Technique improves autonomous car navigation in tricky traffic
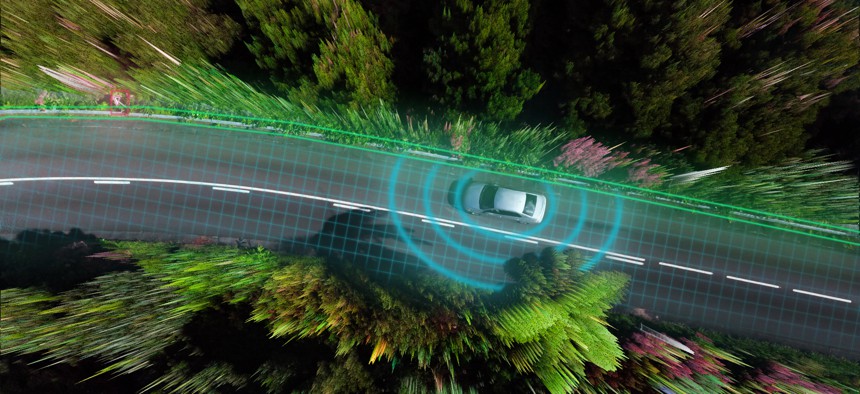
Artur Debat/Getty Images
Connecting state and local government leaders
Researchers have developed a technique that allows autonomous vehicle software to make the relevant calculations more quickly.
The technique improved both traffic and safety in simulated autonomous vehicle systems.
If autonomous vehicles are ever going to achieve widespread adoption, we need to know they are capable of navigating complex traffic situations, such as merging into heavy traffic when lanes disappear on a highway.
“Right now, the programs designed to help autonomous vehicles navigate lane changes rely on making problems computationally simple enough to resolve quickly, so the vehicle can operate in real time,” says Ali Hajbabaie, an assistant professor of civil, construction, and environmental engineering at North Carolina State University, and corresponding author of a paper published in Transportation Research Part C.
“However, simplifying the problem too much can actually create a new set of problems, since real world scenarios are rarely simple.
“Our approach allows us to embrace the complexity of real-world problems. Rather than focusing on simplifying the problem, we developed a cooperative distributed algorithm. This approach essentially breaks a complex problem down into smaller sub-problems, and sends those to different processors to solve separately. This process, called parallelization, improves efficiency significantly.”
At this point, the researchers have only tested their approach in simulations, where the sub-problems are shared among different cores in the same computing system. However, if autonomous vehicles ever use the approach on the road, the vehicles would network with each other and share the computing sub-problems.
In proof-of-concept testing, the researchers looked at two things: whether their technique allowed autonomous vehicle software to solve merging problems in real time; and how the new “cooperative” approach affected traffic and safety compared to an existing model for navigating autonomous vehicles.
In terms of computation time, the researchers found their approach allowed autonomous vehicles to navigate complex freeway lane merging scenarios in real time in moderate and heavy traffic, with spottier performance when traffic volumes got particularly high.
But when it came to improving traffic and safety, the new technique did exceptionally well. In some scenarios, particularly when traffic volume was lower, the two approaches performed about the same.
But in most instances, the new approach outperformed the previous model by a considerable margin. What’s more, the new technique had zero incidents where vehicles had to come to a stop or where there were “near crash conditions.” The other model’s results included multiple scenarios where there were literally thousands of stoppages and near crash conditions.
“For a proof-of-concept test, we’re very pleased with how this technique has performed,” Hajbabaie says. “There is room for improvement, but we’re off to a great start. The good news is that we’re developing these tools and tackling these problems now, so that we’re in a good position to ensure safe autonomous systems as they are adopted more widely.”
Source: NC State
This article was originally published in Futurity. It has been republished under the Attribution 4.0 International license.