NYPD's machine-learning software spots crime patterns
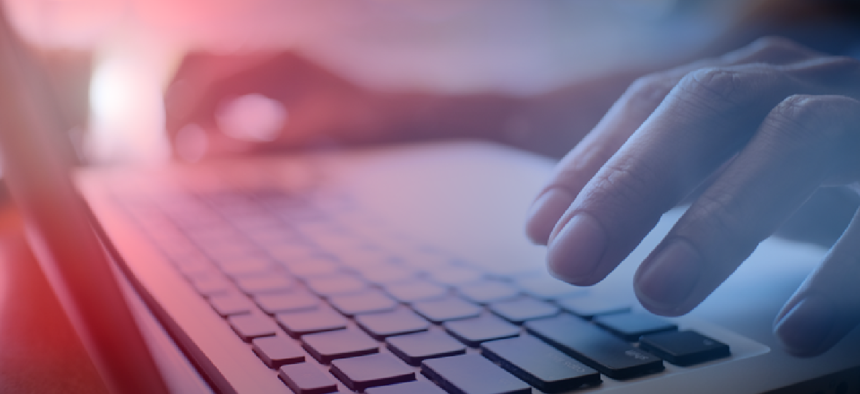
Patternizr's pattern-recognizing algorithms are helping the New York City Police Department find relationships among crime types with greater efficiency and less bias.
Pattern-recognizing algorithms are helping the New York City Police Department sort through crime data to find relationships among three crime types with greater efficiency and less bias.
The department has been using the software, known as Patternizr, since December 2016, but discussed it last month in a report in the INFORMS Journal on Applied Analytics. NYPD is the first law enforcement agency to use this type of tool, according to the report.
Based on machine learning, Patternizr was trained using manually identified patterns for burglaries, robberies and grand larcenies in the city to find relationships among them. The final models were incorporated into NYPD’s Domain Awareness System -- a citywide network of sensors, databases, devices, software and infrastructure. All historical pairs of complaints were then processed in the cloud against 10 years of records of burglaries and robberies, and three years of grand larcenies data. To keep the software up-to-date, similarity scores were calculated and updated for new and revised complaints three times a day, and each was scored against the existing crime data before being incorporated into DAS.
Patternizr has separate models for the three crime types, each of which has many manually identified patterns -- about 10,000 apiece between 2006 and 2015. Each type also has about 30,000 complaint records in which the same person was arrested multiple times for the same offense within two days. Those complaints include unstructured text about the crime and structured data such as date, time, location and suspect information. That data lays the foundation for calculating the five types of crime similarities that Patternizr spots: location, date and time, categorical, suspect and unstructured text.
The Patternizr app is part of DAS on NYPD desktop computers. To use it, analysts select a “seed” complaint, press “Patternize” and the software compares the complaint against the hundreds of thousands of records in the department’s database. It then assigns a similarity score to each comparison to show the likelihood that a pair of crimes are in a pattern. It returns a list of those scores in descending order for the analyst to review.
“The models that comprise Patternizr are supervised machine-learning classifiers; that is, they are statistical models that learn from historical examples where classifications are known and are then used to predict the classification for samples for which the classifications are unknown,” the report stated. “In the case of Patternizr, each example is a pair of crimes, and the classification is whether the two crimes are in a pattern together.”
In addition to the scores, investigators see the distance, time apart and algorithm calculated for each result, in addition to a map showing the seed and similar results. They can then choose what to examine more closely.
“The investigator can actively filter and query the result list,” according to the report. “A general text search, and filters for distance, time apart, presence of arrest, and premise type, are all available and may be used simultaneously.”
The algorithm avoids bias by excluding sensitive information, such as race, about potential suspects, and by “coarsening” location data and other potential proxy variables for sensitive information to keep it from being too specific. Additionally, human reviews are still necessary to establish a pattern. Tests of the software found “no evidence that Patternizr recommends any suspect race at a higher rate than exists with random pairing,” according to the report.
Between January and July 2018, officers ran about 400 complaints per week through Patternizr -- or about 30% of all the burglaries, robberies and grand larcenies recorded by the NYPD during that period, the report states.
Those three crime types were selected because they have a high number of serial offenders, and once a pattern has been found, police can use evidence to more easily find the perpetrator, according to the report.
Historically, police officers relied on their memories to find crime patterns, remembering incidents with similar characteristics and investigating further. Today, search engines help, but they are often limited to precincts -- of which NYPD has 77 -- whereas Patternizr spans them.
An analyst at one precinct was investigating a crime in which a shoplifter attacked an employee with a hypodermic needle. The officer ran the complaint through Patternizr, which found another robbery in another precinct that involved a similar threat with a needle.
“The investigators combined these two complaints into an official pattern, along with two other larcenies committed by the same perpetrator, and the pattern was then passed to the detective squad,” the report states. “NYPD conducted an investigation and arrested the perpetrator, who later pled guilty to larceny and felony assault.”
Patternizr took NYPD two years to develop – an effort that included work on the algorithm, backend systems and user interfaces. A second version may include greater ability to compare across crime types and to filter for the use of force.
“Other police departments could take the information we’ve laid out and build their own tailored version of Patternizr,” Assistant Commissioner Evan Levine wrote in an email to GCN.