Data wrangling: How data goes from raw to refined
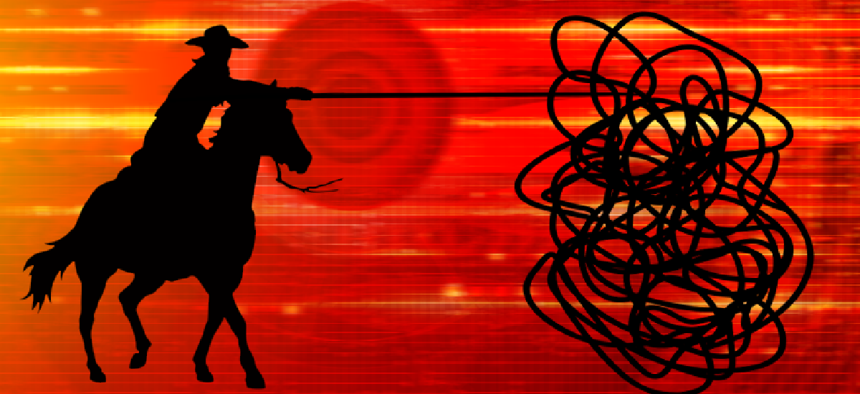
Specialized software combines, formats and manages massive amounts of complex data for accurate and meaningful analysis.
A new approach is helping researchers speed the time it takes to prepare data for analysis -- a task that takes as much as 95 percent of workers’ time on an analytics project, according to some estimates.
Known as data wrangling, the methodology makes it easier for researchers to get data into a format that a software program can use for analyses, such as reports or visualizations such as graphs and 3D models. The process involves finding the best data – that's both accessible and usable -- and then "shaping and combining that data to facilitate the most accurate and meaningful analysis possible," according to a July report on the topic from the National Science and Technology Council.
Data wrangling is necessary because “the dramatic rise in our ability to collect data isn’t yet matched by our ability to support, analyze and manage it,” according to Mark Conrad, archives specialist at the National Archives and Records Administration’s Systems Engineering Division of the Office of Information Services. “We generate more data than we can possibly read or comprehend, and need a way to summarize and analyze the ‘right’ data in order to use this information effectively and efficiently,” he told GCN via email.
There’s no one way to wrangle data, Conrad said, because it depends on wide range of variables: the amount of data; the questions to be answered; the data available to address those questions; the amount of work needed to combine datasets; the software available; whether specialized hardware such as cloud or supercomputing is necessary to handle the volume or complexity of the data; and the audience for the analysis; among others.
“NARA uses data wrangling to support data-driven decision-making for everything from optimizing space utilization across our facilities, to improving our online customer service, to optimizing workflows in order to improve work unit performance,” Conrad said.
For instance, using testbed collections designed to simulate NARA’s electronic records holdings, the agency’s research partners at the Texas Advanced Computing Center extracted metadata from a collection of federal files. They used that metadata to make visualizations based on the agency of origin, file type and preservation concerns.
Similarly, researchers at the University of North Carolina-Chapel Hill -- who are now at the University of Maryland -- used software to detect files with geographic information, determined the geographic coverage of those files and presented findings on an interactive map.
“Both would have been difficult -- if not impossible -- to do without data wrangling,” Conrad wrote.
Researchers at Stanford University and the University of California-Berkeley first used the term “data wrangling” when they created a machine learning-powered tool that would ease the problem of getting data into a required format. Within six months, it had been downloaded 30,000 times, and six years ago the researchers who created it, launched a company called Trifacta to commercialize the technology.
“Now they can do in clicks what used to take months,” Trifacta CEO Adam Wilson said. The tool uses algorithms to learn from the data and understand how users interact with it to provide intelligent recommendations.
One concern is that every user could wrangle data differently. For instance, if an agency has 126 analytics tools, the data shouldn’t be wrangled 126 times and ways, Wilson said. To avoid that, agencies should not embed data wrangling in end-user tools.
“You really want to have a platform-based approach to wrangling the data that ensures you can wrangle the data once and you can use it everywhere,” he said.
The Centers for Disease Control and Prevention used Trifacta’s Wrangler to understand an outbreak of HIV in rural Indiana. Data professionals were trying to make sense of medical records, police files and psychographic data -- much of it unstructured -- to find patterns.
Wrangler applies AI and machine learning to take the data from raw to refined and to infer what users are interested in as they manipulate it. The tool then gives immediate previews of what the data would look like if specific transformations or rules to clean the data are applied.
“What they found after going through all this was that there was a lot of urbanization that was happening in parts of Chicago that was causing migration of some individuals from the big city to these smaller towns,” Wilson said. "That was tied into opioid issues and issues around prostitution and needles, and these communities were ill-equipped to deal with some of these urban problems.”
In truth, data wrangling isn’t new, NARA’s Conrad added. The difference today is the sheer scale of the challenge.
“We have always had data wrangling of one sort or another,” he said. “In the past, data was periodically gathered manually, tabulated, and the aggregated data was summarized in a standardized report. Today, data is generated on a near-constant basis from multiple sources both internal (e.g., data from various applications used by staff) and external to NARA (e.g., web usage statistics, customer comments and survey responses). This data can be manipulated and combined (wrangled) and analyzed and visualized so decision-makers can understand the big picture of relevant activities in near ‘real time.’”
NEXT STORY: How can AI help government improve?