Using AI to recognize dangerous hailstorms
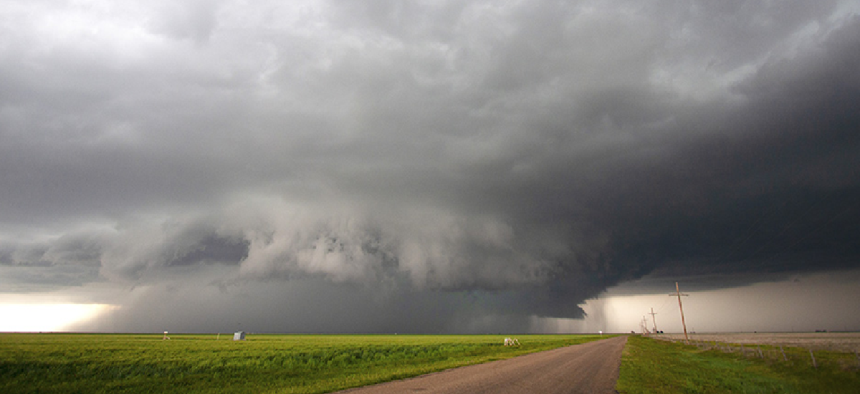
Weather researchers are using facial recognition technologies to analyze approaching storms to determine whether they are likely to produce damaging hail.
Facial-recognition software is already in use at many secure facilities. Now researchers at the National Center for Atmospheric Research (NCAR) are applying similar technologies to examine the “face” of approaching storms to determine whether they are likely to produce damaging hail.
Instead of measuring the distance between facial features -- eyes, noses, lips, etc. -- NCAR scientists are developing a convolutional neural network, a deep-learning model that analyzes the many facets of a storm to determine which combinations result in large, dangerous and potentially costly hailstones.
Whether a given storm is going to unleash the kind of ice balls that crack windshields depends on a complex set of conditions, including colder temperatures and higher humidity near the ground while dryer air prevails higher up. Those factors, combined with strong updrafts that keep small hail in the air long enough to become big stones, are among the major factors.
But it’s not just vertical conditions that matter. Wind directions and speeds also appear to be important.
“The shape of the storm is really important,” said David John Gagne, machine learning scientist at NCAR and leader of the project told NCAR News. “In the past we have tended to focus on single points in a storm or vertical profiles, but the horizontal structure is also really important.”
To analyze the potential for hail, the NCAR team takes 15 “slices” through the storm, measuring five different factors: temperature, geopotential height, dewpoint and east-west and north-south wind components. “We take all of those at three different pressure levels,” Gagne said.
To process all that data, Gagne adapted machine-learning algorithms that were originally designed to analyze visual images and applied them to weather simulations created by NCAR’s Weather Research and Forecasting model.
Once the machine-learning model was trained and showed it could predict the size of hail produced by simulated storms, Gagne reversed the model to determine the main factors correlating with hail being produced by the storm. As it turns out, the most strongly related condition was a storm with strong updrafts. Storms with more circular shapes are also correlated with hail production.
The biggest benefit of the algorithm is reducing the number of false alarms and giving analysts a way to quickly analyze the fire hose of data. “It can eliminate certain areas because the storms aren’t the right shape, or this or that parameter will decrease the threat,” he said.
Gagne said his team is planning to have the machine-learning prediction system -- which doesn’t yet have a name -- processing storm data in real time as early as next spring, with full deployment penciled in for two to three years from now.
The next step in Gagne’s research is to apply the model to actual storm observations and eventually into deployment to help forecasters provide more accurate warnings of potentially damaging hailstorms.
“I think this new method has a lot of promise to help forecasters better predict a weather phenomenon capable of causing severe damage,” Gagne said. “We are excited to continue testing and refining the model with observations of real storms.”