes ask: ‘If we’re ultimately going to be sharing this data with the public, why is security so important?’”
The answer is best addressed by scientific agencies such as NOAA, which has been gathering benchmark data that is at the center of much of the debate about U.S. climate change policy.
“Those policy decisions have significant economic implications regardless of the political leanings,” Szykman said, including decisions about business regulation and investments. “If somehow the security of this long-term climate record were compromised, there would be far-reaching implications about it,” he said. “That’s something we need to worry about in the big data area.”
3. Think big, but plan early
In the move to open data, it has become more and more important to address requirements early in the system life cycle.
“One thing that has not happened in the past is looking at open data requirements very early on in the life cycle,” Syzkman said. “I think there’s going to be less ad hoc modeling, sharing, informing of data and more systematic strategies that look end to end,” he said. “Now at early life cycle stages when we’re putting in place new systems or new mission apps, this is going to be part of what gets addressed early on.”
4. Data authenticity
The importance of big data is not just the records created from the data; much of its value lies in the “reproduce-ability” of scientific results based on the data.
“From an academic perspective, that’s really how you prove the value of what you do: other people can reproduce the results as well,” Szykman said. On the other hand, he said, if you “lose the data on which research results are based, that undermines the legitimacy of some of those results over time.”
5. Laying down the baseline
Sometimes it’s difficult to assess the costs and risks of big data and other cutting-edge programs when few similar applications exist against which information can be drawn or comparisons made. Coming up with cost and risk baselines is a challenge for big data as well data centers, which have not been built with metrics in mind.
“The ability to do simple things like the measuring power consumption in the data center can be challenging if the data center is not constructed and instrumented to support that,” he said. “Baselining of big data — not just from the infrastructure perspective but also the data sets — needs to drive better planning for future resource planning,” he said.
NEXT STORY: Stealth releases fanless, silent mini PC