Why big data isn’t enough for law enforcement: Investigating with critical thinking
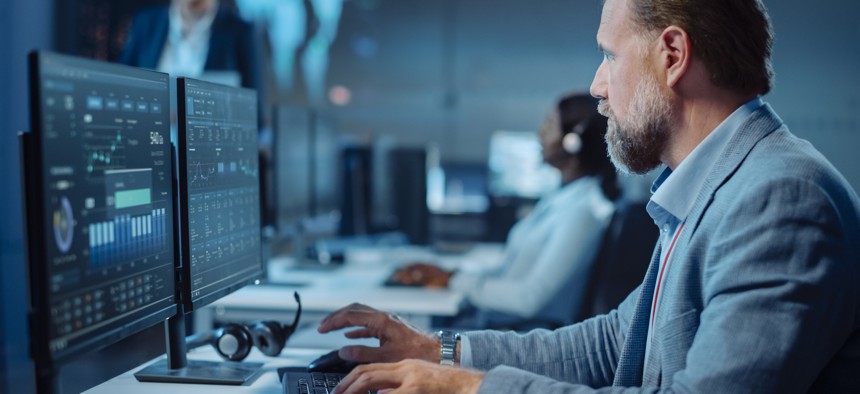
gorodenkoff/Getty Images
Connecting state and local government leaders
Combining decision intelligence methodologies with big data, AI and machine learning can help analysts make sense of data and analytics insights.
There’s a misconception that big data is the main thing that law enforcement needs for effective decision making. Many also assume that big data is flawless and that more data means better data. In fact, big data used for law enforcement investigations and intelligence analysis is often contradictory, partial, biased, non-transparent or even manipulated. To make sense of big data, law enforcement agencies often use a decision intelligence platform for data fusion and analytics. But even that’s not enough.
Once the data analysis process begins, analysts must also determine if they have an intelligence gap. And how do they learn what they don’t know? Proven decision intelligence methodologies—based on critical thinking skills—are required to validate assumptions and make the best decisions with the intelligence at hand.
Benefits and challenges of using technology for data-driven decision-making
Law enforcement agencies gather big data for an investigation by bringing in more and different sources of data, both structured and unstructured. The amount of data and the process of sifting through it can be overwhelming, so the use of technology is imperative. Agencies can use data fusion to enable entity resolution from disconnected data sources and leverage artificial intelligence and machine learning to enrich unstructured data for more effective, automated analytics that improve decision intelligence. AI and ML can also surface previously hidden relationships and patterns between individuals and/or entities; however, these applications are only part of the solution.
On its own, ML is not useful. ML requires human expertise to learn and generate better insights. While it makes sense to apply ML to big data to accelerate decision-making and to find similarities, commonalities and patterns, analysts must still examine the suspicious patterns or irregularities found and what they may indicate. It is a best practice to apply critical-thinking methodologies after uncovering those unique data points that analysts seek. Machine learning should never replace law enforcement analysts. Rather, analysts benefit from the accelerated insights machine learning provides, and then the ML algorithms learn from the analysts’ know-how and experience as inputs.
Critical thinking is a force multiplier for decision intelligence
There are two concepts in critical thinking that reveal the challenges analysts face in critical thinking: failure of imagination and failure of conception. With failure of imagination, analysts have trouble imagining things that they haven’t previously experienced. One example of this is the United States’ inability to imagine a world without the Soviet Union. Despite all the economic and political indicators that pointed to the Soviet Union’s collapse, it remained inconceivable to most. A more recent example is the United States’ failure to predict the rapid Taliban takeover of Afghanistan prior to the final withdrawal of American troops, along with the (incorrect) assessment that the Afghan military and government would be able to maintain control of the country.
Failure of conception is the inability to accept data that conflicts with beliefs and past experiences. The 9/11 attacks are an example of this because many in the U.S. intelligence community believed that terrorism was not a domestic issue—despite those same intelligence organizations knowing that there were foreign nationals learning to fly planes but uninterested in learning how to land. In addition, the intelligence community’s experience was that all of Osama bin Laden’s previous attacks were outside the U.S.
To overcome failure of imagination and failure of conception in investigations, it is wise to apply proven decision intelligence methodologies that can help tackle problems and make sense of data and analytics insights. Here are a few examples:
- Back casting. Imagine starting a movie with its closing scene and then going backwards in time to try to understand who the murderer is, who the hero is and what initiated the sequence of events. The back casting methodology enables analysts to create a decision tree and hypothesize the outcome.
- Competing possibilities. When analysts have a difficult time assessing what will happen, the use of competing possibilities avoids biased conclusions (confirmation bias) and enables analysts to examine the different parameters that support each of the possibilities and note the indicators that will confirm or disconfirm a hypothesis in the future.
- Red cells. Named for government teams that tested the effectiveness and vulnerabilities of military bases, this intelligence methodology requires reviewing the data in detail—with a proverbial magnifying glass—to uncover mistakes. Analysts adopt the premise that mistakes were made but have not yet been found.
- Devil’s advocate. In this methodology, analysts take a position that tests the strength of the opposing arguments, forcing more discussion and consideration.
- Wargaming. In wargaming, analysts take on the role of “players” in a scenario to simulate the decision-making process to provide insights and risk assessment.
The applications of these methodologies are rooted in military intelligence; however, they can and should be applied in law enforcement as well. They can be used in many domains, including financial investigations, customs cases, cybercrime investigations and more. Why? Because these decision intelligence methodologies help analysts realign how they view the data. These methodologies should be used in combination with big data analysis, AI and machine learning to ensure the right results.
It can be difficult to decide which methodologies are best suited to an investigation and to then work through them. Experts in decision intelligence methodologies can guide law enforcement agencies to make the most of their big data, data fusion and analytics. They can assist in testing assumptions and looking at the data and insights from different angles, all to ensure the best outcomes in investigations.
Omer Frenkel is product marketing manager at Cognyte, and Noam Zitzman is chief of intelligence methodology at Cognyte.
NEXT STORY: Lawmakers Introduce Bill to Ban TikTok in US