AI may be able to predict urban landslides
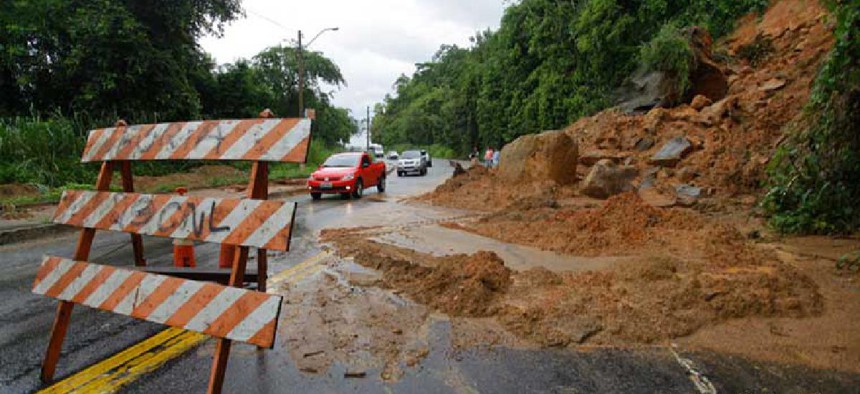
Connecting state and local government leaders
A Pittsburgh-based scientist is looking at ways to use machine learning and edge computing to analyze images of local streets for indications of landslide risk.
Technology that can help predict which roadways are at risk of landslides is under development at Carnegie Mellon University’s Metro21: Smart Cities Initiative.
Christoph Mertz, principal project scientist at CMU’s Robotics Institute, came up with the idea to study images of roads and their surroundings to determine the best indicators of landslide risk, after hearing the keynote speaker at the 2018 launch of Metro21, a research and development arm of CMU, talk about dealing with the aftermath of a record number of landslides in Allegheny County, Pa., that year.
“I have been collecting a lot of pictures from vehicles in the past, and I was thinking to myself, ‘I wonder if you can find indicators of landslides in those pictures,’” said Mertz, whose company, Roadbotics, uses imagery and artificial intelligence to inspect road surfaces for transit agencies. “There’s many things one could see in those images. There might be excess dirt, there might be also excess water, which means there’s a drainage issue. Here in southwestern Pennsylvania, if the red clay is saturated with water, then it starts to move.”
Other potential indicators could be cracks in the road and tree movement, he said. “I’m wondering if they’re just like a dial,” Mertz said of trees. “If there’s a small movement on the ground, there might be a large movement on top.” At this point, studying the positions of the trees is still just an idea because Mertz would need pictures of the same trees over time to see if they move.
To collect data now, he mounts a smartphone on the front windshield of a vehicle and takes video of the road ahead. This is a separate effort from Roadbotics’ primary work using images, AI and machine learning to create interactive maps of road conditions so local governments can strategically treat existing problems.
In the future, Mertz said he plans to work with Pittsburgh’s transit bus system. Because buses travel the same route every day, he would get data on the same area over a long period of time, enabling him to see changes emerge.
The plan is to buy the types of external cameras many buses already use for security and incident monitoring and put software -- neural networks -- on the devices. Mertz would train the networks offline to detect cracks, count parked and moving cars and identify hazards and changes. Using edge computing, the devices would analyze the videos, then geotag and flag the data Mertz seeks. The computer would save the data for 30 days, and snippets of it would go to a central system that might be in the cloud or a server at CMU.
“You have all these cameras on the bus so there’s lots of video streams,” he said. “You cannot upload those to the cloud because it’s just too much data, so we have to do an analysis on the bus itself and then only send out small snippets of data which are important.”
When a change is noted, Mertz said, one approach would be to take all the images from the area, analyze them offline in the lab and make a 3D model. He’s already made two models of a landslide location after photographing it four times in one year to see how it changed. The next step is to share the model with the university’s civil engineering department for additional study.
Mertz doesn’t have a precise timeline for going from ideation to practice, but he has identified a bus company to work with and hopes to have data within a year to start analyses.
“I hope that we will soon have one indicator that can throw up a red flag,” he said. “I might look at cracks and say that if cracks develop faster than a certain speed, then it’s a warning,” and the data could be sent to an engineer who could tell if the crack warranted more investigation.
What’s more, the technology has other potential uses because anything captured in the video -- dead trees, tall grass, invasive plant species and illegal trash dumping, for instance -- can be analyzed and used by the city and county to take action.
Other locations can also benefit. For example, students in a Policy Innovation Lab class co-taught by Metro21 Executive Director Karen Lightman took the landslide monitoring idea to municipal leaders in Westmoreland County, Pa.
“This rural county, they don’t have the resources that Allegheny County has. They don’t have the GIS sophistication that Allegheny County has, so they wanted recommendations of ‘what can we do,’” Lightman said. “There was such interest that the county commissioners asked the students to present to them at a commission meeting [and] … they’re putting forward a proposal for funding to help study this problem.”