Deep learning predicts emergency resolution time
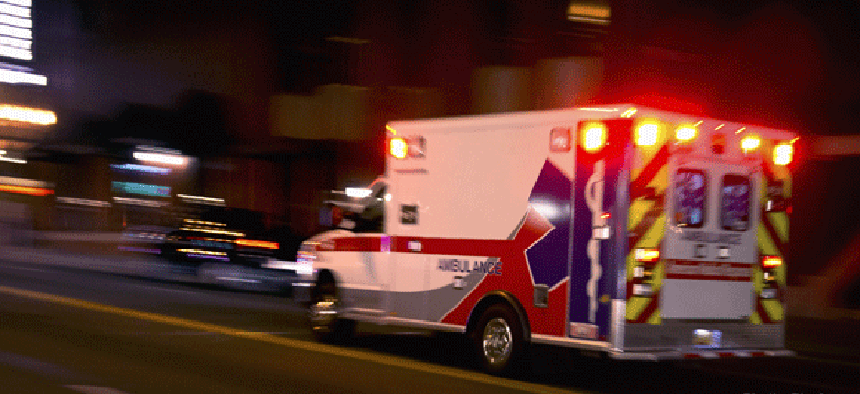
DeepER, a model that uses recurrent neural networks and open data from New York City, predicts how long it takes from the time an incident is reported until it is closed.
A new deep-learning system can help cities predict the time between the reporting and resolution of an emergency.
Researchers at Binghamton University built DeepER, a model that uses recurrent neural networks, to predict the future resolution times of incidents based on historical data. To do this, the system also looks for interdependencies among emergency event types.
The team – comprised of Arti Ramesh and Anand Seetharam, assistant professors in the Department of Computer Science at the Thomas J. Watson College of Engineering and Applied Science, and three students – used eight years of data from New York City’s NYC Open Data portal showing the times incidents were reported and closed.
They preprocessed the data by removing outliers caused by missing data points. For example, the time stamp on closing incidents doesn’t always happen in a timely fashion, Ramesh said. “We have to give it good quality data to look at so that it is actually able to find these real dependencies that exist in the data,” she said.
The researchers also aggregated the data into categories -- fire, law and structural -- and subcategories based on the types of emergencies and the time between report and closure. That’s because similar incident types are more likely to be connected to one another, Ramesh said.
Besides preparing the data, the researchers faced two other main challenges. One was that because it’s nearly impossible to know when emergencies will occur, the time between them could be days or years, making it tough for humans to find patterns, but artificial intelligence can.
The team split the data into two groups: training and testing data. With the training data, DeepER learned how much time each past incident took to resolve and the interdependencies -- how one fire event is related to another fire event, for instance -- and mathematically encoded those.
Then, they introduced the test data -- data DeepER had not seen -- and based on what it learned, it made predictions. After the team told the system how off it was, the system reprocessed the data and corrected its predictions.
“We found that because we trained on past years, it can predict the future pretty well,” Seetharam said. “Specifically, it achieves an average performance improvement of 3% to 16%.”
The project took about a year from when work started to when the team’s paper -- “DeepER: A Deep Learning based Emergency Resolution Time Prediction System” -- was published a few weeks ago.
The next step is to figure out ways to make the information useful to government planners, Ramesh said, and to look at other cities to see how the model can be adapted. The challenges are determining whether cities collect the data in the first place and expanding the model to work in smaller localities.
“To train this kind of model to do an effective prediction, we need lots of data,” Seetharam said. “With respect to smaller cities or smaller towns, they just don’t have those many incidents. They don’t have the same design structure as a city. They’re more spread out, so if you want to get the model to work on a smaller town, mostly what we would have to do is … collect the data from a lot of neighboring towns,” he said. “Together they would have enough data and because geographically they are in a similar area, they would have similar characteristics so then you could have a complete model to train.”
The code is publicly available, so cities that have the data can take DeepER, train it on their data and deploy it to see whether their incident resolution times meet their goals, Ramesh said. If not, they can use the data to drive policy to improve response.
“They can look at whether the predictions are within a desired threshold -- how long it should take and whether they’re comfortable with it or not,” Seetharam said. “They can use the predictions to then hire more people in the future.”
If governments become interested in DeepER, the researchers said, they would look for ways to make it more user-friendly, such as creating an app and website for it to facilitate visualization.