Can big data predict violent behavior?
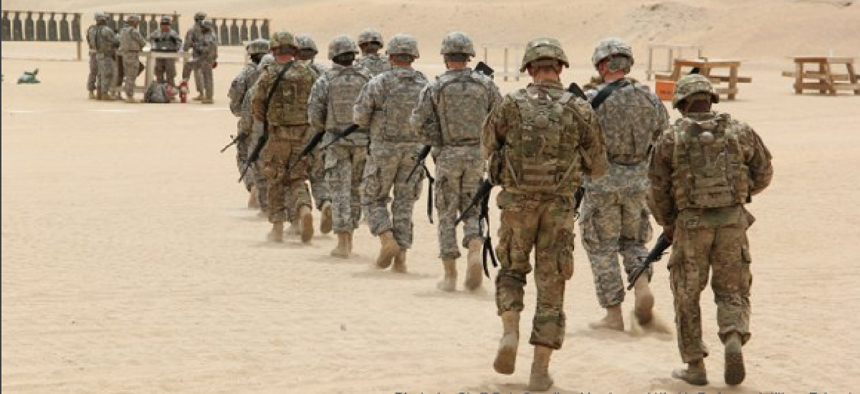
A team from Harvard Medical School is applying machine learning models to U.S. Army personnel datasets to predict violent behavior.
Using machine-learning to analyze over three terabytes of data on military personnel, researchers at Harvard Medical School were able to predict the 5 percent of U.S. Army soldiers who later committed one-third of all violent crimes in the workplace between 2004 and 2009. When analyzing data from 2011 to 2013, the machine-learning model was even more accurate, predicting the 5 percent of soldiers that would commit 50.5 percent of violent crimes.
That research, sponsored by the Department of Defense, fused data from several military datasets, including the Army’s Study to Assess Risk and Resilience in Servicemembers.
The team created a consolidated dataset that pulled data from many different military datasets, including crime data, deployment data and the soldiers’ electronic medical records, said Ronald Kessler, professor of health care policy at HMS and the principal investigator on the project.
According to Kessler, simply getting the data ready for analysis was a major part of the job. “With these big data jobs, 85 percent of the work is usually data management and the other 15 percent is the data analysis,” he said. In fact, the project had four people working for three to four years just preparing the data. When different agencies and offices have collected data over decades, Kessler noted, “variables and names get changed.” And the people who originally structured the data are either gone or have forgotten how it was put together.
“Now it’s a little more organized,” he added. “We have a couple of people who do only data management – updating the data, cleaning the data – and who don’t do any statistical analysis at all.”
As for the statistical analysis, the massive amount of data itself presents a challenge. The project is not interested just in soldiers’ personal data and crime data; it also takes note of when things happen. Accordingly, the unit of analysis is a “person-month.” With data from both 975,000 regular Army soldiers and 750,000 National Guard and Reserves collected over five years, that means 32 million person-months in the project file. “And in each of those 32 million person-months, we have a couple thousand variables,” said Kessler. “So for a given year, it’s 32 million records, times 2,000 variables, times 12 months.”
And each variable changes not only with each individual, but over time. “Did you just get demoted?” Kessler asked, rhetorically. “Being demoted could be a risk factor, but it’s not a risk factor for the rest of your life. It’s maybe only a risk factor in the month after a demotion. That’s the highest risk, but then it goes down with time.”
The specific machine-learning algorithms applied to the data depend on a number of factors. According to Kessler, there are more than 50 algorithms available and none of them are appropriate for every application, “so the issue is selecting the right one for an application.”
“You have such an enormous number of variables, you’ll always find something that predicts anything,” said Kessler. “The question is how stable it is.”
Accordingly the team had to do a great deal of cross-validation. “It takes a lot of computer time,” he noted. “It might take three or four days for something to converge.”
What the project is finding at this stage, Kessler stressed, is correlation, not causation. “If we discover that being a left-handed midget is a significant predictor of suicide – which, by the way, it’s not – then that’s in the model, as long as it’s a stable predictor,” he said. “We’re trying to be agnostic as to what causes what.”
It’s important to be cautious in interpreting causal links, Kessler stressed. “We’ve tried to play down the importance of any one predictor.”
Even within the 5 percent of soldiers at highest risk for committing violent crimes, there could be three or four subgroups. A 17-year-old who came into the Army from a bad family environment and a soldier with 28 years of service who had never been married and who was involuntarily discharged may both be at risk, he said, but “the issues for those two people are very different.”
Knowing how those subgroups are different through sharpening the understanding of causal links is the next step in the project. “We want to be able to help the clinicians identify those at most risk,” said Kessler.
The project will move beyond assessing risk for violence within the military. In the future, for example, Kessler said he expects to apply the methods being developed to finding appropriate treatments for depression. “With depression, there’s no one kind of treatment that works best for every person,” he said. “Can we, without having to go through long trial and error, figure out which kinds of things are going to work for which kinds of patients?”
That would be especially valuable, since he noted that part of the problem with depression is that individuals give up on treatments easily when they don’t produce results. “The vast majority of patients will be helped eventually by some treatment,” Kessler said. “We’re trying to develop the same kind of complicated models [as in the military project] to use information about the individual to pick the best bet for a treatment.”
NEXT STORY: Army gets a GRRIP on remote communications