Controlling cross-border traffic without sacrificing security
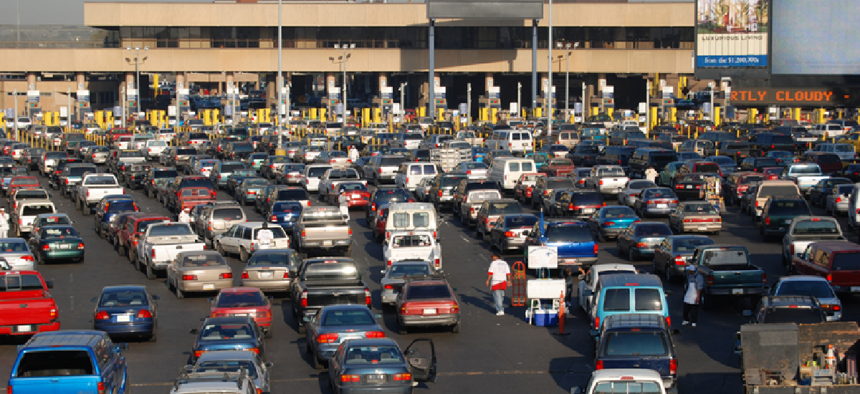
Connecting state and local government leaders
Combining data analytics with machine learning can help border security agencies ensure safe passage across land, air and sea.
“A needle in a haystack” is the phrase that comes to mind when thinking about securing international borders. The border security agent’s work gets more difficult every day with growing threats from terrorism and drug and human trafficking -- not to mention the increasing number of international travelers and cargo shipments. But advanced data analytics and machine learning offer all-new tools for border agencies to keep the lines moving quickly for law-abiding travelers and shipments, while clamping down on those that are not.
According to the World Tourism Organization, more than 2.5 billion people travelled internationally in 2017. Meanwhile, international air freight is experiencing annual double-digit growth. Most international travelers are vacationing or traveling for business. Fewer than one in 1,000 travelers and shipments may pose a threat, so the border agent's challenge really is like finding a needle in a haystack.
Worse, a border officer has just two seconds to decide whether to grant or deny clearance to cross the border.
While most border agencies use computerized targeting tools to help them determine which people or cargo shipments might be dangerous or illegal, those tools employ simple pattern matching to detect threats based on historical experience. For instance, an agency may determine that drug smugglers typically buy their plane tickets within 24 hours of traveling; pay in cash; and take a long route that involves transiting a third country. If this pattern is encoded as a rule, the targeting system automatically flags travelers who exhibit those same behaviors. It sounds good, but in reality, it’s like driving a car by only looking in the rear-view mirror – the system will never be able to detect new threats (or even old threats that change their patterns). As a result, pattern-matching systems tend to be highly inaccurate -- with up to 90 percent of flagged travelers and shipments turning out to be false positives. These false alarms waste border agency resources, delay the clearance of legitimate travelers and shipments and frustrate front-line border officers.
In an attempt to make pattern-matching tools more accurate, border agencies continually create new rules and revise old ones based on new observations. But they are always playing catch-up with criminals who change their patterns to avoid detection. And over time, the systems themselves become unmanageable, with thousands of overlapping, contradictory and out-of-date rules.
The targeting solutions used by most border agencies today fail to detect some threats while erroneously flagging far too many others. That’s a real problem considering the significant threats and growing international travel volumes.
A new targeting approach, using statistical analysis and data analytics, can deliver the improved accuracy that eludes pattern-matching tools. By detecting anomalies in the data, in addition to known patterns, statistical analysis identifies patterns that may represent a new threat. In essence, statistical analysis lets the data itself determine what to look for -- rather than depending on humans to define what the computer should look for.
With the addition of machine learning, the targeting system can learn from experience. If a border agent inspects a cargo shipment flagged as potentially risky and finds nothing, that result goes back into the system, and the targeting algorithms are automatically updated to be more accurate the next time.
By continually assessing travelers and cargo based on all information available at the time, agents can detect suspicious activity before bad actors even pack their bags for the airport. The system could start analyzing data from when a ticket is booked, for example, building a risk profile and allowing border agencies to investigate potential risks or take preventive actions. These new capabilities constitute a paradigm shift in border security measures and methods.
Combining the power of analytics with machine learning can maximize efforts of today’s border security agencies to ensure safe passage across land, air and sea.
NEXT STORY: DNA: Nature's data center