The power of analytics and visualization for city governments
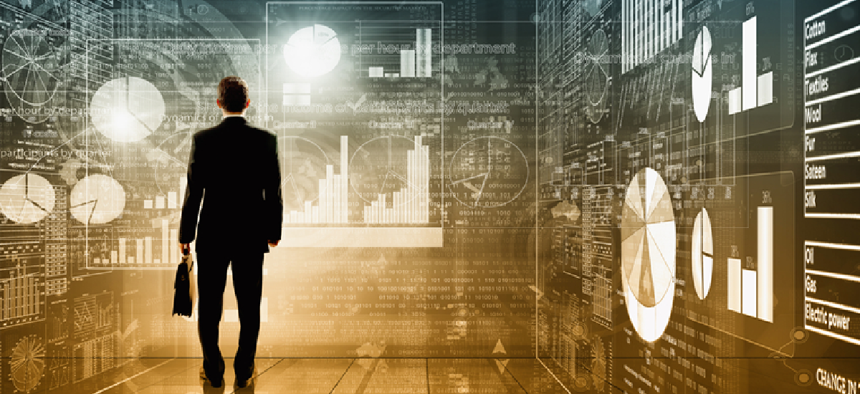
Connecting state and local government leaders
Cities are using insights gathered from the internet of things, embedded analytics, machine learning and experience management to create new programs, become more efficient and deliver consumer-grade services.
Cities have always been active collectors of data. For decades they gathered reams of information and stashed it in file cabinets and on hard disks, where most of it sat until someone made a deliberate effort to look it up.
Today, government decision-makers can’t afford to let data sit. They need to collect it, keep it safe and share it across departments to do the work they’ve been entrusted to accomplish: ensure data privacy across organizational silos, protect the community, provide services and help the economy prosper. To succeed, governments are embracing technologies that analyze and visualize data coming from places as disparate as sewers and tax forms.
Public organizations are using insights gathered from smart technologies such as the internet of things, embedded analytics, machine learning and experience management to create new programs, become more efficient and deliver consumer grade services.
Data from IoT sensors is unlocking new opportunities. Analysis and connectivity, coupled with machine learning, can support managing and evaluating infrastructure, assets, traffic and the environment. Remote condition monitoring provides real-time data from public infrastructure to help predict maintenance needs. Additionally, sensor data can help the government track water containment to reduce flooding, monitor soil for landslide risk and track endangered wildlife.
Embedded analytics can deliver real-time visibility into changing environments, as the following three cities illustrate.
Floods, terrorism and taxes
Buenos Aires used IoT to solve a chronic flooding problem. After a particularly disastrous flood killed nearly 100 and forced thousands of evacuations, the city put sensors on 30,000 drains to measure the water’s direction, speed and levels, and it set up automated maintenance on 1,500 kilometers of drainage pipes. Data was transferred immediately to a cloud platform from which city workers monitored water levels around drains and dispatched crews to clear away debris before problems developed. Analyzing the sensor data enabled Buenos Aires predict flooding and alert residents of high water. The year after sensors were installed, the city didn’t flood.
In the resort town of Antibes, France, government leaders set up a similar array of 2,000 sensors along 200 miles of water pipes and at the bottom of a reservoir. They had a different goal in mind: protecting the city’s residents and tourists from terrorist attacks on the water supply. Local officials set up a two-pronged defense that analyzed data in real time from water sensors and deployed end-to-end encryption, allowing the city to detect an intrusion on the water supply and trigger an alarm.
In Queensland, Australia, the Office of State Revenue tapped machine learning to predict which taxpayers were likely to incur debt. In a proof-of-concept, the department crunched 187 million tax records from 97,000 tax-payers to spot patterns in payment activity. The system predicted, with 71% accuracy, when a taxpayer would default. Officials were also able to classify non-tax-payers into two groups and devised mitigating strategies for each. The group designated as having no intention of paying received proactive notifications and strongly worded letters. Those who wanted to pay but were unable were contacted with more-flexible payment terms.
Conclusion
Government agencies are pulling in more data than ever before. But unlike in past decades, they’re doing more than storing it. They’re deploying new technologies to evaluate and leverage information in real time to become more anticipatory, as well. City governments that use analytics and visualization most skillfully will stand the best chance of meeting citizens’ rising demands.
NEXT STORY: Solving the time-to-value data challenge