It's data science, not rocket science
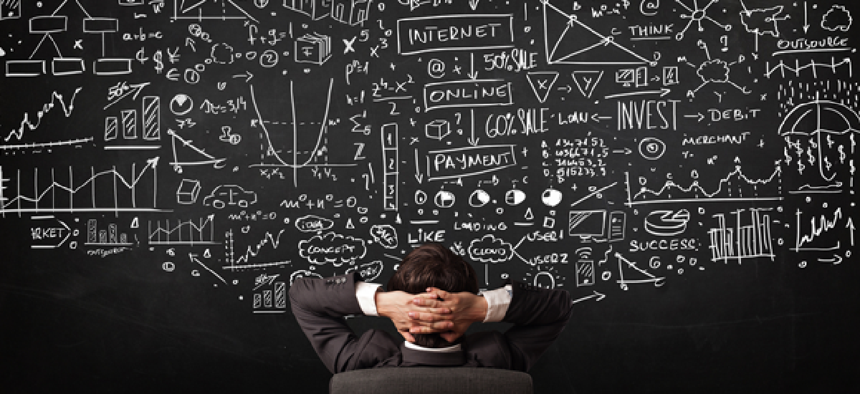
Connecting state and local government leaders
7 steps to a successful data science strategy.
Data scientists and chief data officers are the hot hire these days, and government agencies at all levels are working to get more out of their rapidly growing troves of data. Determining how to approach all that data, however, can be daunting.
For agencies that don't have DJ Patil on speed dial, a recent TDWI report offers a good place to start. (TDWI, like GCN, is owned by 1105 Media.) "Seven Steps for Executing a Successful Data Science Strategy" is just what the title suggests -- a checklist of seven basic tenets of smart and results-oriented data science.
"To solve business problems, develop new products and services and optimize processes," TDWI's Dave Stodder wrote, "organizations increasingly need analytics insights produced by data science teams with a diverse set of technical skills and business knowledge who are also good communicators." To make the most of such investments, the report recommends:
1. Identify your key business drivers for data science
Before getting started, an organization must ask what real data science efforts can provide that traditional business intelligence and analytics are not. If there are gaps, it's critical to hire personnel with real "knowledge of and curiosity about the business" to help fill them.
2. Create an effective team
It takes more than curiosity, however. And hiring a multitalented superstar -- which is "like chasing unicorns" to begin with -- can leave an agency with a one-off, artisanal operation whose creator then leaves for greener pastures. "[A] wiser course is to develop a stable team that brings together the talents of multiple experts."
3. Emphasize communications skills
"Organizations that use data science successfully almost universally point to communication as a key ingredient to their success," TDWI found. Organizations should "make it a priority as they evaluate candidates for data science teams."
4. Expand the impact through visualization and storytelling
"Data science thrives in an analytics culture," Stodder wrote, but "not all personnel... are going to be part of data science teams, nor should they be." Finding ways to help non-statisticians grasp the insights in the data is critical to getting real value out of the investment.
5. Give the data scientists all the data
While traditional analytics often focus on a carefully defined set of structured data, data science has the potential to draw value from the vast messes of unstructured data that most organizations create. "Data scientists need to work closely with data at every step so they know what they have," Stodder wrote -- and they need to have as much of it as possible.
6. Pave the way for operationalizing the analytics
Descriptive analytics are useful, but predictive analytics are far more valuable -- and prescriptive analytics offer the most potential benefit by far. To make this possible, "data science teams can move away from uncoordinated, artisanal model development and toward practices that can include quality feedback sessions to correct flaws."
7. Improve governance to avoid data science "creepiness"
Both data science teams and top leadership "must be cognizant of the right balance between what they can achieve ... and what is tolerable -- and ethical -- from the public's perspective."
For the full report, go to TDWI.org.