Predictive policing shows promise in Chicago
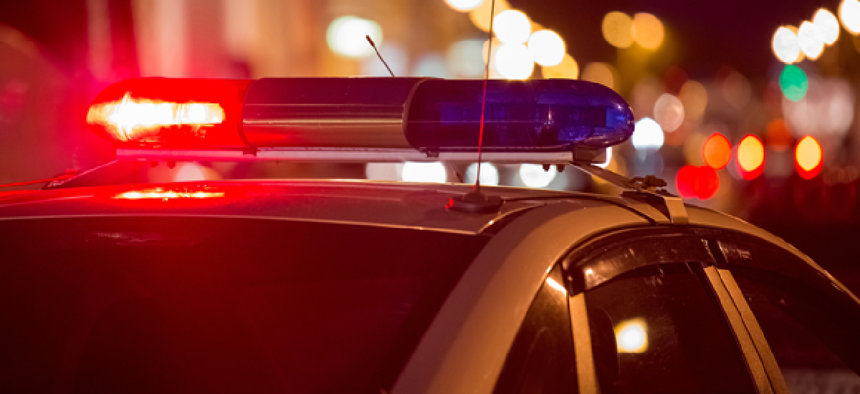
Connecting state and local government leaders
Districts where predictive software is fully operational have experienced fewer shootings and homicides.
As police departments across the country experiment with predictive policing technologies, at least one city is seeing some progress.
In Chicago, where city’s overall murder rate has gone up 3 percent, the districts that have implemented the predictive technology have seen a drop in the number of shootings and homicides, according to Reuters.
Three districts saw between 15 percent and 29 percent fewer shootings, and 9 percent to 18 percent fewer homicides, according to Reuters’ analysis of department data. And the 7th District saw a 39 percent drop in shooting in the first seven months of 2017 when compared to the same period last year.
One of the tools Chicago police are using is HunchLab, predictive policing software based on risk terrain modeling, according to The Verge. It takes many different variables into consideration for its model, including crime statistics, bar locations, weather conditions and even lunar phases. These risk factors are mapped to a grid of the city so police can determine where to place their resources.
The use of these predictive policing tools has raised concern with some activists. Organizations like the American Civil Liberties Union say that predictive policing tools will only perpetuate bias within policing. If specific communities have been more heavily policed in the past, then using historic data could show those communities have higher risk.
St. Louis County Police Department took these concerns into consideration when implementing HunchLab by getting the software to surface predictions of serious felonies rather than low-level crimes like drug possession, The Verge reported.
To help project managers and developers mitigate the unintended bias in the algorithms behind predictive programs, the Center for Democracy & Technology has released a digital decisions tool.
The digital decisions tool "translates principles for fair and ethical automated decision-making into a series of questions that can be addressed during the process of designing and deploying an algorithm,” CDT Policy Analyst Natasha Duarte explained in a blog post.
The questions address what data developers use to train an algorithm, the factors or features in the data they should consider, how to test the algorithm and how to ensure fairness.