Combatting facial recognition bias with better data
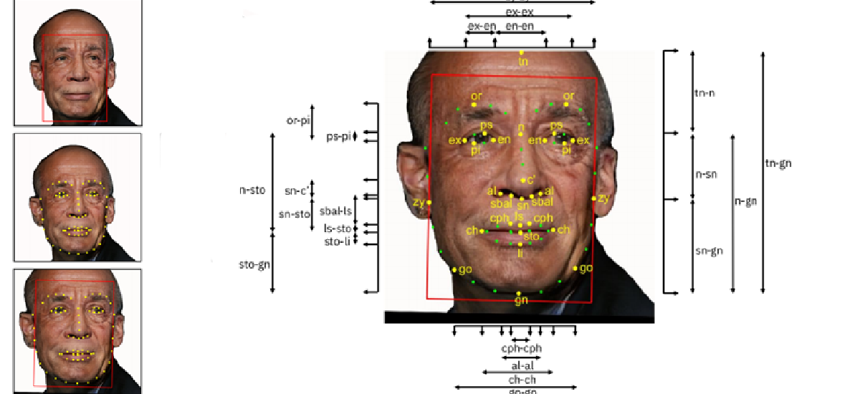
Connecting state and local government leaders
IBM's Diversity in Faces dataset is an annotated collection of 1 million facial images drawn from pictures and videos compiled from Creative Commons data available on Flickr.
A major criticism of facial recognition technology is that it can only deliver results based on the data used to train it. And when it learns from datasets where young white men are overrepresented, it has difficulty correctly identifying women and people with darker skin tones, leading to false positives and charges of bias in the artificial intelligence algorithms.
To increase the variety of the training data for facial recognition algorithms, IBM has released the Diversity in Faces dataset, an annotated collection of 1 million human facial images.
The DiF dataset is drawn from the publicly available YFCC-100M Creative Commons dataset, 100 million images and videos compiled from data available on Flickr. IBM researchers tagged selected photos with 47 feature dimensions and used 10 coding schemes to obtain objective measurements of features of human faces.
To select the photos from Flickr, IBM researchers first filtered the YFC-100M images for pictures with Creative Common licenses, according to a research paper describing the process. They then used a convolutional neural network to identify faces and a machine-learning toolkit to select photos of a usable size where the subject was facing the camera.
The resulting 1 million face images were tagged with 19 landmarks that are used in the coding schemes to describe facial features in terms of their distance from each other, size and symmetry as well as skin color, age and gender predictions and contrast, which tends to vary with age. The researchers also used crowdsourcing to obtain human-labeled gender and age annotations.
While the IBM researchers acknowledged the 47 total feature dimensions may not evenly cover the diversity among human faces, "we believe the approach outlined in this work provides a needed methodology for advancing the study of diversity for face recognition," they said.
Although facial recognition systems are just starting to be used by government agencies, their reliability has been questioned. In July 2018, the ACLU released the results of a study that found Amazon’s version of the technology, Rekognition, misidentified 28 members of Congress.
These examples, and other have prompted calls from academics and tech companies for federal leadership over privacy protections and how the technology will be deployed.
The technology has enjoyed some successes.
Many states are using facial recognition technology to prevent the issuance of fraudulent drivers licenses that contribute to identity theft. The software compares facial characteristics on a driver’s license or ID photos with other images on file with the Department of Motor Vehicles. Officials in New York, which has been using the program since 2010, in August 2017 said the technology has resulted in spotting some 21,000 cases of possible identity fraud, with the number of positive matches increasing as the technology improves.
In October 2018, Customs and Border Protection’s tech trial of biometric entry/exit identification nabbed two imposters attempting to cross from Mexico into the U.S. near Yuma, Ariz., using someone else’s border-crossing cards. Dulles International Airport, an early adopter of the system, identified its first imposter in August.
The IBM dataset is available to the global research community upon request.
NEXT STORY: The 5G skills gap: What does government need?