DARPA tunes machine learning to radio signals
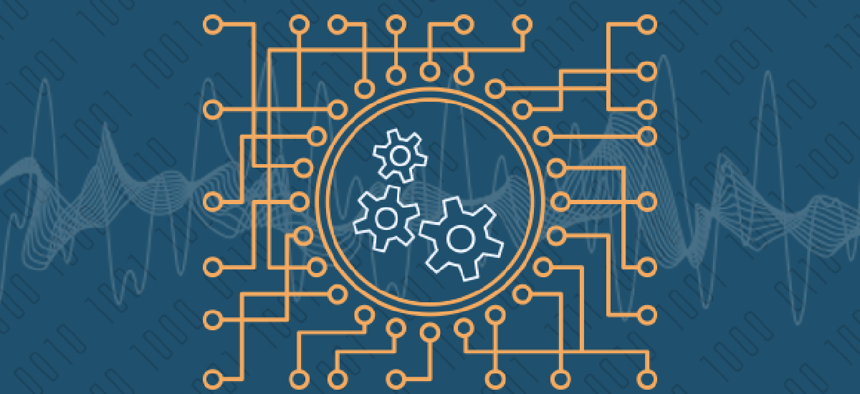
Connecting state and local government leaders
By applying machine learning to the radio spectrum, the research agency hopes to give the military situational awareness in the wireless domain.
The Defense Advanced Research Projects Agencies is looking to apply the same kind of machine learning to the radio spectrum as is used by advanced systems for applications ranging from voice recognition to management of internet-of-things devices to autonomous vehicles.
DARPA has issued a broad agency announcement for a new Radio Frequency Machine Learning Systems (RFMLS) program that will address the need for enhanced situational awareness regarding the ever-changing composition of RF signals in the IoT and spectrum sharing.
Machine learning is widely used to manage data and images, but the similar work in the radio spectrum offers unique challenges, making a more compelling case for developing a native approach.
“What I am imagining is the ability of an RF machine learning system to see and understand the composition of the radio frequency spectrum – the kinds of signals occupying it, differentiating those that are ‘important’ from the background, and identifying those that don’t follow the rules,” said DARPA’s Microsystems Technology Office Program Manager Paul Tilghman.
An RFMLS would be able to discern subtle differences in the RF signals among identical, mass-manufactured IoT devices and identify signals intended to spoof or hack into these devices. “We want to … stand up an RF forensics capability to identify unique and peculiar signals amongst the proverbial cocktail party of signals out there,” Tilghman said.
The program features four technical components that would integrate into future RFML systems:
Feature learning: From RF training data, RFML systems would be able to learn how to identify and characterize signals in various civilian and military settings.
Attention and saliency: An RFMLS would be able to identify, recognize and prioritize important visual and auditory stimuli.
Autonomous RF sensor configuration: The RFMLS would be able to optimize the huge number of possible configurations (analog electronics, beam steering angle, bandwidth sensitivity, position, etc.) to create the RF data required to improve overall task performance.
Waveform synthesis: A full RFMLS also should be able to learn or create new waveforms to achieve a task.
“If we get this right, we will have RF systems with the ability to discern and characterize signals in the ever-more-crowded spectrum,” Tilghman said. “And that will give emerging automated systems, and the military commanders that rely on them, much needed information to understand the landscape of the wireless domain.”
NEXT STORY: A back-to-basics stand-in for GPS navigation