Building a resilience framework from phone location data
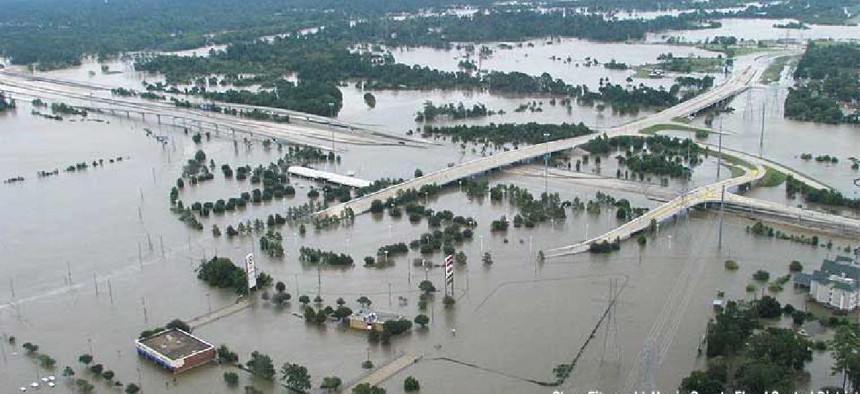
Connecting state and local government leaders
Researchers at Texas A&M University have mined cellphone location data to build a framework that tracks fluctuations in a population's activity before, during and after a natural disaster to determine its level of resilience.
To get better information on how well a community is recovering from a disaster, researchers at Texas A&M University have developed a mathematical framework that tracks fluctuations in a population's activity during a natural disaster to determine its level of resilience.
A community’s resilience, or the ability to bounce back from a disaster, depends on factors like the level of preparedness, the accessibility and distribution of resources and the connections between residents within a community. Traditionally, resilience data is collected through surveys, which only provide general information long after a disaster has occurred, university officials told Texas A&M Today.
Before, during and after Hurricane Harvey, the 2017 storm that dumped between 27 and 54 inches of rain on Houston, the researchers analyzed Harris County residents’ visits to essential businesses like pharmacies, churches and building supply and grocery stores. This data allowed them to see how Harvey disrupted to lifestyles, the built environment and the status of business, they wrote in a paper published in Interface.
The team analyzed anonymized cellphone data collected by SafeGraph, a company that tracks consumers’ visits to particular stores, to obtain location data for Harris County residents around the time of Hurricane Harvey as they visited hospitals, gas stations and stores that would be expected to see more visitor traffic due to the hurricane.
The researchers then mined the data to find the number of visits to each point of interest before and during the hurricane and calculated how long it took before visits returned to the pre-disaster levels.
While it was not surprising to find that communities with low resilience experienced more flooding from the storm, the researchers also found that the level of impact from the hurricane did not necessarily correlate with recovery.
“It’s intuitive to assume, for example, that businesses impacted more will have slower recovery, which actually wasn’t the case,” said Ali Mostafavi, associate professor in the Zachry Department of Civil and Environmental Engineering and director of the Urban Resilience.AI Lab at Texas A&M. “There were places where visits dropped significantly, but they recovered fast. But then others that were impacted less but took longer to recover, which indicated the importance of both time and general resilience in evaluating a community’s recovery.”
By identifying which areas are most affected by a disaster and which can recover fastest, resources can be directed to areas that most need them, he said.
“For federal agencies allocating funds, recovery information is actually needed in a faster and more near real-time fashion for communities that are trailing in the recovery process,” Mostafavi said. “The solution, we thought, was to look for emerging sources of data … that could provide more granular insights into community recovery at a scale not previously investigated.”
Although the researchers focused on Hurricane Harvey, the framework can be applied to other natural disasters, Mostafavi said.