Are agency researchers being misled by Twitter data?
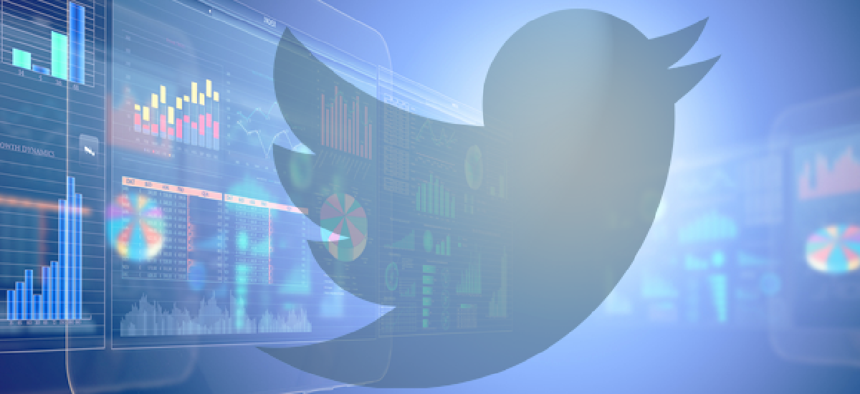
Connecting state and local government leaders
The use of social media as a public outreach and data collection tool is now actively promoted by government agencies. But do the data samples paint an accurate representation of public sentiment?
The FBI and the Department of Homeland Security are scanning social media to track criminals and potential terrorists. The Centers for Disease Control likewise monitors social media to track outbreaks and spread of diseases. And other federal agencies are analyzing social media to monitor public sentiments about agency projects and services.
The use of social media as a public outreach tool and source of user data is now actively promoted by the General Services Administration's DigitalGov platform. Users interested in taking advantage of social media can access the developing Federal Social Media Metrics Toolkit by joining (or even just visiting) the Federal Social Media Community of Practice at DigitalGov.
What you won't find at DigitalGov – or at least I couldn't find it – is warnings about the ease of being misled by data collected from social media.
According to Juergen Pfeffer, assistant research professor at Carnegie Mellon University's Institute for Software Research, while social media delivers immense amounts of data, it's often not clear what that data means and who it is coming from.
"Are the people on Twitter or Pinterest representative of the population that we want to analyze?" asked Pfeffer. "Some groups are overrepresented and others are not, especially when we analyze critical questions like how people vote. It turns out it's pretty difficult to use information that people give on Twitter to generalize."
Let's assume, posed Pfeffer, that you collect millions of tweets over time to analyze how people feel about ISIS, the Islamic State in Iraq and Syria, after news coverage of beheadings staged by the group.
"You collect your 25 million tweets from March to December 2014, you count the tweets, you most likely look at some keywords that show up, you count the proportion of English vs. Arabic tweets, you try to do some text analysis," Pfeffer said.
"You count the number of positive vs. negative adjectives, you put this on a line chart and you say, 'Oh, look, the emotions of people in the Western world go down but in the Arabic world they go up.' This is a typical study. I guess they're probably 250 companies doing this right now while we are speaking."
But according to Pfeffer, there are a lot of assumptions buried in such an analysis.
"When you do over-time analysis, there's a good chance there are different people in your sample," he noted. "Maybe in March and April it is only insiders who discuss ISIS. And starting with the beheadings, the general population starts to discuss it. So you're comparing completely different samplings of the population with each other."
What's more, in many cases, when a company or a government agency subscribes to data feeds from social media, the feeds may have been massaged using algorithms that the subscriber can't analyze.
Pfeffer added that models in use for detecting the political sentiments of people on social media currently work well when the people using that media are activists, because they use jargon and language that makes it easier to detect their positions.
"But if you use the same model to analyze non-activists it doesn't work as well," he said. "In fact, it doesn't work at all."
While most professional pollsters and social scientists are aware of these sampling and data context issues, many of those analyzing social media data are not.
Accordingly, in a commentary published in the Nov. 28 issue of the journal Science, Pfeffer and Derek Ruths, an assistant professor of computer science at McGill University, argue that information professionals need to do a better job of correcting implicit bias in data collection via social media.
"Our main contribution is to increase awareness regarding these questions of what is actually being analyzed when we look at this data," Pfeffer said.
The bottom line is that big data research often has an air of authority it hasn’t earned. "The moment you talk about 50 million tweets it sounds like you have the truth," said Pfeffer. "I'd like to see more awareness of these issues of bias, starting with the data collection."
NEXT STORY: Federal Laboratory Consortium launches tech transfer tool