Facial recognition inappropriate for high-risk applications, experts say
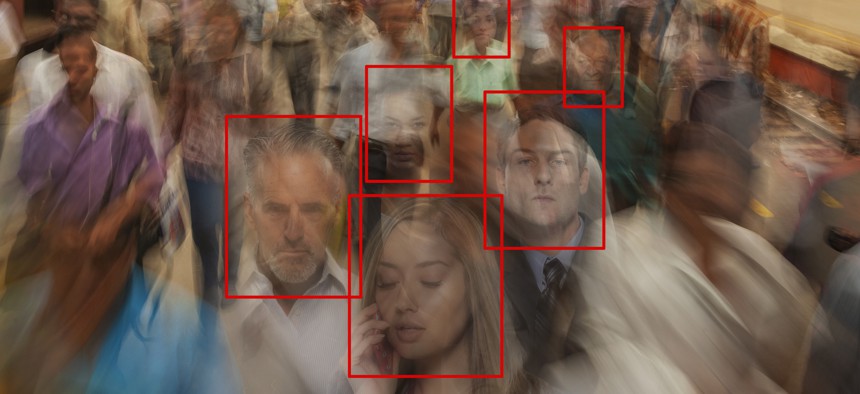
GettyImages/ John Lund
Accuracy, privacy and transparency issues make facial recognition technology too risky for local agencies, according the University of Pittsburgh’s Institute for Cyber Law, Policy and Security.
Use of algorithms can improve government efficiency, but local agencies should avoid using facial recognition and related systems, according to a new report.
There’s potential for harm with facial recognition and related technologies in high-risk applications that, even if accuracy improves, “could result in invasive surveillance that would undermine privacy,” according to the Report of the Pittsburgh Task Force on Public Algorithms. “Governments avoid such systems for the foreseeable future.”
That’s just one of seven recommendations from the task force. Hosted by the University of Pittsburgh’s Institute for Cyber Law, Policy and Security (Pitt Cyber), the group formed in 2020 to study local government’s use of algorithms with the understanding that although they can bring many benefits, they “carry risks that can and should be guarded against,” such as bias and a lack of transparency.
The task force defined a public algorithmic system as “any system, software or processes that uses computation, including those derived from machine learning or other data-processing or artificial intelligence (AI) techniques, to aid or replace government decisions, judgments, and/or policy implementations that impact opportunities, access, liberties, rights, and/or safety.”
“There is evidence that some algorithmic systems can lock in and exacerbate bias and harms (especially along racial and gender lines), leading to more inequity and injustice,” the report stated. Additionally, there are few requirements for “regional governmental agencies to share information about algorithmic systems or to submit those systems to outside and public scrutiny.”
The task force found that many Pittsburgh residents felt kept in the dark about the use of algorithms and were frustrated that government is harnessing data to target enforcement rather than deliver resources. They expressed a desire for more democratic consideration and transparency with algorithmic systems.
The report’s first recommendation is to encourage meaningful public participation in line with the risk level of a potential system. To assess that, it suggested a framework by Gretchen Greene of the AI and Governance Assembly, which identifies risk levels according to “the likelihood of causing serious harm through discrimination, inaccuracy, unfairness or lack of explanation.” For instance, algorithms for infrastructure maintenance and repair are low risk, while those for child protective services are high.
For higher risk applications, the report recommended third-party reviews. They can help identify shortcomings and biases before the system goes live, and they add a layer of visibility into the system that can garner public trust.
Additionally, governments can use procurement frameworks for assessing whether a planned purchase might include an algorithmic system. For example, Pittsburgh’s Department of Innovation and Performance, which handles software acquisition, including algorithmic systems, states in its Data Governance Operational Charter that it remains “committed to ensuring that our usage of data does not in any way infringe upon the privacy or civil liberties of citizens, and that we maintain accountable and bias-free utilization of computational algorithms.”
To further transparency, a group within the task force is creating a website prototype that could be the basis for city and county sites listing the algorithmic systems agencies use. “As was similarly recommended by New York City’s Automated Decision Systems Task Force, local leadership should also consider making the information available in printed form at Carnegie Library branches and other culturally relevant sites, ensuring broad access even where internet access might be limited,” the report added.
But among the riskiest applications of algorithms is in biometrics. For instance, Pittsburgh’s Bureau of Police used a state facial-recognition system called JNET “to match a social-media image to one in the database in order to identify a person they charged with crimes. The bureau apparently disregarded an agency policy that it ‘does not use facial recognition software or programs,’ highlighting the urgent need for more robust oversight of facial-recognition capabilities,” according to the report. After that, Pittsburgh City Council approved a bill in September 2020 to regulate facial-recognition technology.
Community feedback about use of algorithms highlighted concerns. “When the algorithms go wrong, who is at fault?” one person asked, according to the report.
“I think what happens is a lot of government agencies are just procuring algorithms off the shelf, and people aren’t being trained in the right ways,” Pitt Cyber Executive Director Beth Schwanke told Technical.ly. “Instead of being a tool to our government, they’re becoming a tool that is relied on in an unsafe way…. We should want governments to use data, that’s a good thing. We just want to be doing it the right way.”
“There is a tremendous opportunity in this country to fashion a framework for managing and harnessing public algorithmic systems,” David Hickton, Pitt Cyber founding director, wrote in The Hill. “Our Task Force has offered recommendations that we believe can achieve that goal. We are seizing the initiative in Pittsburgh — and humbly hoping that others will follow our lead.”
Stephanie Kanowitz is a freelance writer based in northern Virginia.