When data science shortcuts are a bad idea
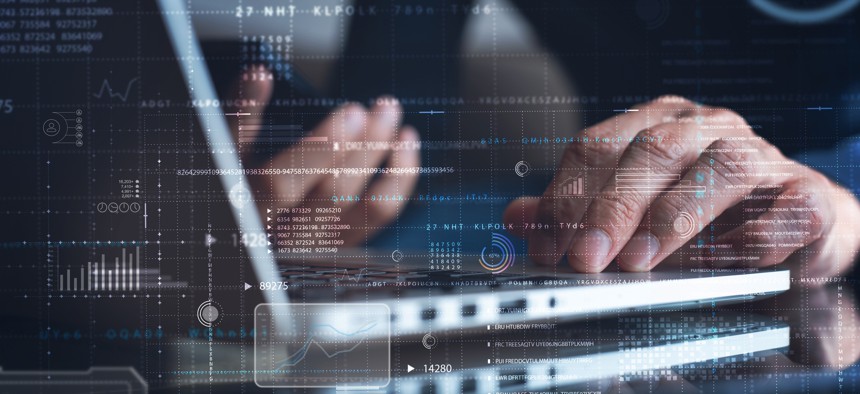
Tippapatt/Getty Images
Connecting state and local government leaders
Auto-ML can help non-data scientists write programs, but the resulting applications may be flawed, biased or useless, an expert says. Still, agencies can minimize risk and optimize the use of citizen data scientists.
Enabling employees to work as citizen data scientists has many benefits—if agencies take the right precautions, according to a recent paper.
The idea of a citizen data scientist—a person who creates analytic models such as artificial intelligence (AI) and machine learning (ML)—makes sense, said Reid Blackman, who coauthored “The Risks of Empowering ‘Citizen Data Scientists’” with Tamara Snipes, chief data scientist at UnitedHealthcare. Harvard Business Review published the paper in December 2022.
“Data scientists are a very narrow bunch of people,” Blackman said in an interview with GCN. Their expertise is relatively narrow, and they usually aren’t familiar with business problems specific to the government sector, he explained. Agencies looking to leverage AI might think: “It would help if people who aren’t actually data scientists but are really familiar with a problem that has to be overcome have some degree of training … to say, ‘Actually, you know what? I think we could solve this problem for you with such and such data.’”
Public-sector use cases for what the paper terms auto-ML, or “software that provides methods and processes for creating machine learning code,” include tax fraud detection, facial recognition to track down missing children and uncovering red flags that could lead law enforcement to further investigate and thwart terrorist activities, said Blackman, founder and CEO of Virtue, an ethical risk consultancy.
But the paper identifies three main problems associated with citizen data scientists using auto-ML. The first is that the technology “does not solve for gaps in expertise, training and experience, thus increasing the probability of failure,” according to the paper. For example, a dataset containing few instances of suspicious transactions must be sampled carefully to be usable as training data—something that “sits squarely in the expertise of the experienced data scientist.”
Second is biased AI, which experts are still working to understand. Even if AI novices are aware of the potential for bias, they will “certainly not know how to identify those risks and devise appropriate risk-mitigation strategies,” the paper states.
Third, because of the first two potential pitfalls, citizen data scientists’ work may be fruitless, amounting to “wasted efforts and internal resources,” the paper states.
Although the risks are the same in the public and private sectors, Blackman told GCN, the stakes are often higher with government, which “has a greater responsibility than a corporation to secure the welfare of a population to guard against violations of human rights, people being wronged, etc.”
One particular area of concern is privacy. Because the general rule is that “the more data you have, all things being equal, the more accurate your AI is going to be…. You’re highly incentivized to gather as much data as you can, which, in turn, incentivizes you to gather data that [you] might not have the right to have,” Blackman said. It could be a violation of privacy for a government agency to acquire all that data, he added.
Privacy must also be considered in the outputs of the ML model to avoid making inferences. He cited the example of Target using algorithms to determine the likelihood that female shoppers are pregnant—a move that revealed a teen’s pregnancy to her father.
Despite these risks, democratized AI offers many benefits and isn’t about to go away, Blackman said. “If any type of emerging tech is here to stay, it’s certainly that,” he said. “Billions and billions of dollars are being poured into AI research.”
To minimize risk, agencies can ask themselves if the benefits outweigh the threats both in general and in particular, such as those related to a single use case or application. But it’s not always about a cost/benefit analysis, Blackman said. “In some instances, we might be able to maximize benefit by wronging some subset of people,” he said. “Just thinking in terms of cost/benefit can be dangerous because it ignores the fact that certain benefits are not worth pursuing if it requires violating people’s rights.”
The paper offers more tips for optimizing the use of citizen data scientists:
- Vet AI for technical, ethical, reputational, regulatory and legal risks before putting it into production.
- Provide ongoing education on best practices and use cases that the agency can pull from.
- Create an expert mentor program for those new to AI.
- Have experts review all projects before AI is used.
Getting a handle on this one area will help with other emerging technologies that affect government, such as blockchain, augmented and virtual reality and digital twins, Blackman said. “One thing that I worry about is that government does need to take care to address the risks of AI, but it needs to widen its purview to recognize that there are lots of digital technologies that are here and then coming down the pipeline,” he said.
Stephanie Kanowitz is a freelance writer based in northern Virginia.
NEXT STORY: Philosophers have studied ‘counterfactuals’ for decades. Will they help us unlock the mysteries of AI?