COVIDScholar uncovers nonobvious insights in virus research
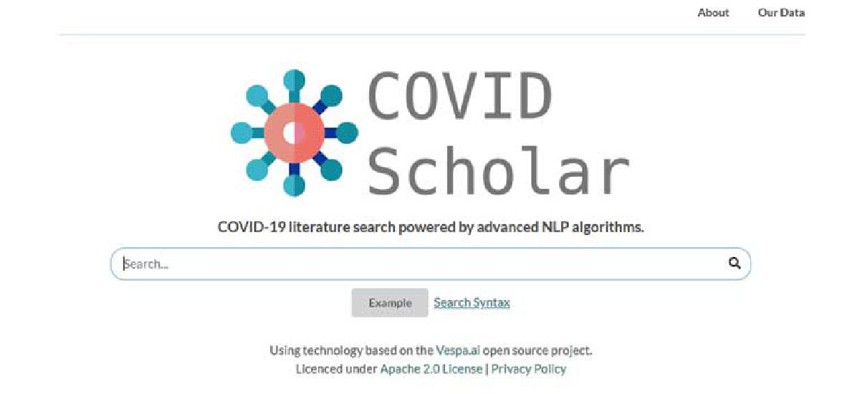
Connecting state and local government leaders
Scientists at Lawrence Berkeley National Laboratory have built a text-mining tool that uses natural language processing to not only scan and search research papers on COVID-19 but also help identify connections that may not be readily apparent.
To help researchers pull insights from the massive number of scientific papers being written on COVID-19, materials scientists at Lawrence Berkeley National Laboratory have built a text-mining tool that uses natural language processing to not only scan and search research papers, but also help identify connections that may not be readily apparent.
COVIDScholar is based on a similar tool developed at Lawrence Berkeley called MatScholar. That tool scanned abstracts of 3.3 million unlabeled papers and extracted 80 million materials-science-related named entities, which were then represented as a database entries in a structured format. Users can query the database “to answer complex ‘meta-questions’ of the published literature that would have previously required laborious, manual literature searches to answer,” the developers explained.
“On Google and other search engines people search for what they think is relevant,” said Berkeley Lab scientist Gerbrand Ceder, one of the project leads. “Our objective is to do information extraction so that people can find nonobvious information and relationships. That’s the whole idea of machine learning and natural language processing that will be applied on these datasets.”
Within about a week of coming up with the idea of adapting MatScholar to COVID-19 research, the Berkeley Lab team got a prototype up and running -- automated scripts grab new papers, clean them up and make them searchable. A little over a month later, COVIDScholar has collected over 61,000 research papers -- about 8,000 of them specifically about COVID-19 and the others abut viruses and pandemics in general.
With 200 new journal articles are being published every day on the coronavirus, more papers are added all the time, often within 15 minutes of the paper appearing online.
The site has been getting more than 100 unique users every day, all by word of mouth, Lab officials said. This week the team released an upgraded version for public use that allows researchers to search for “related papers” and sort articles using machine-learning-based relevance tuning into subcategories, such as testing or transmission dynamics, for specialized searches.
The entire tool runs on the supercomputers of the National Energy Research Scientific Computing Center, a Department of Energy Office of Science user facility located at Berkeley Lab. The online search engine and portal are powered by the Spin cloud container platform at NERSC.
A similar project, CORD-19, is a collection of over 57,000 machine-readable peer-reviewed articles that can be searched with AI-enabled tools. It is designed to help researchers find relevant studies and identify connections between papers and assist developers with building tools to uncover new insights about the virus.