Fighting bias in AI-powered decisions
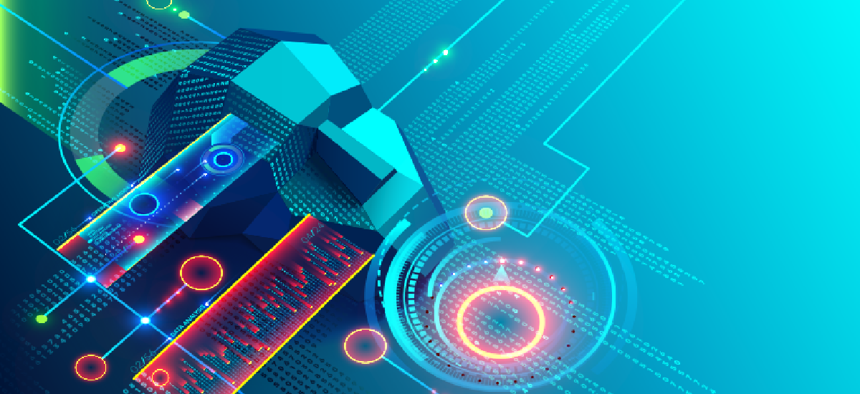
Connecting state and local government leaders
Artificial intelligence can streamline or eliminate manual labor, but organizations still need managers to monitor and make corrections when results discrimination surfaces.
Artificial intelligence and automation can streamline or eliminate manual labor, but public- and private-sector organizations still need managers to monitor and make corrections when algorithms exhibit biases.
At a Nov. 20 panel on “Artificial Intelligence at Work” hosted by Workday and Politico, Rep. Bill Foster (D-Ill.) pointed to the history of loan discrimination against minority groups to highlight the need for to the ability to overwrite neural networks to prevent unintended bias. “It is statistically true that people of different racial groups are more or less likely to have relatives that are wealthy because of unjustifiable past discrimination,” he said at the panel. “The problem is you then can have two identically situated families [represent] two different racial groups, so the neural network will identify proxies for race, and you’re left with a choice. Are we going to tell the neural network “No” that even though this is a statistically valid way to maximize your profits?”
Part of the problem is that bias is hard to assess, according to National Science Foundation Assistant Director Dawn Tilbury, who heads NSF's Engineering Directorate. She pointed to her agency's Future of Work at the Human-Technology Frontier program as one effort to monitor how humans and technology interact, particularly when the outcome of algorithms can easily be mapped, but their intention is harder to parse.
“How would we define that a data set is unbiased or fair? I don't think we have that definition,” she said. “[The NSF] wants to make sure that these algorithms are fair, whether they’re used for hiring or whatever. A lot of what the government can do is fund basic research to help advance these understandings -- because the private industry is just going to do what's most profitable.”
NSF scrutinizes its own projects as much as possible, Tilbury said. “For example, we look at how many of our project proposals were submitted by women, then how many women-led projects were actually funded,” she said. “If only a certain amount of women’s projects were funded or submitted we ask, ‘What happened there?’”
She pointed to studies demonstrating that projects from people with foreign-sounding names, or whose names sounded more feminine, often did not get accepted for funding, something the NSF looked to push back against. “If you feed that bias into your algorithms, you’ll get the same biased results unless you retrain the system.”
Foster appeared to agree with Tilbury’s assessment on the panel, stating that while there wasn’t a specific law on the books to combat discrimination in technology, efforts were being made within government to address the interactions of discrimination and AI.
“We have a couple of papers coming out that address this point and the problem with AI solutions and hiring when you look at the non-digital world, [such as] the kind of the criteria to evaluate bias and discrimination concerns based on outcome measures,” he said. “Did the employer exhibit evidence of intent to discriminate? How do you measure the intent of an algorithm?”
This article was first posted to FCW, a sibling site to GCN.
NEXT STORY: How government uses AI