Predicting traffic congestion from cell signals
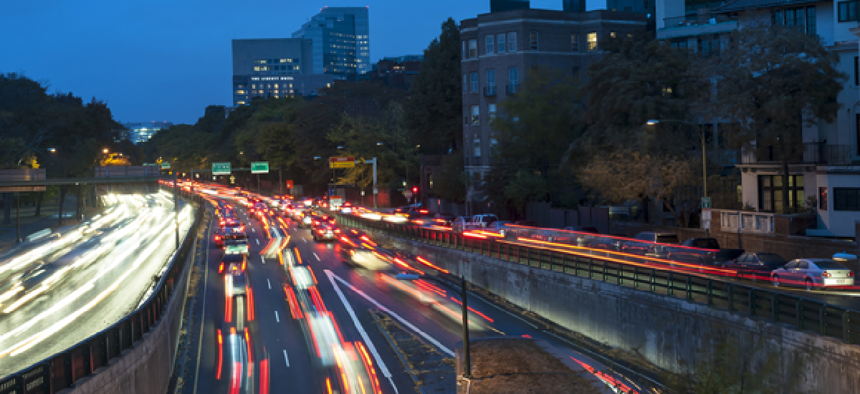
Researchers at MIT are working on a tool that may help city planners and traffic managers better anticipate potential traffic problems by analyzing cellphone signals.
There are few things as frustrating -- and as bad for the environment -- as gridlocked traffic. Researchers at MIT are working on a tool that may help city planners and traffic managers better anticipate potential problems by analyzing cellphone traffic.
While that may seem like small potatoes -- given that smartphone apps such as Waze have for several years been collecting data from smartphones and sending out real-time traffic reports -- the MIT team aims to collect a broader sampling than just data from smartphones that have a certain app installed.
“A lot of people are using the cheapest cell phones, not smartphones,” said Marta Gonzalez, associate professor of civil and environmental engineering and the project’s team leader. That means depending on app-generated data doesn’t paint a full picture of traffic.
So instead of relying on a smartphone app to collect location data, the MIT team accessed and then analyzed data collected by cell service providers from all cellphones when they interact with cell towers. “With that we can triangulate to get an approximate location of the phone,” Gonzalez said.
After receiving six weeks of data from 192 million devices in the Boston area from a commercial provider – Airsage – the team applied its own algorithms to analyze travel patterns. The algorithm assumes, for example, that if a specific device communicates consistently from the same location early in the morning and then again in the evening, that that location is “home.” Similarly, if the device communicates during the day from a consistent location for extended periods, the user is likely at work.
While also recording location data between home and work, the researchers divided each day of the week into 10-minutes slices, then applied a predictive model to project the likelihood of users changing locations, their possible destinations and the length of time that would be spent at each destination.
The MIT project isn’t designed to accurately track or predict the locations of individuals -- Gonzalez stressed that all the data is anonymized. In the aggregate, however, the data can be used to quickly build near-real-time models of urban mobility patterns.
The technology could replace surveys of individual residents about their travel patterns, which tend to result in significant lag times and force planners to model with mobility patterns that may have already changed. In fact, Gonzalez noted that Boston is still generating mobility models from survey data gathered in 1994.
In contrast, the MIT project employs data that is received in real time. The group’s algorithm then requires about 30 minutes to run.
Using only triangulated data from cell towers, Gonzalez’s team can’t infer individuals' mode of travel. Also, she said, “We can tell when a phone passes by location, but we do not have enough accuracy to determine speed.”
Despite these limitations, Gonzalez said that the emphasis in this first iteration is on getting as broad a dataset as possible. “We wanted to be sure to get the largest number of users possible,” she said.
In the future, the team would like to add data that can be inferred from the many sensors, including GPS receivers, that are available on smartphones. “Apps on smartphones are going to be a complement to this,” Gonzalez said. Additionally, she hopes to cut down the processing time to make the results available even faster.
The team is also developing an application for event planners that will deliver estimates of travel demands during the Olympic Games or large concerts.