We use big data to sentence criminals. But can the algorithms really tell us what we need to know?
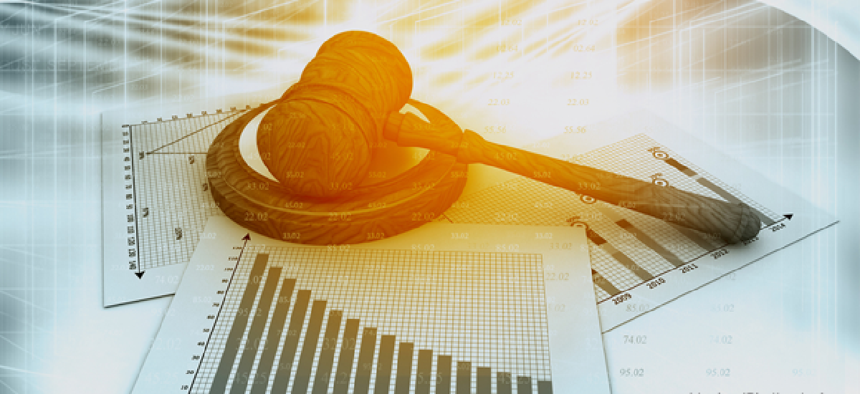
Connecting state and local government leaders
Data-driven tools cannot make the individual predictions that sentencing decisions require.

This article first appeared on The Conversation.
In 2013, a man named Eric L. Loomis was sentenced for eluding police and driving a car without the owner’s consent.
When the judge weighed Loomis’ sentence, he considered an array of evidence, including the results of an automated risk assessment tool called COMPAS. Loomis’ COMPAS score indicated he was at a “high risk” of committing new crimes. Considering this prediction, the judge sentenced him to seven years.
Loomis challenged his sentence, arguing it was unfair to use the data-driven score against him. The U.S. Supreme Court now must consider whether to hear his case -- and perhaps settle a nationwide debate over whether it’s appropriate for any court to use these tools when sentencing criminals.
Today, judges across the U.S. use risk assessment tools like COMPAS in sentencing decisions. In at least 10 states, these tools are a formal part of the sentencing process. Elsewhere, judges informally refer to them for guidance.
I have studied the legal and scientific bases for risk assessments. The more I investigate the tools, the more my caution about them grows.
The scientific reality is that these risk assessment tools cannot do what advocates claim. The algorithms cannot actually make predictions about future risk for the individual defendants being sentenced.
The basics of risk assessment
Judging an individual defendant’s future risk has long been a fundamental part of the sentencing process. Most often, these judgments are made on the basis of some gut instinct.
Automated risk assessment is seen as a way to standardize the process. Proponents of these tools, such as the nonprofit National Center for State Courts, believe that they offer a uniform and logical way to determine risk. Others laud the tools for using big data.
The basic idea is that these tools can help incapacitate defendants most likely to commit more crimes. At the same time, it may be more cost-effective to release lower-risk offenders.
All states use risk assessments at one or more stages of the criminal justice process -- from arrest to post-prison supervision. There are now dozens of tools available. Each uses its own more or less complicated algorithm to predict whether someone will reoffend.
Developers of risk assessment tools usually follow a common scientific method. They analyze historical data on the recidivism rates of samples of known criminals. This helps determine which factors are statistically related to recidivism. Characteristics commonly associated with reoffending include a person’s age at first offense, whether the person has a violent past and the stability of the person’s family.
The most important predictors are incorporated into a mathematical model. Then, developers create a statistical algorithm that weighs stronger predictors more heavily than weaker ones.
Criminal history, for instance, is consistently one of the strongest predictors of future crime. Thus, criminal history tends to be heavily weighted.
The tool typically divides results into different categories, such as low, moderate or high risk. To a decision-maker, these risk bins offer an appealing way to differentiate offenders. In sentencing, this can mean a more severe punishment for those who seem to pose a higher risk of reoffending. But things are not as rosy as they may appear.
Individualizing punishment
In the Loomis case, the state of Wisconsin claims that its data-driven result is individualized to Loomis. But it is not.
Algorithms such as COMPAS cannot make predictions about individual defendants, because data-driven risk tools are based on group statistics. This creates an issue that academics sometimes call the “group-to-individual” or G2i problem.
Scientists study groups. But the law sentences the individual. Consider the disconnect between science and the law here.
The algorithms in risk assessment tools commonly assign specific points to different factors. The points are totaled. The total is then often translated to a risk bin, such as low or high risk. Typically, more points means a higher risk of recidivism.
Say a score of 6 points out of 10 on a certain tool is considered “high risk.” In the historical groups studied, perhaps 50 percent of people with a score of 6 points did reoffend.
Thus, one might be inclined to think that a new offender who also scores 6 points is at a 50 percent risk of reoffending. But that would be incorrect.
It may be the case that half of those with a score of 6 in the historical groups studied would later reoffend. However, the tool is unable to select which of the offenders with 6 points will reoffend and which will go on to lead productive lives.
The studies of factors associated with reoffending are not causation studies. They can tell only which factors are correlated with new crimes. Individuals retain some measure of free will to decide to break the law again, or not.
These issues may explain why risk tools often have significant false positive rates. The predictions made by the most popular risk tools for violence and sex offending have been shown to get it wrong for some groups over 50 percent of the time.
A ProPublica investigation found that COMPAS, the tool used in Loomis’ case, is burdened by large error rates. For example, COMPAS failed to predict reoffending in one study at a 37 percent rate. The company that makes COMPAS has disputed the study’s methodology.
Deciding on Loomis
Unfortunately, in criminal justice, misinterpretations of risk assessment tools are pervasive.
Based on my analysis, I believe these tools cannot, scientifically or practically, provide individualized assessments. This is true no matter how complicated the underlying algorithms.
COMPAS documents state the results should not be used for sentencing decisions. Instead, it was designed to assist in supervisory decisions concerning offender needs. Other tool developers tend to indicate that their tool predicts risk at a rate better than chance.
There are also a host of thorny issues with risk assessment tools incorporating, either directly or indirectly, sociodemographic variables, such as gender, race and social class. Law professor Anupam Chander has named it the problem of the “racist algorithm.”
Big data may have its allure. But, data-driven tools cannot make the individual predictions that sentencing decisions require. The Supreme Court might helpfully opine on these legal and scientific issues by deciding to hear the Loomis case.
NEXT STORY: GCN founder Izzy Feldman dies at 86