Criminal justice algorithms: Being race-neutral doesn’t mean race-blind
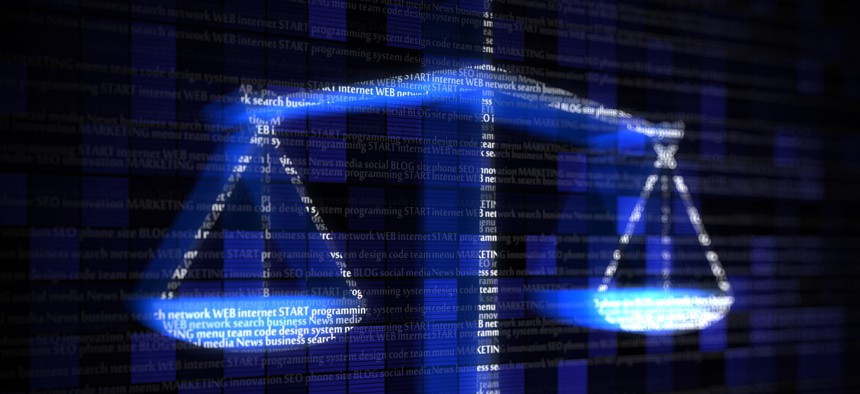
alengo / Getty Images
Connecting state and local government leaders
A risk-assessment tool is aiming to shorten some criminal sentences by predicting potential candidates eligible for early release.
Justice is supposed to be “blind.” But is race blindness always the best way to achieve racial equality? An algorithm to predict recidivism among prison populations is underscoring that debate.
The risk-assessment tool is a centerpiece of the First Step Act, which Congress passed in 2018 with significant bipartisan support, and is meant to shorten some criminal sentences and improve conditions in prisons. Among other changes, it rewards federal inmates with early release if they participate in programs designed to reduce their risk of re-offending. Potential candidates eligible for early release are identified using the Prisoner Assessment Tool Targeting Estimated Risk and Needs, called PATTERN, which estimates an inmate’s risk of committing a crime upon release.
Proponents celebrated the First Step Act as a step toward criminal justice reform that provides a clear path to reducing the prison population of low-risk nonviolent offenders while preserving public safety.
But a review of the PATTERN system published by the Department of Justice in December 2021 found that PATTERN overpredicts recidivism among minority inmates by between 2% and 8% compared with white inmates. Critics fear that PATTERN is reinforcing racial biases that have long plagued the U.S. prison system.
As ethicists who research the use of algorithms in the criminal justice system, we spend lots of time thinking about how to avoid replicating racial bias with new technologies. We seek to understand whether systems like PATTERN can be made racially equitable while continuing to serve the function for which they were designed: to reduce prison populations while maintaining public safety.
Making PATTERN equally accurate for all inmates might require the algorithm to take inmates’ race into account, which can seem counterintuitive. In other words, achieving fair outcomes across racial groups might require focusing more on race, not less: a seeming paradox that plays out in many discussions of fairness and racial justice.
How PATTERN works
The PATTERN algorithm scores individuals according to a range of variables that have been shown to predict recidivism. These factors include criminal history, education level, disciplinary incidents while incarcerated, and whether they have completed any programs aimed at reducing recidivism, among others. The algorithm predicts both general and violent recidivism, and does not take an inmate’s race into account when producing risk scores.
Based on this score, individuals are deemed high-, medium- or low-risk. Only those falling into the last category are eligible for early release.
The DOJ’s latest review, which compares PATTERN predictions with actual outcomes of former inmates, shows that the algorithm’s errors tended to disadvantage nonwhite inmates.
In comparison with white inmates, PATTERN overpredicted general recidivism among Black male inmates by between 2% and 3%. According to the DOJ report, this number rose to 6% to 7% for Black women, relative to white women. PATTERN overpredicted recidivism in Hispanic individuals by 2% to 6% in comparison with white inmates, and overpredicted recidivism among Asian men by 7% to 8% in comparison with white inmates.
These disparate results will likely strike many people as unfair, with the potential to reinforce existing racial disparities in the criminal justice system. For example, Black Americans are already incarcerated at almost five times the rate of white Americans.
At the same time that the algorithm overpredicted recidivism for some racial groups, it underpredicted for others.
Native American men’s general recidivism was underpredicted by 12% to 15% in relation to white inmates, with a 2% underprediction for violent recidivism. Violent recidivism was underpredicted by 4% to 5% for Black men and 1% to 2% for Black women.
Reducing bias by including race
It is tempting to conclude that the Department of Justice should abandon the system altogether. However, computer and data scientists have developed an array of tools over the past decade designed to address concerns about algorithmic unfairness. So it is worth asking whether PATTERN’s inequalities can be remedied.
One option is to apply “debiasing techniques” of the sort described in recent work by criminal justice experts Jennifer Skeem and Christopher Lowenkamp. As computer scientists and legal scholars have observed, the predictive value of a piece of information about a person might vary depending on their other characteristics. For example, suppose that having stable housing tends to reduce the risk that a former inmate will commit another crime, but that the relationship between housing and not re-offending is stronger for white inmates than Black inmates. An algorithm could take this into account for higher accuracy.
But taking this difference into account would require that designers include each inmate’s race in the algorithm, which raises legal concerns. Treating individuals differently on the basis of race in legal decision-making risks violating the 14th Amendment of the Constitution, which guarantees equal protection under the law.
Several legal scholars, including Deborah Hellman, have recently argued that this legal concern is overstated. For example, the law permits using racial classifications to describe criminal suspects and to gather demographic data on the census.
Other uses of racial classifications are more problematic. For example, racial profiling and affirmative action programs continue to be contested in court. But Hellman argues that designing algorithms that are sensitive to the way that information’s predictive value varies across racial lines is more akin to using race in suspect descriptions and the census.
In part, this is because race-sensitive algorithms, unlike racial profiling, do not rely on statistical generalizations about the prevalence of a feature, like the rate of re-offending, within a racial group. Rather, she proposes making statistical generalizations about the reliability of the algorithm’s information for members of a racial group and adjusting appropriately.
But there are also several ethical concerns to consider. Incorporating race might constitute unfair treatment. It might fail to treat inmates as individuals, since it relies upon statistical facts about the racial group to which they are assigned. And it might put some inmates in a worse position than others to earn early-release credits, merely because of their race.
Key difference
Despite these concerns, we argue there are good ethical reasons to incorporate race into the algorithm.
First, by incorporating race, the algorithm could be more accurate across all racial groups. This might allow the federal prison system to grant early release to more inmates who pose a low risk of recidivism while keeping high-risk inmates behind bars. This will promote justice without sacrificing public safety – what proponents of criminal justice reform want.
Furthermore, changing the algorithm to include race can improve outcomes for Black inmates without making things worse for white inmates. This is because earning credits toward early release from prison is not a zero-sum game; one person’s eligibility for the early release program does not affect anyone else’s. This is very different from programs like affirmative action in hiring or education. In these cases, positions are limited, so making things better for one group necessarily makes things worse for the other group.
As PATTERN illustrates, racial equality is not necessarily promoted by taking race out of the equation – at least not when all participants stand to benefit.
Duncan Purves, Associate Professor of Philosophy, University of Florida and Jeremy Davis, Postdoctoral Associate, University of Florida
This article is republished from The Conversation under a Creative Commons license. Read the original article.
NEXT STORY: How agencies can build data literacy