No shortcuts: Only well-managed AI will deliver on its promise
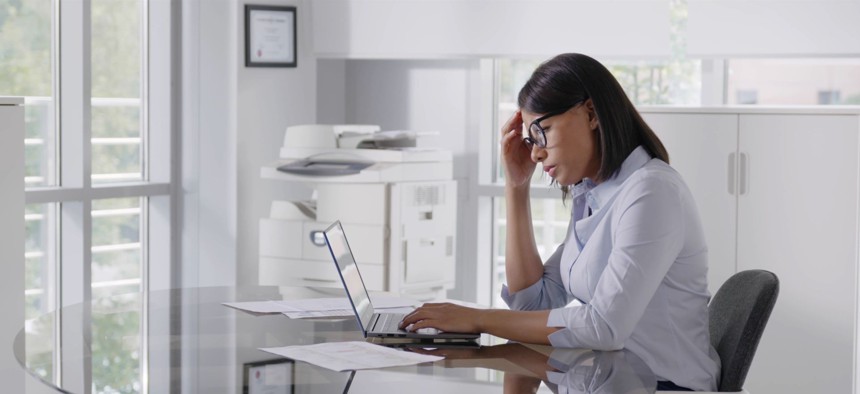
Nimito/GettyImages
COMMENTARY | Artificial intelligence opens new opportunities for policymaking and service delivery, but success still depends on understanding residents’ needs and on the thoughtful handling of their data.
From the White House to city hall, federal, state, local and nonprofit leaders see many exciting opportunities ahead for artificial intelligence-enhanced policymaking and service delivery. Experts believe AI may soon become like GPS: a technology so integrated into daily life that we won’t remember how we navigated without it.
AI’s benefits have been known for some time now. Whether the task is identifying at-risk youth for enhanced services, giving high school students advice on applying to college or summarizing unstructured responses to open-ended survey questions, AI is already proving to be extremely useful. And although AI is helping government workers use data to evaluate social programs more efficiently and develop software to serve more people in better, more personalized ways, it’s not magic. Success in program design and service delivery still depends on understanding the power and limitations of the tool being used and on the careful handling and understanding of the data being examined.
The Promise and Perils of GenAI
Generative AI tools can help create more equity in education. For example, the Washington Student Achievement Council has developed a chatbot, OtterBot, designed to give students timely advice, guidance and motivation through the college application process.
Gen AI can also help reduce the burden on government officials as they review voluminous comments from the public regarding proposed regulations. One new tool for categorizing and summarizing such comments is already deployed in the field, saving people hundreds of hours of work.
But like any powerful tool, Gen AI should be used judiciously:
Watch for bias. AI trained on data that has some latent bias can reinforce that bias. If the data used to build a predictive model in a criminal justice project, for example, is drawn from records in a system with a history of racial discrimination, the model may reflect that history. Discrimination in social policy can result not only from deliberate action, but also from unspoken assumptions and practices that have been baked into systems. Responsible use of data—including data analyzed via AI—should question, rather than reinforce, those biases.
Check for accuracy. ChatGPT, currently the most popular Gen AI program, generates text based on the likeliest response, somewhat like auto-complete on a smartphone. Often, that likely response will be the correct one—but not always. Developers have not found a 100% foolproof way around these errors yet, although systems are improving. For now, AI-generated text, especially when used to deliver important services, must undergo human review. And for the foreseeable future, it will remain important to keep a “human in the loop.”
Monitor impact on staff. Gen AI will likely eliminate some entry-level jobs, which could exacerbate the challenge of finding work, especially for those facing other employment-related disadvantages. In IT, for instance, it’s unclear how entry-level workers will gain the experience they need to move to more advanced projects if there is less demand for humans on help desk duty. As a result, workforce training programs will need to pivot in response to the latest economic and technological shifts, and social policies must help distribute new job opportunities fairly and effectively.
Six Tips to Get AI Right
How should a government administrator or service provider integrate AI into agency programs? For starters, don’t think of AI as an “easy button.” It’s a powerful tool, but it still needs to be managed. Here are six strategies that can help:
1. Think it through. Before diving in, make sure that AI makes sense for the problem you are trying to solve. Develop a theory of change. Socialize the idea. Does the idea involve some kind of repeatable process? Is there the prospect for personalization or tailoring? Does it seem plausible that people will engage with the tool?
2. Start with a pilot program. It’s difficult to know in advance what will work, what won’t, and what the unintended consequences might be, so agencies should start small. That allows leaders to validate a program’s safety and effectiveness before they scale it—or determine that scaling is not worthwhile. For example, my organization tested whether machine learning could improve intake procedures at Per Scholas, a nonprofit that trains low-income people for skilled tech jobs. The pilot found that the models were not sufficiently reliable to use. Similarly, analysts concluded that AI-based algorithms could help prioritize high-needs cases in some workforce development programs, but that they involved tradeoffs—in increased cost, complexity and risk of bias—that made simpler methods “good enough.”
3. Keep analyzing system performance. Ideally, agencies should use quantitative methods, such as Sankey data flow analysis, and complementary qualitative methods—particularly customer journey maps—to observe how participants engage with technology platforms.
4. Evaluate different aspects of the program with rapid A/B testing. When one group receives the program’s original application while the other receives a new iteration, it’s easy to see whether the new version is actually better. This iterative testing is not geared toward building evidence for the field as a whole, but it can improve individual AI programs or processes much more quickly.
5. Make sure data is collected and used in ethical, auditable ways. At a minimum, a diverse review board should oversee that process. But it’s best to go further by creating internal policies, resources and systems that explain agency values and how they shape the approach to data.
6. Understand context. Creating a “participant advisory board” of people personally affected by social programs and policies can help ensure the answers that AI generates are on target, and that the right questions are being asked. People with different perspectives and backgrounds can make sure the right questions are asked, findings are interpreted correctly, and services designed to be the most helpful.
In the end, AI really is like GPS: In most circumstances, it will help agencies reach their destination sooner, with less anxiety. All the same, government leaders will need to keep their eyes on the road. Even if GPS says to turn right, if the hill ahead is covered in ice, it’s smart to take a different route. In short: with AI, as with GPS, it remains important to know the terrain, or travel with a human companion who does.
Richard Hendra is the director of the Center for Data Insights at MDRC, a nonpartisan social policy nonprofit celebrating its 50th anniversary this year.