The hidden dangers of big data tools
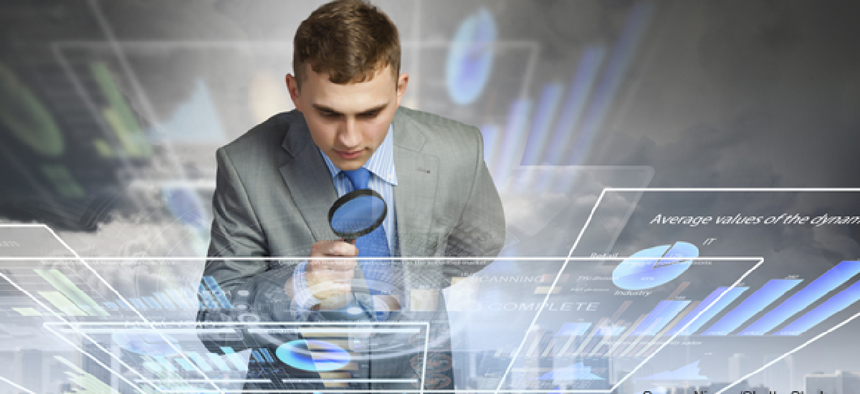
While big data tools are very powerful, the results they deliver tend to be only as good as the strategy behind their deployment.
When a new, powerful tool comes along, there’s a tendency to think it can solve more problems than it actually can. Computers have not made offices paperless, for example, and Predator drones haven’t made a significant dent in the annual number of terrorist acts.
To judge by the number of requests for proposals coming out of agencies and departments, the current power tool of choice is one that can apply analytic tools to massive amounts of data to find underlying, meaningful patterns. Government agencies, for example, are using big data tools to detect crime patterns and Medicaid fraud. The Department of Homeland Security is using big data tools to scan social media for signs of terrorists, and private companies are using similar tools to detect insider threats.
While big data tools are, indeed, very powerful, the results they deliver tend to be only as good as the strategy behind their deployment. A closer look at successful big data projects offers clues as to why they are successful … and why others fall short of the mark.
One of the most effective big data projects I’ve covered in recent years is the USDA Risk Management Agency's program to set crop insurance rates and to detect fraudulent claims of crop losses. The agency starts with FCI-33 rate maps created in ESRI ArcView that combine data from a variety of sources, including soil data from the Natural Resources Conservation Service, floodplain data from the Federal Emergency Management Agency, farm location and crop data from the Farm Services Agency, historic weather data and satellite imagery.
After assessing this data, agency analysts create zones that establish crop insurance rates for each parcel of land. The bonus is that when claims are made, the same system can be used to detect and investigate potentially fraudulent claims.
The RMA project has the characteristics that mark a solid big data project: A measurable, defined goal and the use of data that is measurable and clearly relevant to the goal.
The data going into the RMA's model – historic crop data, historic flood data, soil characteristics and weather patterns – are clearly related to the likelihood of crop damage. (At the same time, there may be factors overlooked by the model, so the model has to be continually tested for accuracy.)
So what happens if you take the tool and apply it to another task, say, analyzing patterns of student loan default rates? The first step is to figure out what data to collect that is relevant: student age, gender and socio-economic indicators; type of school (public, private, for-profit); geographic location.
The tool kicks out a result: student loan default rates are higher at for-profit schools than at other schools. But why? Does that mean those colleges aren’t doing a good job of preparing students for jobs? Or does it mean less-qualified students are going to those schools because they can’t get into more competitive, public universities? Or does it mean for-profit schools are training students for jobs that are lower paying or that are in sectors that aren’t hiring?
Big data tools get even more problematic when they are applied to such goals as ferreting out insider threats or terrorist activity. When the Department of Homeland Security scans social media for people talking about bombs and using racist or intolerant language, how does it distinguish between people who are likely to actually take action versus those who are just venting? Surely there are many more who fall in the latter category. How effective is such a big data tool if it sends agents out on wild goose chases?
What’s more, even if the sentiment analysis tools used by DHS are accurate enough to distinguish between someone who is a real threat and someone who is just venting or writing fiction, analysts need to consider the impact of the tool itself on what is being studied.
While the RMA’s project doesn’t affect the weather or soil characteristics, it can affect the behavior of people who may file false crop damage claims. Likewise, as it becomes known that law enforcement agencies – or corporations on the lookout for insider threats – are scanning social media, the behavior of those using social media is likely to change. And the very people those agencies are looking for are the people who are most likely to change their behavior, which means that the rate of “false positives” is likely to increase over time.
In short, the key to a successful big data project isn’t the bigness of the data or the slickness of the dashboard a given tool provides. It’s the quality of the selection and analysis of the data. Unfortunately, in many cases, those who use the big data tools may not even be aware of the underlying logic of the data selection and analysis.