IBM calls for AI standards, regulation
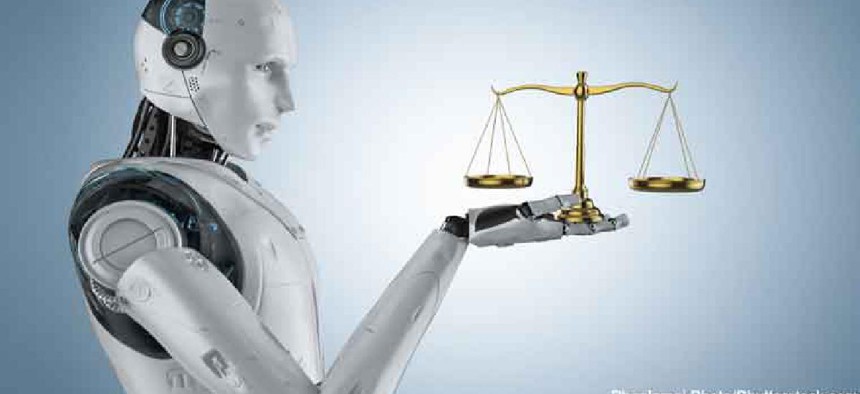
IBM is urging the public and private sector to work together to combat discriminatory practices fostered by unregulated artificial intelligence.
Outlining standards to eliminate bias in artificial intelligence, IBM is urging the public and private sector to work together to combat discriminatory practices that could harm women, minorities, the disabled and others.
"It seems pretty clear to us that government regulation of artificial intelligence is the next frontier in tech policy regulation," said Chris Padilla, VP of government and regulatory affairs at IBM, a day ahead of a panel discussion on AI at the World Economic Forum in Davos, Switzerland. The panel included IBM CEO Ginni Rometty and Chris Liddell, White House deputy chief of staff for Policy Coordination.
IBM's risk-based AI governance policy is based on accountability, transparency, fairness and security. The computing giant wants companies and governments to develop standards that will, for example, address bias in algorithms that rely on historical data (such as ZIP codes or mortgage rates) and ensure that African-Americans have fair access to housing. Such standards would be likely be developed by National Institute of Standards and Technology, which would convene stakeholders to identify and promote definitions, benchmarks, frameworks and standards for AI systems, the company said in a policy blog.
Governments also should support the financing and creation of AI testbeds and incentivize participants to voluntarily embrace standards, certification and validation.
One step companies could take, IBM suggested, is to appoint AI ethics officers charged with determining how much potential harm an AI system might pose and maintain documentation about data when "making determinations or recommendations with potentially significant implications for individuals," so that the decisions can be explained.
"Bias is one of those things we know is there and influencing outcomes," Cheryl Martin, chief data scientist at Alegion, an Austin-based provider of human intelligence solutions for AI and machine learning initiatives, told GCN's sibling site Pure AI in an earlier interview. "But the concept of 'bias' isn't always clear. That word means different things to different people. It's important to define it in this context if we're going to mitigate the problem."
Martin laid out the four types of bias during that interview: sample/selection bias (the distribution of the training data fails to reflect the actual environment in which the machine learning model will be running), prejudices and stereotypes (which emerge in the differentiation process), systematic value distortion (when a device returns measurements or observations that are imprecise), and model insensitivity (the result of the way an algorithm is used for training on any set of data, even an unbiased set).
This article was first posted to PureAI, a sibling site to GCN.
NEXT STORY: Automation pays off