Agencies can accelerate AI deployments with ModelOps
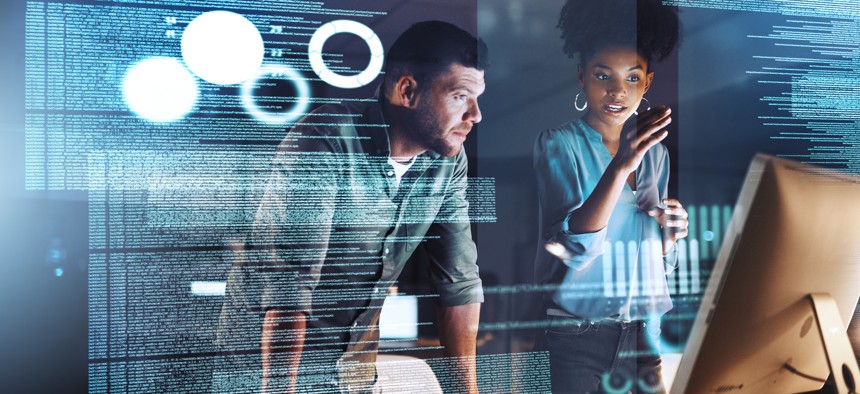
GettyImages/ Jay Yuno
ModelOps helps agencies get models from the lab through validation, testing and deployment phases as quickly as possible while ensuring quality results.
Government agencies at every level are embracing artificial intelligence and machine learning models to turn massive volumes of data into fresh insights and information. A 2021 Gartner CIO survey found that 36% of government respondents planned to increase AI/ML investment in 2021.
AI/ML models can effectively access vast amounts of unstructured data to identify patterns for predictive purposes. Model development and deployment are complex, however. Only about 50% of models are put in production, and those that are, take at least three months to be ready for deployment. This time and effort takes on a real operational cost and results in a slower time to value – luxuries government agencies do not have.
For this reason, ModelOps is gaining attention among agency decision-makers looking to lift the percentage of analytical models that reach deployment and accelerate the time-to-value. Benefiting from ModelOps requires understanding how it works, the critical issues it addresses and how it is already being applied to public sector initiatives.
The importance of modeling
Agencies have turned to DevOps over the past few years to accelerate software development, making the process more agile and collaborative between developer, operations and QA teams. DevOps offers an instructive parallel for the benefits of ModelOps.
Data is the foundation on which models are built. Development must be efficient, automated where possible and explainable in order to drive adoption. Model lineage, version history and track-back information (like features used in decisions, datasets and analytic techniques) should also be preserved for governance purposes. And models must be validated, deployed and monitored for consistency and repeatability to ensure they continue to produce the right insights.
What is ModelOps?
All of this leads toward ModelOps: the discipline of reviewing and monitoring model performance. It is a critical capability for rapidly moving models through the analytics life cycle, so they’re easily deployed, perform as expected, scale improved decision-making and
deliver business value. While DevOps focuses on application development, ModelOps focuses on getting models from the lab through validation, testing and deployment phases as quickly as possible while ensuring quality results.
ModelOps uses long-standing DevOps principles. It’s a must-have for implementing scalable predictive analytics. But to be clear: model development practices are not the same as software engineering best practices. The difference soon becomes apparent.
AI/ML modeling challenges
Organizations build powerful analytic models that don't see the light of day because of the difficulty of operationalizing them. Hiring data scientists, buying new analytics tools, building data warehouses, data marts and lakes can all be core investments. Still, unless an organization has a cohesive analytics platform or is very analytically mature, it can be a struggle to operationalize analytics to keep up with new demands. Open-source tools and new technologies complicate deployment environments further.
Three common modeling challenges should be top-of-mind when operationalizing the model life cycle through ModelOps:
1. Data quality. Subtle changes or shifts in data that might go unnoticed or may have a lesser effect on traditional analytical processes can significantly affect ML model accuracy. As part of ModelOps efforts, it’s important to properly assess the data sources and variables used by models so you can answer the following questions:
- What data sources will be used?
- Would the agency be comfortable telling a congressional oversight that a decision was made based on that data?
- Do the data inputs directly or indirectly violate any regulations?
- How has model bias been addressed?
- How frequently are new data fields added or changed?
- Can feature engineering be replicated in production?
2. Time to deployment. Data scientists tend to focus on building a better model or applying the latest technique. They’re not typically exposed to, nor trained on, the process-centric work required to get an analytic model efficiently into production. Likewise, IT does not typically train on how analytical models are built and how to interpret them. As a result, when faced with complex models created by data scientists, IT teams may have difficulty readying them for deployment, which often leads to rework or simplification by both the data scientist and IT, increasing taxpayer cost and reducing the value provided to the government.
3. Degradation. All models degrade, and performance suffers if they are not given regular attention. Models are like cars: To ensure quality performance, they need regular maintenance. Look out for challenges like drift and bias, which can be addressed by creating a solid approach to model stewardship in the organization. If everyone from model developers to business users takes ownership of the models' health, these problems can be solved before they impact the nation’s health, security or operations.
ModelOps benefits
ModelOps can also help agencies drive innovation, improve collaboration and provide taxpayers with significant time and cost savings. Enterprises and government agencies alike are reaping the rewards of ModelOps in the following ways:
- Accelerated analytics modeling processes. ModelOps allows agencies to move models from the lab to validation, testing and production as quickly as possible while ensuring quality results. It enables them to manage and scale models to meet demand and continuously monitor them to spot and fix early signs of degradation.
- Improved quality of AI/ML models. By encompassing culture, processes and technology, ModelOps enables organizations to efficiently and continuously develop and deploy models, not just to get more of them into production but also to deliver more advanced analytics solutions more often. Operationalizing models creates value sooner while avoiding excess “handoffs,” which create complexity and recoding.
- Better collaboration. Most organizations have a missing link between the teams building and deploying the AI models and the teams overseeing and managing model operations. ModelOps closes the gap by sharing transparent goals for each model, outlining clear processes for both teams and assigning definitive responsibilities to every role involved.
- Better transparency and explainability. Whether it is health care, tax fraud or power supply management, government operations must be explainable and transparent. Conducting the work of the people requires a high level of governance and accountability. ModelOps embraces a team approach that demands open and clear communication flow from operations to data scientists to IT. It helps governments meet the demand to use AI/ML while providing open, clear guidance and powerful governance.
By implementing ModelOps, agencies will drive continued improvements and accountability, quickly getting more high-quality models into production and ensuring they stay at peak performance to drive valuable decisions. We believe more agencies will be turning to ModelOps to facilitate smooth, efficient and continuous development and deployment of high-impact analytic models for the best business insights.