An analytics engine for personalized emergency response
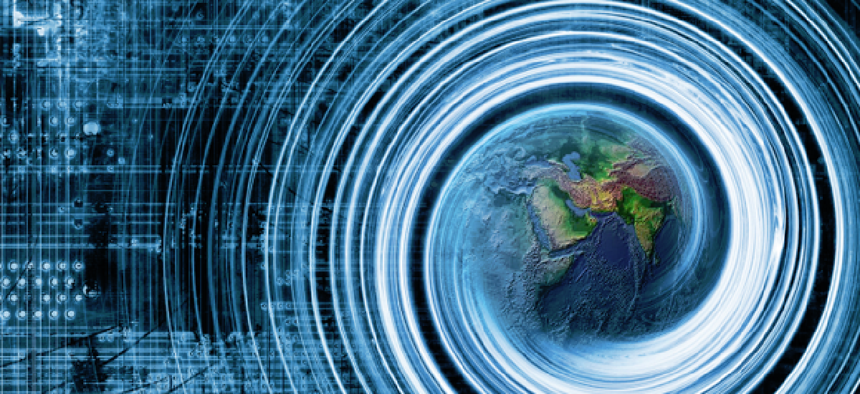
Connecting state and local government leaders
The synthetic information platform organizes large-scale interacting datasets so policymakers can predict how people will respond in a disaster.
Ever wonder what you should do immediately after a terrorist attack? Analytics can tell you.
The synthetic information platform developed by researchers at Virginia Tech’s Network Dynamics and Simulation Science Laboratory provides the kind of policy informatics required to respond to an emergency. The platform can simulate myriad scenarios, including terrorist attacks, natural disasters and disease outbreaks, and determine how each individual in the affected area will respond.
In an epidemic, for example, no single agency or leader has access to all the information and must rely on input from of a network of stakeholders who can be scattered across the globe and have limited ability to communicate with each other.
VT’s platform takes data on a large number of people acting and reacting to various situations and connects those dots to provide insight into their actions before and after a catastrophic event, giving policy makers models for forecasting and experimentation.
“This platform allows you to organize this diverse mesoscopic, massively interacting information and do things with it in a very large scale,” said Chris Barrett, director of Virginia Tech’s Biocomplexity Institute, which houses the lab.
Lab Director Madhav Marathe said his team provides simulations as a service, data analysis as a service and decision support as a service.
One tool the platform uses is called Synthetic Information Based Epidemiological Laboratory, which the lab built for the Defense Threat Reduction Agency. A web-based application, SIBEL also has been used by the Defense Advanced Research Projects Agency, Intelligence Advanced Research Projects Activity and National Institutes of Health. It’s been part of more than 30 Defense Department case studies, including planning exercises and Ebola response. It’s free to use, but first-timers must submit a request for an account to access it.
SIBEL’s backend is the synthetic information system, which has detailed information on cities and regions worldwide.
The second component is forward simulations, which take an understanding of a disease in a mechanistic form and use networks to simulate how it would spread. It also incorporates changes in actions resulting from the introduction of interventions such as vaccinations, isolation and school closures.
The third component is the software system that makes the platform and tools accessible to users with little to no tech-savvy.
“We can take a high-school kid now and, in about a day, train the high school kid to start using the system,” Marathe said. And that’s the point, he added. Once the lab created the platform and tools, researchers assumed they would be widely used. They weren’t, though, because the platform was too sophisticated. “We want to provide the same kind of service that Google provides when they do a search.”
That’s why SIBEL lets users choose an area and disease of interest, set parameters by pointing and clicking, add in potential interventions and pull in any known data about the disease. When a user presses “start,” the backend initiates the simulation and executes a series of jobs on high-performance computing clusters where the synthetic population data resides. When the process is done, users can see actionable information, such as which interventions are likely to be the most successful and what part of the region could be hit hardest.
“You can go to the map and see how the hotspots might look like,” Marathe said. "All of this is automatically produced at the end of one simple 15-minute setup followed by the runs on a supercomputer that we have housed here.”
Other tools include EpiCaster, which provides near-real-time forecasts of epidemics, and My4Sight, which uses crowdsourced data and human computation to enhance disease forecasting. It’s a deep technology stack that includes basic networks, high-performance computing, artificial intelligence and machine learning with web apps at the top, Marathe said.
He sums it up as p-cubed analytics: precise, personalized and pervasive. “It’s pervasive in that we want this set of tools to be used by anyone at any place with any device,” Marathe said. Whether it's a mother trying to take care of her children or the mayor evaluating policy options, everyone should be able to use this tool and get personalized information based on their location or role, he said. The mayor should get data that is specific and actionable enough to make a policy change. During an epidemic, parents could use it data to choose the best evacuation route.
The ability to account for heterogeneity is the platform’s differentiator from past catastrophic-event analyses tools, Barrett said.
“It changes the whole point of what the research is,” he said. “It isn’t about delivering a study to people who then make a decision and then 20 years from now you do another study. You have continuous access to this technology to make decisions … and it makes this science plug into modern life in a way that research has only dreamed about.”