Can crowdsourcing decipher the roots of armed conflict?
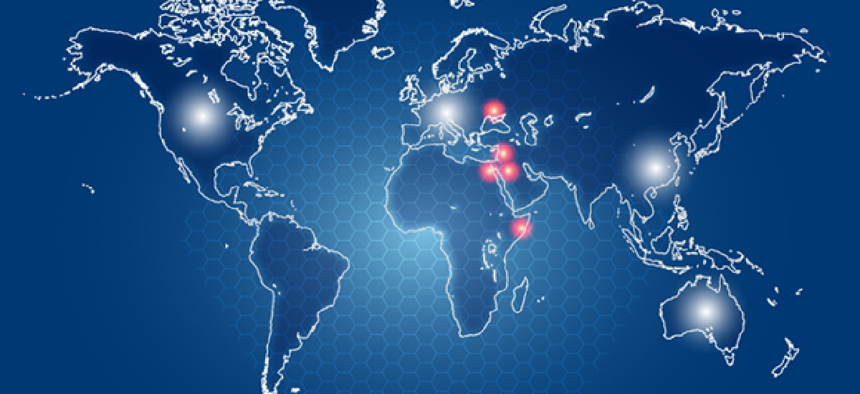
The Correlates of War project, a long-standing project that studies the history of warfare, is now experimenting with crowdsourcing as a way to improve its global conflict database.
Researchers at Pennsylvania State University and the University of Texas at Dallas are proving that there’s accuracy, not just safety, in numbers. The Correlates of War project, a long-standing effort that studies the history of warfare, is now experimenting with crowdsourcing as a way to more quickly and inexpensively create a global conflict database that could help explain when and why countries go to war.
The goal is to facilitate the collection, dissemination and use of reliable data in international relations, but a byproduct has emerged: the development of technology that uses machine learning and natural language processing to efficiently, cost-effectively and accurately create databases from news articles that detail militarized interstate disputes.
The project is in its fifth iteration, having released the fourth set of Militarized Dispute (MID) Data in 2014. To create those earlier versions, researchers paid subject-matter experts such as political scientists to read and hand code newswire articles about disputes, identifying features of possible militarized incidents. Now, however, they’re soliciting help from anyone and everyone -- and finding the results are much the same as what the experts produced, except the results come in faster and with significantly less expense.
As news articles come across the wire, the researchers pull them and formulate questions about them that help evaluate the military events. Next, the articles and questions are loaded onto the Amazon Mechanical Turk, a marketplace for crowdsourcing. The project assigns articles to readers, who typically spend about 10 minutes reading an article and responding to the questions. The readers submit the answers to the project researchers, who review them. The project assigns the same article to multiple workers and uses computer algorithms to combine the data into one annotation.
A systematic comparison of the crowdsourced responses with those of trained subject-matter experts showed that the crowdsourced work was accurate for 68 percent of the news reports coded. More important, the aggregation of answers for each article showed that common answers from multiple readers strongly correlated with correct coding. This allowed researchers to easily flag the articles that required deeper expert involvement and process the majority of the news items in near-real time and at limited cost.
“In terms of this being more cost-effective, it’s a night-and-day difference,” said David Reitter, an assistant professor at Penn State’s College of Information Sciences and Technology. “Paying two or three [experts] for a few months will cost you a lot of money, but paying people on a per-document basis – and we pay about $1 per document” is much less expensive.
And in some cases, human readers are being removed from the equation entirely. “We will have technology that basically combines crowdsourcing and natural language processing so that certain sources that cannot be given to crowd workers can be analyzed by natural language processing … where we can extract information that is useful,” Reitter said. “We’re looking at algorithms that can continue to learn over time and react to a changing environment.”
The project bases its natural language learning on the semi-supervised deep neural networks of the brain in order to create a computer program that can learn well. Reitter likened semi-supervised learning to how babies develop, taking cues about what’s violent or not from direct words such as “hitting hurts” and from environmental context. In this way, the computer programs must be given a few examples of what descriptions of violence look like in an article, but the algorithms also begin to pick up on those cues on their own.
“Our challenge really is now to get the quality to the point where it’s good enough to form this dataset,” Reitter said.
The project began in 1963 and covers data from 1816 to 2010. An award of about $1 million from the National Science Foundation late last year helped fund the latest development of the algorithms and a dataset for the period 2011 to 2017. NSF also awarded the project more than $62,000 in 2000.
Although Reitter sees potential for the resultant technology in areas of government not necessarily related to conflict, the use of crowdsourcing to digitize information is not new in the public space. The National Archives and Records Administration invites the public to transcribe documents in the National Archives Catalog, for example. Among the records available for transcription is a letter dated Aug. 8, 1793, from Chief Justice John Jay to President Washington.
Additionally, NARA was part of a group that launched the Federal Crowdsourcing and Citizen Science Toolkit in September 2015 to help other federal agencies start crowdsourcing initiatives. The toolkit was developed and launched by the Federal Community of Practice on Crowdsourcing and Citizen Science, a group of more than 35 agencies that meet regularly to share lessons learned and develop best practices.
Editor's note: This article was changed Jan. 14 to correct NARA's role in the development of the Federal Crowdsourcing and Citizen Science Toolkit.