In search of better flu forecasting
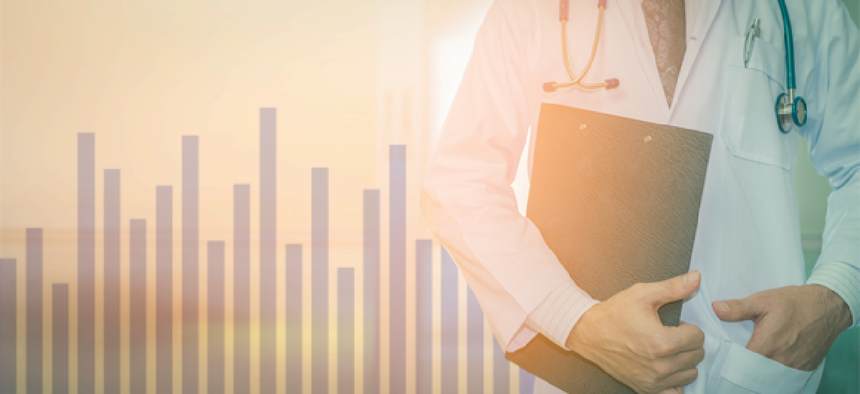
Connecting state and local government leaders
The Centers for Disease Control and Prevention is working with 21 research teams to develop flu forecasting capacity in the United States.
The Centers for Disease Control and Prevention recently released its weekly flu forecasts for the 2016-2017 season on FluSight, a beta website housing influenza activity forecasts provided by 21 research teams.
The research initiative was begun by the CDC in 2013. "Predict the Influenza Season Challenge" is a public contest to encourage people from around the world to predict the timing, peak and intensity of each year's flu seasons using social media data alongside data from the organization's own flu "surveillance" systems. The latter parses reports describing flu-like symptoms from doctor offices and health clinics. Accurate flu predictions help public health officials and other healthcare professionals schedule their vaccination campaigns, issue flu information and make staffing decisions.
Last year, Carnegie Mellon University’s Delphi research group contributed the three most accurate national-level flu forecasts during the 2015-2016 flu season, besting the forecasts of 10 other external groups working with CDC.
At CMU, the research team's top-ranked forecasting system uses machine learning to make predictions based on historic and current data from the CDC. Its second-ranked system uses weekly predictions fed by ordinary people into the "Influenza Edition" of Epicast. Each week, registered users spend a couple of minutes predicting current and future flu activity within one or more of the various health and human services regions of the United States.
This human-powered approach was actually the top-ranked forecasting system for the 2014-2015 flu season, said Roni Rosenfeld, professor in the School of Computer Science's Machine Learning Department and Language Technologies Institute, in a CMU News article about the work. "Any one human did not do better than the statistical system," he emphasized. "They did worse. But in the aggregate, the human system did better that season."
This year, the team is hoping to beat its record.
They'll be monitoring the results of various approaches to figure out which one is more accurate: using artificial intelligence and machine learning, or crowdsourcing the predictions. What they learn from the process isn't limited to the flu, however, as their work can be applied to predict other kinds of outbreaks.
"Our predictions last season proved to be reasonable, but the truth is that when it comes to forecasting epidemics, whether it be for the flu or for other diseases, we're just getting our feet wet," Rosenfeld said.
It's too early to tell how the forecasting will turn out this year. The season typically runs from October through May. Due to reporting lags, however, the true flu activity levels won't be known until the season is done.
While the researchers are refining their prediction methods and models on the flu, they're also applying their systems to forecasting outbreaks of dengue fever, which strikes about 100 million people around the world each year, killing thousands, according to the CDC. Eventually, the team would also like to apply its forecasting efforts to HIV, drug resistance, Ebola, Zika and Chikungunya.
This article was first posted to Campus Technology, a sister site to GCN.