Spark fires up near-real-time big data
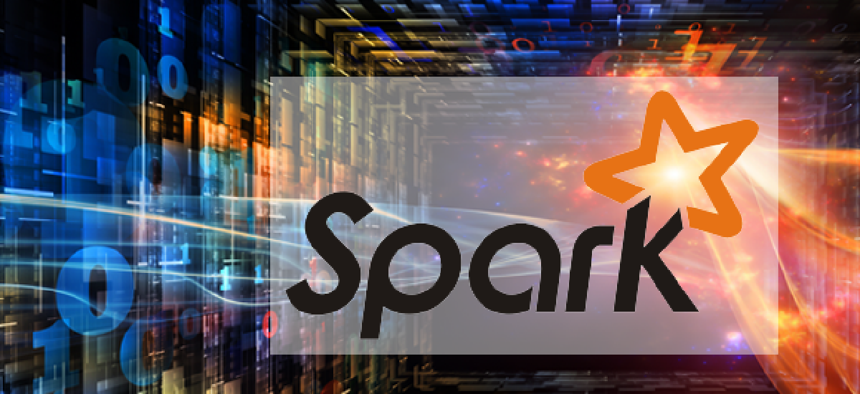
Connecting state and local government leaders
Rapid in-memory processing of distributed data is the “core capability” of Apache Spark, which enables the analysis of streaming big data.
With the “next big thing” in IT there inevitably comes a time when user experience falls short of the hype. So it is with big data and its promise of fast and precise analysis of huge volumes of distributed data.
In the current big data universe, Hadoop is the software used to store and distribute large amounts of data and MapReduce is the engine used to process it. The combination has proven itself in non-time critical, batch processing of data.
But what about analysis of near real-time big data? Apache Spark, the most advanced of these next-generation, open source technologies, sets the stage for analysis of streaming data from video, sensors and transactions as well as for machine learning and predictive modeling. It enables genomics research, packet inspection, malware detection and the Internet of Things.
Like MapReduce it can be used for batch processing, but for those algorithms that perform a number of interactions on a dataset, Spark can store the intermediate results of those actions in cache memory. MapReduce, in contrast, has to write the result of each action to disk before it can be brought back into the system for further processing.
That rapid in-memory processing of resilient distributed datasets (RDDs) is the “core capability” of Apache Spark.
“Once operations are done (on the datasets) they can be streamed and connected to each other so that transformations can be made very quickly,” said Dave Vennergrund, director of predictive analytics for Salient Federal Solutions, which is working on developing analytics products for government organizations using Spark.
“Couple that with the ability to do this across many machines at the same time, and you have a recipe for a very strong response,” he added.
Proponents of Spark claim both scale and speed advantages for Apache tool compared to its competitors. It’s been shown to work well for small datasets up to volumes measuring in the petabytes.
A November, 2014, benchmark contest had Apache Spark sorting 100 terabytes of data three times faster than Hadoop MapReduce, on a machine cluster one-tenth of the size of that used for the MapReduce sort.
A recent survey by Typesafe, a software developer, showed a rising level of interest by organizations in using Spark.
Only 13 percent were currently using it, but over 30 percent were evaluating it, a fifth of the respondents were planning to begin using it sometime this year, and another 6 percent expected to use it in 2016 or later. However, 28 percent of those surveyed also had no knowledge of Spark, which emphasizes its still “bleeding edge” status.
For the government space, “testing and evaluation is where it’s at right now,” said Cindy Walker, vice president of Salient’s Data Analytics Center of Excellence. Agencies that have “sandboxes and R&D budgets” are the early adopters, she said.
“Many of our customers aren’t yet signing on the bottom line to implement big data, in-memory analytics, streaming solutions,” she said. “So, at this time, we are using Spark to help guide them to what they can expect once they get to that point.”
So while Spark won’t be a replacement for MapReduce, it will eventually claim a section of the big data analytics spectrum devoted to speedy data processing, according to analysts.
The Apache Spark ecosystem comprises several integrated components:
Spark Core, the underlying execution engine for the platform, supports a range of applications, as well as Java, Scala and Python application programming interfaces (APIs).
Spark SQL (structured query language), with which users can explore data.
Spark Streaming enables analysis of streaming data from sources such as Twitter. This is in addition to Spark’s ability to also do batch processing.
Machine Learning Library (MLlib), a distributed machine learning framework, delivers high-quality algorithms up to 100 times faster than MapReduce.
Graph X helps users build and manipulate graph-based representations of text and tabular data to find various relationships within the data.
SparkR, a package for the R statistical language with which R users can use Spark functionality from within the R shell.
BlinkDB, a massively parallel engine that allows users to run “approximate” SQL queries on large volumes of data, useful in situations where speed is more important than absolute accuracy.
NEXT STORY: Navy’s intell requires automated analytics