Holding algorithms accountable
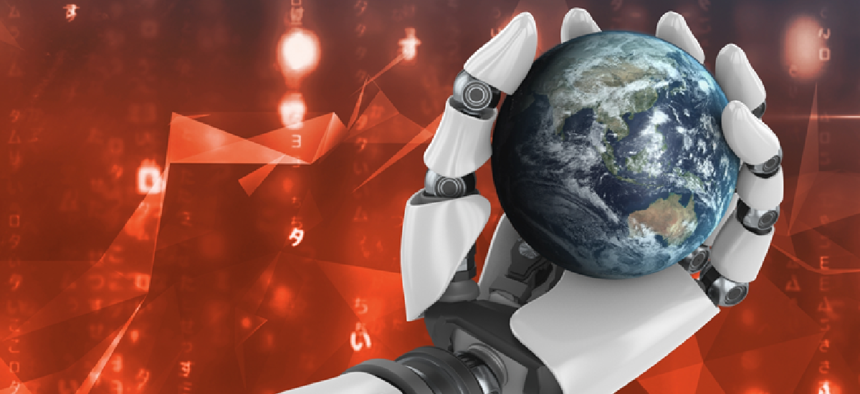
Connecting state and local government leaders
A new report offers some measures for ensuring that algorithms behave as expected and that operators can identify and rectify harmful outcomes.
Algorithms are increasingly being used to make decisions in the public and private sector even though they have been shown to deliver biased outcomes in some cases. Although several methods of governing algorithms have been proposed, a new report from the Information Technology and Innovation Foundation's Center for Data Innovation argued that previous proposals fall short, and it outlined a method of “algorithmic accountability” meant to protect against undesirable outcomes.
Prior efforts to combat bias fall into four categories, CDI said: the algorithmic transparency or explainability mandate, the creation of a regulatory body to oversee algorithms, general regulation and just leaving algorithms alone.
Each of these proposals has faults, the report authors said. If all artificial intelligence must be explainable, for example, then that holds this technology to a higher standard than we apply to human decision making. Meanwhile, there are some kinds of algorithmic implementations that don’t need regulations. Dating apps, the report argued, could result in a bad date, but that doesn't mean they should be regulated.
For CDI, algorithmic accountability has three goals: promoting desirable or beneficial outcomes; protecting against undesirable, or harmful, outcomes; and ensuring laws that apply to human decisions can be effectively applied to algorithmic decisions. Therefore, the authors argued, a governance framework should employ a variety of controls to ensure operators can: verify an algorithm works in accordance with the operator’s intentions and identify and rectify harmful outcomes.
Transparency is one way algorithms can be made accountable. Even with "black box" algorithms, transparency would allow third parties to determine if the software is functioning as intended.
In general, an algorithm should prioritize accuracy over transparency, CDI said. And even when there is transparency, the decision-making processes for machine learning applications is often not understood by the developers themselves. But in some government use cases, like risk assessment algorithms, transparency could be beneficial.
“[R]isk-assessment algorithms, such as those used to inform sentencing decisions, may rely on many different variables in their assessments but be static and relatively straightforward, making it is easy for their operators to assess the variables involved and determine whether they are appropriate -- as well as observe how a certain data point might impact a risk score because the system is hard-coded to give that variable a particular weighting,” the report said.
The report quoted Caleb Watney, a technology policy fellow at the R Street Institute, who argued that because sunshine laws have set a precedent of transparency in the justice system, it could be appropriate to “mandate all algorithms that influence judicial decision-making be open-source.”
The report concluded that it would be reasonable to mandate public-sector agencies go through an “impact assessment” process for any algorithms they plan to use.
New York has taken steps toward that goal. Acknowledging how algorithms are increasingly incorporated into software that makes decisions about school placements, criminal justice or the distribution of social services, New York Mayor Bill de Blasio recently announced plans to set up a task force review the city's automated decision systems for equity, fairness and accountability.
The AI Now Institute at New York University recently released a report on assessing the impact of algorithms that recommends a pre-acquisition review and a chance for public comment.
NEXT STORY: What are these 'levels' of autonomous vehicles?