How MassHealth cut Medicaid fraud with predictive analytics
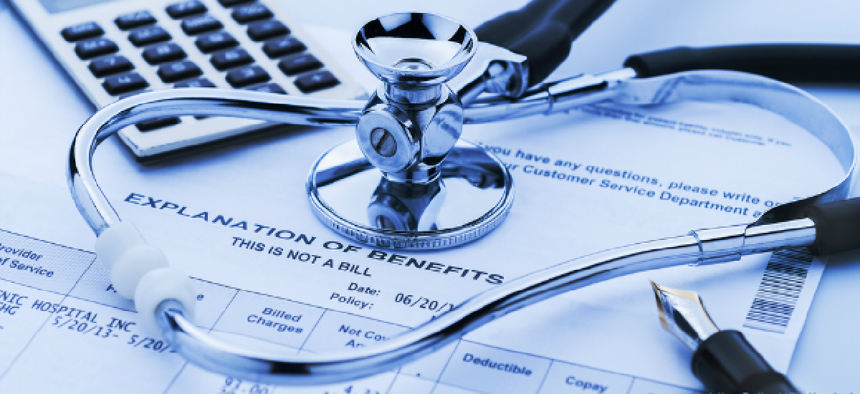
Connecting state and local government leaders
Predictive analytics system based on NetReveal fraud detection protects MassHealth from fraud and unnecessary expenditures.
Massachusetts is using predictive modeling to combat fraud in Medicaid payments, saving the Commonwealth from shelling out millions of dollars in false claims to health care providers.
The predictive analytics application supports Massachusetts' Executive Office of Health and Human Services fraud investigations by providing real-time risk assessment on health claims.
By shifting away from a "pay-and-chase" model, investigators have been able to recover $2 million in improper payments and have avoided paying hundreds of thousands of dollars in fraudulent claims during the first six months of operations, said Joan Senatore, director of the Massachusetts Medicaid Fraud Unit.
The system went live on May 27, 2013, with a pre-payment screening for claims that helps protect MassHealth from unnecessary expenditures and creates a more accurate reimbursement for billing providers, Senatore said.
Almost every state uses a pay-and-chase method for processing Medicaid and Medicare claims. After paying a claim, state investigators examine claims randomly, search for fraud patterns on their own or get tips from whistleblowers. If they detect fraud or waste, they have to prove fraud has been committed and then attempt to recover the money.
It's an inefficient process in which states often do not get back all of the money paid out in bad claims, said Kathy Baird, vice president and general manager of state and local programs with Dynamic Research Corp. (now a part of Engility), the systems integrator leading the predictive analytics project.
Most states have tools for analyzing claims that work with back-end IT systems, and MassHealth still uses those types of tools for post-payment analysis, Senatore explained. But state officials wanted to identify anomalies and patterns much earlier in the process, she said.
To better manage these cases and ensure compliance by providers, MassHealth used BAE Systems' NetReveal (formerly known as Detica NetReveal) fraud detection software that incorporates predictive analytics and social networking analysis to identify claims that could lead to improper payments.
NetReveal integrates with Massachusetts’ Medicaid Management Information System (MMIS) and analyzes public and other Commonwealth data stored in a data warehouse, automatically assessing risk scores associated with claims, Senatore said.
Investigators always had access to databases and other data sources, but now "we bring all data into one area," she said, such as the master death file and the Office of Inspector General's provider exclusion list.
"We download all files into the predictive model and can identify if someone has died or if a provider has been excluded by Medicaid and Medicare," Senatore explained. "These are things we do check on at the back end, but now we are checking them much sooner."
Within seconds after a claim passes through MMIS for verification, the predictive analytics system takes it and applies models to detect any anomalies. A claim can be denied right away, put on hold while investigators do further analysis or paid while the investigation is ongoing, DRC's Baird said.
Choose data sources carefully
To ensure that predictive models can accurately identify patterns and relationships between people and data, analysts must carefully choose which data sources to start with, Baird said. They should start out with small data sets and build analytics around them. Then, build models that can look for overlapping information.
For instance, a provider should not submit claims for in-home services and out-of-home services from the same client. Analysts might find that a health care provider has been doing this for 10 years, she said. Information gleaned from analytics can then be edited back into the main Medicaid system to prevent bad claims.
NetReveal's origins were in fraud detection for the financial sector. As a result, specialists who understood medical claims had to create detection models while technology experts configured the models for the IT systems, Baird explained. MassHealth officials also chose to keep the predictive analytics system within its own IT infrastructure along with MMIS rather than outsource anything to a cloud computing environment, she noted.
The Medicaid Fraud Unit had to build a team of investigators and analysts to move into the new area of predictive analytics. The team consists of five investigators and two additional analysts that work the data once an anomaly has been identified. The unit also refers cases to the post-payment review analysts to investigate.
MassHealth officials will continue to build on the system. "We are still in the infancy stage now," Senatore said. "We keep adding new detection scenarios identified through the system" as well as scenarios suggested by investigators and analysts, she said.
With more data coming in from outside data sources, the tool will have better information to identify changing regulatory and fraud-related issues. "Ideally, if we had Medicare data, it would be a lot better," Senatore said.
The analytics system is not a "be-all" tool for fraud, but it cuts down on time to analyze claims. "Hopefully, it will send a message to the provider community that we are looking right away, and that will deter the fraudulent claims coming in," Senatore said.
NEXT STORY: Report: Best practices for big data projects