Machine learning: The good, the bad and the ugly
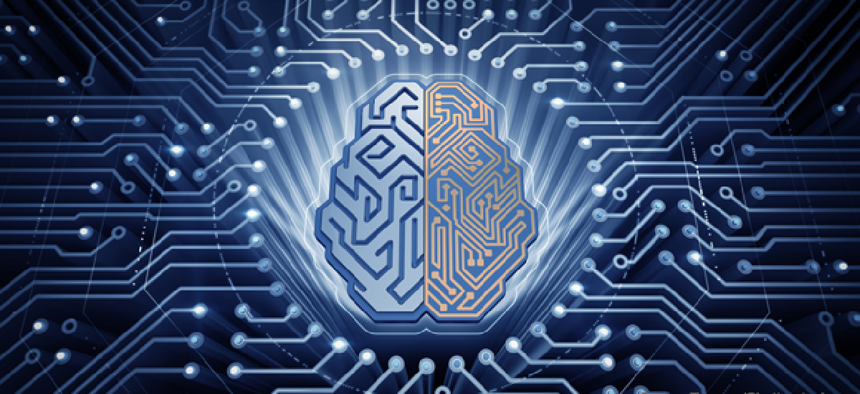
Connecting state and local government leaders
While machine learning might be the enabling technology for the future, for the Intelligence Advanced Research Projects Activity, it's old school.
While machine learning might be the enabling technology of the future, for the Intelligence Advanced Research Projects Activity, it's old school.
Founded in 2006 to drive research and innovation within the federal government’s intelligence agencies, IARPA has been researching machine learning from the beginning.
“Machine learning has been a priority research area since we were created 10 years ago,” IARPA Director Jason Matheny said. “In fact, most of our first programs were in machine learning.”
Some of these early efforts include the Biometrics Exploitation Science and Technology program, which developed tools for facial recognition that have since been widely adopted. Aladdin Video, for example, was an effort to identify actions in streaming video. It could "tell whether this is a video of a birthday party, or a video of someone break dancing, or a video of somebody describing how to build an explosive device,” Matheny said. Other programs focused on natural-language processing.
These projects laid the foundation for the use of machine learning in more complex applications, such as predicting cyberattacks based on chatter in hacker forums and the market price of malware; forecasting military mobilization and terrorism; and developing accurate 3-D models of buildings or entire cities from satellite imagery.
But IARPA is also researching ways of improving the fundamental architecture upon which machine learning is built: the neural network, “a very rough approximation of how we thought the brain worked in the 1950s," Matheny explained. "Our machine learning approaches, in general, haven’t caught up with neuroscience.”
One effort to close this gap is the ongoing Machine Intelligence from Cortical Networks program that seeks to reverse engineer the algorithms of the brain. In its first year, MICrONS has developed the largest dataset of wiring diagrams of the circuits responsible for learning in animal brains, which, at this point, are much better than machines at learning.
“Not only are they able to learn from a much smaller number of examples than typical machine learning systems, but they do so with much less energy -- about one one-millionth of the amount of energy in typical computers,” Matheny said.
The agency is also in the early stages of looking at what quantum computing will mean for machine learning.
There are two different kinds of quantum computing, Matheny said. One variety, gate-based, is years away from being a viable technology. The other, quantum annealing, could prove useful in the shorter term by solving optimization problems that could improve machine learning -- like finding the shortest path between two points. But the research is still fairly new, he said.
All of these projects rely on having quality data to train the program. Anyone building an application that relies on machine learning must first understand how the program will be used and then “make sure the training data is representative of the data the tool would be applied to -- and representative in a statistically sound way,” Matheny added.
This training could become even more important in combating machine learning’s dark side: spoofing, or adversarial machine learning, he said. Spoofing changes a select few pixels in an image. Humans might not notice the change, but image recognition systems can be tricked into misclassifying a school bus as an ostrich. There are many examples of researchers duping image recognition systems, like the MIT researchers who recently made one system think a picture of a gun was a helicopter, according to Wired.
Research on how systems will combat spoofing is in still in development. But having systems “arm wrestle” against “adversarial examples while training” could teach them how to spot such trickery. Another defense is model averaging, “where you don’t use the neural net approach, but you might have a neural net and a support vector machine that’s not vulnerable to the same kinds of attacks,” Matheny said.
But solving this kind of problem will be vital to having machine learning implemented in increasingly important tasks.
“It’s a deep problem in machine learning,” Matheny said of spoofing. “But before machine learning is assigned to doing high-consequence analysis, some of these vulnerabilities need to be fixed.”