How to craft fair, transparent data-sharing agreements
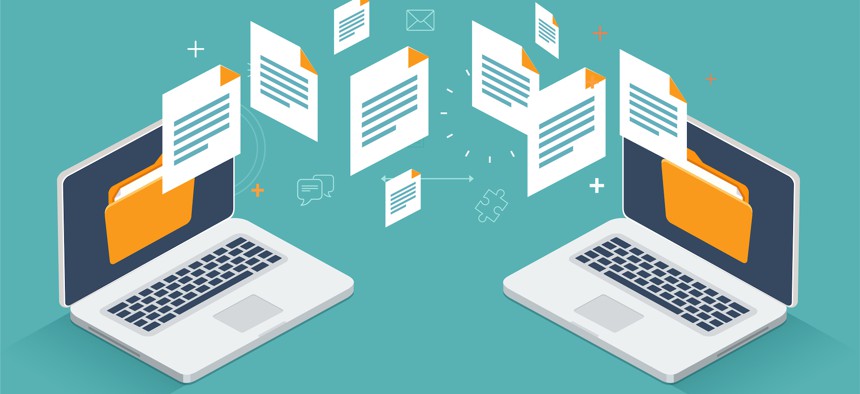
Megapixel8 via Getty images
Effective decision making often requires a complex process of gathering data from different sources. A new framework for data-sharing agreements streamlines the process.
Data collaborations are critical to government decision-making, but actually sharing data can be difficult—not so much the mechanics of the collaboration, but hashing out the rules and policies governing it. A new report offers three resources that will make data sharing more straightforward, foster accountability and build trust among the parties.
“We’ve heard over and over again that one of the biggest barriers to collaboration around data turns out to be data sharing agreements,” said Stefaan Verhulst, co-founder of the Governance Lab at New York University and an author of the November report, “Moving from Idea to Practice.” It’s sometimes a lot to ask stakeholders “to provide access to some of their data,” he said.
To help, Verhulst and other researchers identified three components of successful data-sharing agreements: conducting principled negotiations, establishing the elements of a data-sharing agreement and assessing readiness.
To address the first, the report breaks the components of negotiation into a framework with four tenets: separating people from the problem, focusing on interests rather than positions, identifying options and using objective criteria. From discussions with stakeholders in data sharing agreement workshops that GovLab held through its Open Data Policy Lab, three principles emerged—fairness, transparency and reciprocity.
The result is a checklist agencies can use in negotiations. It calls for steps like defining what assets each party brings to the table and considering how compliance with an agreement can be guaranteed.
Second, the report explains how to balance out what Verhulst said is often an asymmetry between those who have data and those who need it. First developed by GovLab in 2019, the “contractual wheel of data collaboration” provides seven specific steps “that organizers can follow in order to develop a data sharing agreement that meets the needs of all partners,” the report states.
The steps include defining the problem the data will solve, the value proposition of the data, who will be involved, how sharing will happen, what boundaries are necessary and where the data will be shared.
The third resource is a readiness matrix stakeholders can use to determine if they are prepared to share data. The report provides a table with 10 defining conditions for a successful data-sharing agreement and a ranking system to evaluate data holders’ maturity across each.
“What we hope to do here is to demystify this whole area because most of the time, it’s all legalese, as opposed to common sense language,” said Verhulst, who’s also director of GovLab’s Data Program. Each resource in the report is designed to fit together, he added: Determine the principles for negotiation, figure out what the data sharing agreement must entail for those participants and establish readiness to share and work cooperatively.
Data sharing models are increasingly important in today’s data-driven environment, said Daniel Castro, director of the Center for Data Innovation.
“It used to be that for a particular problem, you needed a particular dataset and that dataset could possibly be held and collected by one entity,” Castro said. “Increasingly, what researchers are looking for are datasets that are real-time [and] that involve data that really needs to be aggregated from multiple sources.”
“Exploring Data-Sharing Models to Maximize Benefits From Data,” which the center published on Oct. 16, looks at the pros and cons of six models. Data-sharing partnerships with several collaborators are used to conduct research, develop new products and enhance evidence-based decision-making. They “leverage the collective expertise, resources and data holdings of multiple parties to address complex questions and generate valuable insights.” These large collaborative projects have benefits such as protecting sensitive information of individuals, but also constraints, such as varying quality of datasets.
No one collaboration model is better suited toward one type of data, Castro said. Instead, decisions tend to be based on who has the data and what their motivations are for sharing it. “For example, if there isn’t a lot of trust, maybe the solution is to use something like federated data analytics, where all the processing happens in a distributed fashion,” he said. “Instead of centralizing the data in one place, everyone retains their own.”
The explosion of artificial intelligence, especially generative AI, in the past year highlights the necessity of data sharing, both experts said.
Verhulst said GovLab is looking for ways to use generative AI to make open data more shareable and easier to use.
“At the moment, if you want to use open data, you have to go to an open data portal, you have to download whatever file in whatever standard it is, hopefully, through an API, but then you have to make sense of it,” he said. “Can we actually use generative AI to have more of a conversational experience with open data?”
“So much of AI is of course dependent on the datasets that are available to train these models,” Castro added. “Government is going to increasingly recognize that they have a very big role to play in creating datasets that are reflective of their communities…. There are so many datasets that the government holds or has access to or helps create and has some linkage to,” he said. “It has a responsibility, I think, to try and make that data available.”
Stephanie Kanowitz is a freelance writer based in northern Virginia.
NEXT STORY: AI could make cities autonomous, but that doesn’t mean we should let it happen