Beyond Flint: How RPA is changing the inspection process
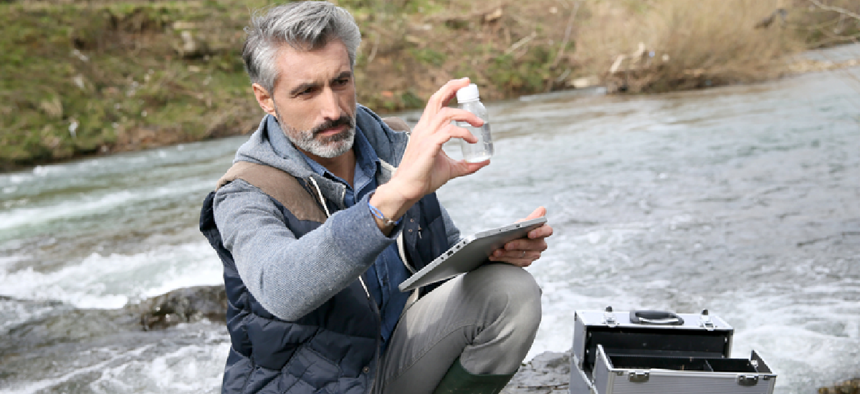
Present in almost every agency, inspection functions are the perfect jumping-off point for artificial intelligence, machine learning and robotic process automation technologies.
We often take for granted government's responsibility to ensure safety by inspecting everything from the water we drink and the legitimacy of the organic stamp on our eggs to the safety of our cars -- not to mention the roads on which they travel and the security of the buildings to which we drive. Most of the time, we see how important this work is only when violations like the water crisis in Flint, Mich., hit the national stage. The harsh reality is that 25% of America’s water systems fail to meet safety standards set by the Environmental Protection Agency, showing the importance of inspections. Unfortunately, many oversight agencies are hampered by budget cuts and understaffed teams working with outdated processes.
Most inspections are performed the same way they were decades ago -- data is entered by hand on paper-based forms that require inspectors to spend significant time in the office rekeying their findings. Augmented robotic process automation (RPA) changes the way government agencies works. It eliminates manual, inefficient and error-prone data entry by using inspectors' own data to automate hundreds of manual tasks, creating a more-efficient inspection process and making inspectors 10 times more productive. That means more inspections can be completed and violations detected, keeping citizens safe.
Data-driven enforcement to predict public risk
The idea of data-driven enforcement is not new. For example, transportation and law enforcement agencies have been seeing great results by combining traffic and crime data to pinpoint where police presence could have the greatest impact on improving the community's quality of life.
For inspections, a data-driven process means that organizations can prioritize where they send inspectors based on the potential risk to the community or history of violations. It means inspectors have access to full case files in the field via mobile devices to help put real-time issues into context. Inspectors’ daily routes and workflow are planned using artificial intelligence and machine learning based on real-time traffic and activity data, enabling them to optimize their time in the field, visiting more sites per day.
The impact on agency missions
Key objectives of government agencies can be realized with a data-driven process that delivers data transparency and security. Every employee, manager and executive has the same access to all of the data related to an inspection via one electronic platform. Changes or inconsistencies become evident quickly as dashboards and data reports are instantly made available to all stakeholders.
A centralized view of all data also helps agencies fully realize the value of internet-of-things investments. Those that have deployed sensors, drones and cameras are frequently not taking full advantage of the data these devices capture. Though IoT devices can alert agencies to anomalies in real time, that data may not be used after the issue is addressed. In a data-driven inspection environment, that data is captured and aggregated to show trends for proactive efforts to improve safety.
The impact of data-driven inspections goes beyond the people directly involved in regulating. Through better and more visible access to data, it becomes easier to find and stop organizations or people who are not following guidelines or trying to beat the system for personal gain. This means catching violations before they harm communities, not after. When agencies are more efficient at finding violations, they not only mitigate risks and bring in more revenue through fines, but they also can channel resources into other areas of their mission.
Beyond theory: Data-driven inspections in the field
At one state environmental agency responsible for inspecting and regulating sites including gas, solid and hazardous waste, inspectors took notes by hand, including hand drawing maps, and then returned to the office to key in all of their data. This manual process not only took time, but it also opened up the data to data-entry errors. To improve the process and bridge the gap between workforce and workflow, the agency initiated a Smart Inspection initiative. All data is now collected on tablets and sent directly into agency systems. Inspectors can pull up maps, take pictures and make annotation directly on images before uploading them. In the office, managers use predictive algorithms to prioritize inspectors' schedules by identifying regulated sites with the highest risk for regulatory non-compliance.
In another instance, inspectors working on hurricane recovery used tablets loaded with a specific workflow, checklists for debris removal and federal recovery funding requirements. All information was stored in that property’s file and sent immediately to the Federal Emergency Management Agency for funding decisions. Those overseeing the cleanup had a centralized portal to view status and progress. With all of this data in one place, they prioritized high-risk inspections and more effectively mapped routes for the most effective use of inspectors' time. Because all of the information was captured in a prescribed fashion, funding decisions can be based solely on data collected. There is less room for human bias or interpretation to color funding decisions.
Inspection functions, which are present in almost every agency, are the perfect jumping-off point for AI, machine learning and RPA technologies. All agencies are looking for ways to do more with less and meet modernization goals to become more data-driven. Adopting these technologies in inspections and enforcement is not only a quick win for modernization goals, but also key to stopping preventable issues before they impact our communities.
NEXT STORY: Why U.S. Tech Inventors Are So Highly Clustered