Can electricity use predict a bad morning commute?
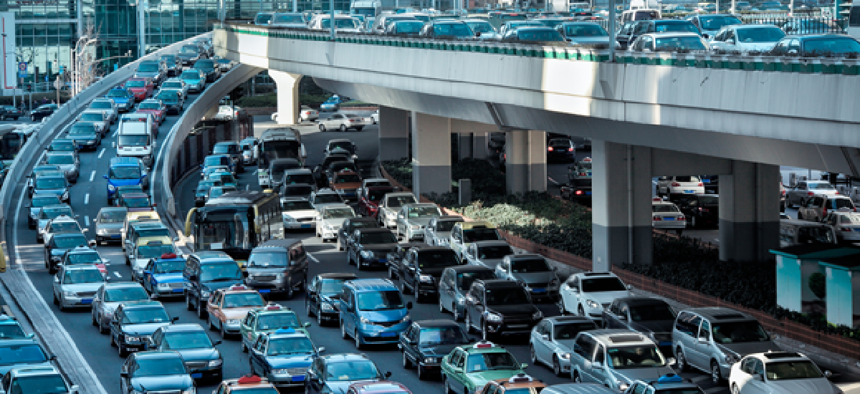
Data on household electricity consumption could help transit agencies predict morning traffic congestion, new research suggests.
Why are some morning commutes so much worse than others?
New research shows that nighttime and early-morning energy use can be a good predictor of morning traffic congestion.
Sean Qian, an assistant professor of civil and environmental engineering at Carnegie Mellon University, and Ph.D. student Pinchao Zhang created a model that mined data on electricity consumption from 322 homes in Austin, Texas, and used artificial intelligence to predict what traffic would look like the next morning.
The anonymous electricity data was collected by Pecan Street Inc., which had enrolled hundreds of homes in the Austin area in an advanced metering infrastructure, or smart meter, program that tracks energy consumption. Travel time data came from the National Performance Management Research Data Set.
The model sorted users into groups by the amount of electricity they used at midnight and early in the morning. The model learned what features of the user categories related to morning traffic congestion and used AI to make predictions. It even showed that day-to-day changes in households' energy consumption patterns was reflected in the time congestion started.
“If we know that out of the 322 households that 300 households are going to sleep early, then there is a positive correlation to an earlier congestion time the next day,” Qian told GCN.
The study showed, he explained, that urban systems are interdependent and one where known variables can be used to predict other unknown conditions.
This kind of information could be useful to local transportation departments. Knowing when congestion is going to start could give them the opportunity to make an announcement and try to get ahead of problems, Qian said.
Electricity use out-performed real-time traffic data as a predictor of roadway congestion in this study because the threshold -- the number of cars on the road -- at which a roadway goes from smooth sailing to congestion is largely random, Qian said. There is no clear pattern in the transition from free flow traffic to congestion, he wrote in the research paper.
Electricity use, however, proved to be a decent predictor, and trend data showed that congestion time correlated with electricity use.
But this is early research, and it’s not perfect.
“The maximum prediction error on a day can be as many as 50 min.,” the paper concluded. “In other words, aggregated features imply useful information regarding the commuters’ activities, but the prediction may be further improved.”
Accuracy could be boosted by having more homes contribute data to the model and by adding more systems, like water and sewer, Qian said.
“We’re showing that this is a plausible research direction for the future,” he said.
NEXT STORY: Helping smart cities tap into federal funding