Bringing machine learning to image analysis
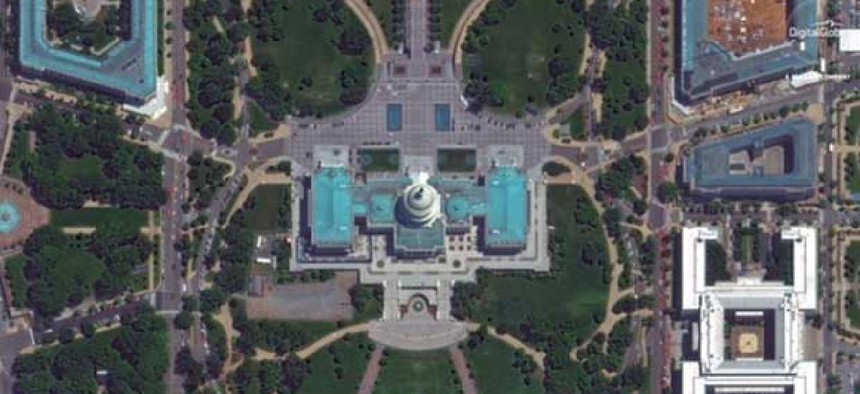
Connecting state and local government leaders
AWS technology is helping DigitalGlobe streamline the processes for building, training and deploying models that can improve the analysis of satellite images.
Satellite image ©2018 DigitalGlobe, a Maxar company.
Satellite data is being generated at a volume to large for humans to process, according to Nate Ricklin, director of platform engineering at DigitalGlobe, a satellite imaging company with a number of government customers.
“What this means is we need to go way, way faster in pulling information out of these pixels,” he told attendees at the June 21 at the AWS Public Sector Summit. The solution: artificial intelligence.
In 2017 DigitalGlobe moved its 100-petabyte imagery library to an Amazon Web Service's cloud where it uses Amazon SageMaker to streamline the processes for building, training and deploying machine learning models.
The first step is collecting training data. “That’s the hardest part,” Ricklin said. “The training data has to be super high quality, and you need a lot of it.”
The training process requires a human to examine images and draw boxes around the objects the model should recognize. After reviewing hundreds of marked up images, the model learns what it should be looking for. A model can be made more robust by adding synthetic training data, like 3D images inserted into satellite images, to help improve the model, Ricklin said.
Ricklin demonstrated one model that was able to recognize man-made structures, in real time, as he scrolled around a satellite image. Another did the same for airplanes parked at an airport.
The technology can also compare two images -- one old and one more recent -- of the same location to show changes over time, he said. The requires two models, one running on each image, to look for whatever object the model is trained to recognize, like a specific building. It then automatically, in real time, highlight all of the structures that are “new” or that appear in the most recent image, but not the older image.
“But it's not perfect,” he said. In this example, the system thought there was no building in the older image, but there really was. These kinds of abilities will improve the more they’re used, he added. "That’s why we need you, we need everyone in the community to come … and help advance the state of AI in satellite imagery.”
Editor's note: This arictle was changed June 26 to replace the image and add a credit.