GeoAI: A prescription for COVID-19 prediction
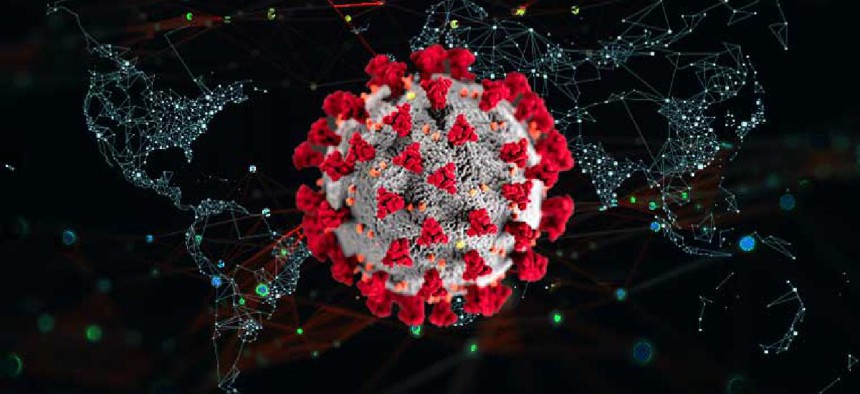
Connecting state and local government leaders
Algorithm-powered location intelligence can show geographic patterns and trends and help public health officials make predictions about fast-spreading viruses.
Online dashboards that track the number of COVID-19 cases are popping up worldwide as health officials scramble to contain the virus, but one aspect that may be underused is the ability to use artificial intelligence to predict what regions might experience an outbreak next.
Location intelligence software and GIS technology is powering many of the dashboards that organizations worldwide, including the World Health Organization, are implementing to monitor the flu-like illness.
Johns Hopkins University’s (JHU) Center for Systems Science and Engineering was the first to stand up and regularly update an online dashboard showing statistics about the number of confirmed cases, deaths and recoveries. Launched Jan. 22, the dashboard displays a map that shows at a glance places with high infection rates as well as areas that remain untouched as yet. Since then, dashboards in Chinese, Spanish, Lithuanian and other languages have emerged.
But there’s more that could be done, said Este Geraghty, Esri’s chief medical officer.
“When you do statistical analysis that considers geography, it’s a little bit different from traditional kinds of analysis.… Things that are closer together are more likely to happen in a similar way vs. things that are further apart,” Geraghty said. “You can take that idea -- we call it spatial autocorrelation -- and use that to make predictions and to see patterns and trends geographically over space and time.” That data opens the possibility for predictive analytics, machine learning and artificial intelligence applications.
Esri makes available many datasets for free in its Living Atlas repository of geographic data, including data contributed by users such as the Centers for Disease Control and Prevention, which shares its Social Vulnerability Index, and the Robert Wood Johnson Foundation, which has county community health rankings. Now, JHU is sharing the data it’s collecting from sources such as the Chinese government and social media data feeds. Plus, the company contributes its own curated data -- in particular, demographic data that can help health officials determine areas’ populations and identify groups that may be more susceptible to a virus.
There are “many, many tools that you can use to do analytics,” Geraghty said. “You might start with something like a hot spot analysis to see where things are flaring up.”
The maps on JHU’s dashboard don’t incorporate spatial analytics, but if they did, officials might be able to detect other patterns that could show the direction of coronavirus spread and then correlate that with other datasets such as airline travel, public transportation or cities with higher density, where person-to-person transmission may be more likely. All of those factors could be put into a predictive model along with data on humidity and temperature “because viruses like colder temperatures, which is why you don’t see it as much in southern latitudes,” Geraghty said.
An AI platform was among the first to issue a warning shot about the coronavirus. Canadian firm BlueDot, which uses AI-powered algorithms to identify disease outbreaks and forecast their spread, warned customers on Dec. 31, 2019, to avoid Wuhan, China, where the coronavirus originated. That was about 10 days before WHO issued its notice.
An article last year in the International Journal of Health Geographics touts GeoAI -- the combination of AI and GIS -- and health intelligence, which it defines as “the specific application of AI and data science methods and tools to provide accurate, efficient, and productive insights into healthcare and medicine.”
The article cites several applications, such the use of deep learning neural networks to track real-time flu forecasting at regional and city spatial scales in the United States based on spatial big data from Google Flu Trends and climate information from the National Climatic Data Center. Another study developed a machine learning model to detect foodborne illnesses using anonymous and aggregated Google search-and-location data to estimate the number of people who visited a particular restaurant and subsequently searched for terms related to food poisoning, with the idea of better targeting restaurant food inspections.
“The value of geography in monitoring and managing health crises of many kinds goes well beyond a map,” Geraghty said. “Location intelligence helps explain “not only what’s going on, but to take actions in more meaningful and directed, evidence-based ways,” she said.