Confidence, scope, scalability challenge military AI
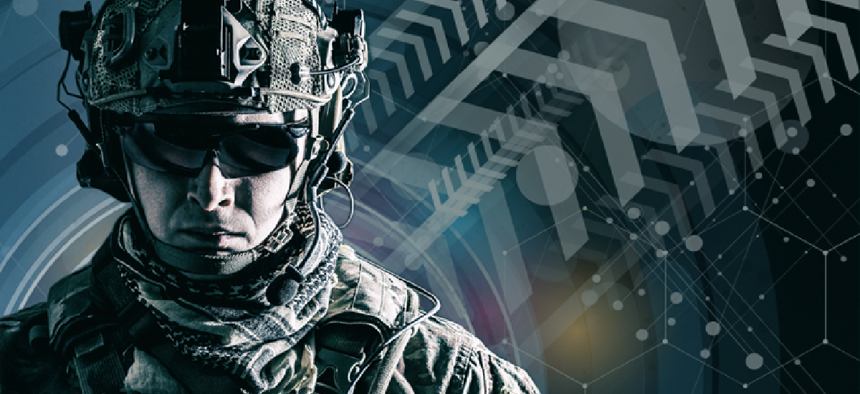
Connecting state and local government leaders
As the Defense Department shifts from manual and human-based decision-making to automated, machine-led analysis, it is uncovering new challenges to using the technology.
To help sift and synthesize massive amounts of network, video and sensor data it collects, the Defense Department is racing to test and adopt artificial intelligence and machine learning solutions and uncovering new challenges to using the technology in modern warfare.
The broader shift from manual and human-based decision-making to automated, machine-led analysis presents new challenges. For example, analysts are used to discussing their conclusions in terms of confidence levels, something that can be more difficult for algorithms to communicate. The more complex the algorithm and data sources it draws from, the trickier it can be to unlock the black box behind its decisions.
"When data is fused from multiple or dozens of sources and completely automated, how does the user experience change? How do they experience confidence and how do they learn to trust machine-based confidence?" Terry Busch, a division chief at the Defense Intelligence Agency, asked during an Apr. 27 virtual event hosted by Government Executive Media.
The Pentagon has experimented with new visualization capabilities to track and present the different sources and algorithms that were used to arrive at a particular conclusion. DOD officials have also pitted man against machine, asking dueling groups of human and AI analysts to identify the location of an object -- like a ship -- and then steadily peeling away the sources of information those groups relied on to see how it impacts their findings and the confidence in those assertions. Such experiments can help determine the risk versus reward of deploying automated analysis in different mission areas.
Like other organizations that leverage such algorithms, the military has learned that many of its AI programs perform better when they're narrowly scoped to a specific function and worse when those capabilities are scaled up to serve more general purposes.
Nand Mulchandani, CTO for the Joint Artificial Intelligence Center at DOD, said the paradox of most AI solutions in government is that they require very specific goals and capabilities in order to receive funding and approval, but that hyper-specificity usually ends up being the main obstacle to more general applications later on. It's one of the reasons DOD created JAIC in the first place, Mulchandani said, likening his role to that of a venture capitalist on the hunt for the next killer app.
"Any of the actions or things we build at the JAIC we try to build them with leverage in mind," Mulchandani said at the same event. "How do we actually take a pattern we're finding out there, build a product to satisfy that and package it in a way that can be adopted very quickly and widely?"
Scalability is an enduring problem for many AI products that are designed for one purpose and then later expanded to others. Despite a growing number of promising use cases, the U.S. government still is far from achieving desired end state for the technology. The Trump administration's latest budget calls for increasing JAIC's funding from $242 million to $290 million and requests a similar $50 million bump for the Defense Advanced Research Projects Agency's research and development efforts around AI.
Ramping up the technology while finding the appropriate balance in human/machine decision-making will require additional advances in ethics, testing and evaluation, training, education, products and user interface, Mulchandani said.
"Dealing with AI is a completely different beast in terms of even decision support, let alone automation and other things that come later," he said. "Even in those situations if you give somebody a 59% probability of something happening … instead of a green or red light, that alone is a huge, huge issue in terms of adoption and being able to understand it."
A longer version of this article was first posted to FCW, a sibling site to GCN.
NEXT STORY: 3 ways agile taught me to be a better leader